Using edit distance and junction feature to detect and recognize arrow road marking
ITSC(2014)
摘要
Arrow road markings usually appear on freeway surface and they convey important navigation information to autonomous driving. But detecting and recognizing them is a tough task because they suffer from numerous deviations like objects' interference and themselves' abrasion, etc. We therefore propose a novel local junction feature (L-junction) to describe each road marking as a junction string, different deviation is dispersed into different junction. We encode those junctions within a range as the same code. To measure the similarity between detected junction string and ground truth junction string, we design a weighted edit distance strategy and assign different deviation with different weight so that our framework is robust enough to deviations in arrow road marking but sensitive to non- arrow road markings' deviations. To test our framework, we collect three freeway datasets with our self-driving car: clean/dirty arrow road marking images (300 images respectively), a video dataset (arrow road marking and non-road marking images (670 images)). Another deep learning framework (Boosting+Convolutional Deep Neural Network (CDNN)) is also implemented for comparison. Extensive experimental results well demonstrate the superior performance of our framework.
更多查看译文
关键词
driver information systems,image recognition,learning (artificial intelligence),neural nets,object detection,video coding,cdnn,l-junction,arrow road marking,arrow road marking detection,arrow road marking recognition,autonomous driving,boosting-convolutional deep neural network,clean arrow road marking images,deep learning framework,dirty arrow road marking image,freeway datasets,freeway surface,ground truth junction string,junction encoding,local junction feature,navigation information,nonarrow road marking deviations,self-driving car,video dataset,weighted edit distance strategy,learning artificial intelligence
AI 理解论文
溯源树
样例
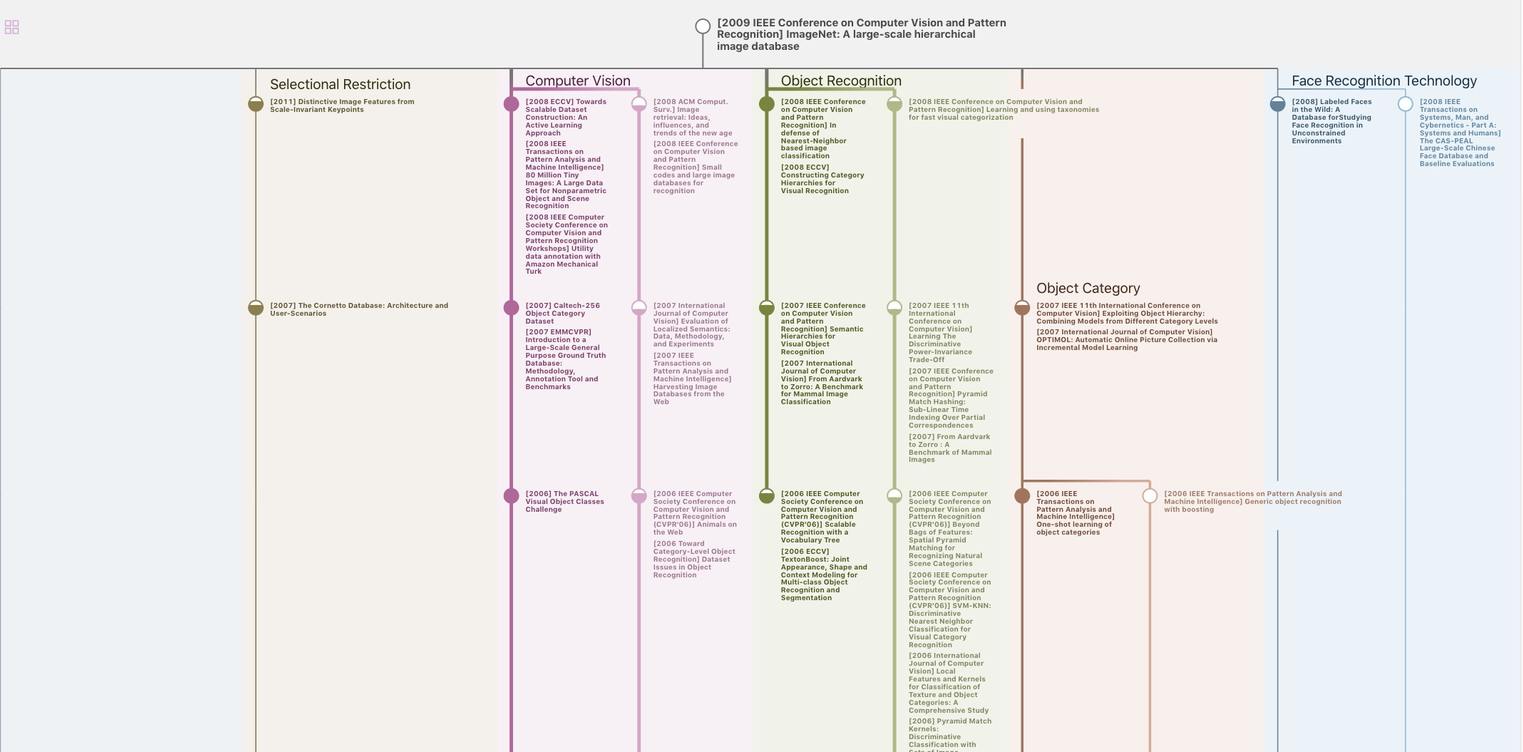
生成溯源树,研究论文发展脉络
Chat Paper
正在生成论文摘要