A Statistical Prediction Model Based on Sparse Representations for Single Image Super-Resolution
Image Processing, IEEE Transactions (2014)
摘要
We address single image super-resolution using a statistical prediction model based on sparse representations of low- and high-resolution image patches. The suggested model allows us to avoid any invariance assumption, which is a common practice in sparsity-based approaches treating this task. Prediction of high resolution patches is obtained via MMSE estimation and the resulting scheme has the useful interpretation of a feedforward neural network. To further enhance performance, we suggest data clustering and cascading several levels of the basic algorithm. We suggest a training scheme for the resulting network and demonstrate the capabilities of our algorithm, showing its advantages over existing methods based on a low- and high-resolution dictionary pair, in terms of computational complexity, numerical criteria, and visual appearance. The suggested approach offers a desirable compromise between low computational complexity and reconstruction quality, when comparing it with state-of-the-art methods for single image super-resolution.
更多查看译文
关键词
image representation,image resolution,least mean squares methods,statistical analysis,MMSE estimation,computational complexity,data clustering,feedforward neural network,high-resolution image patch,low-resolution image patch,numerical criteria,single image super-resolution,sparse representations,sparsity-based approach,statistical prediction model,visual appearance,Dictionary learning,MMSE estimation,feedforward neural networks,nonlinear prediction,restricted Boltzmann machine,single image super-resolution,sparse representations,statistical models,zooming deblurring
AI 理解论文
溯源树
样例
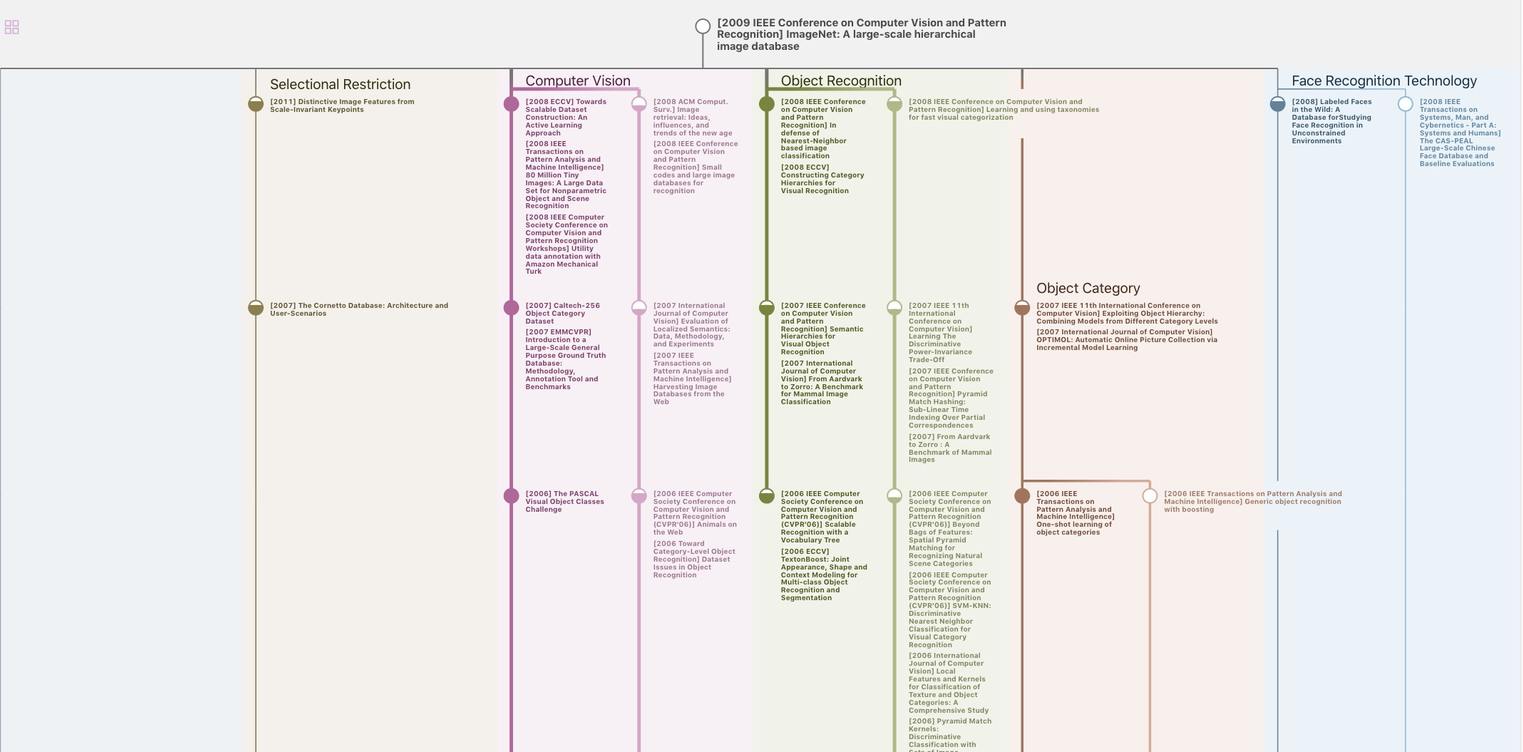
生成溯源树,研究论文发展脉络
Chat Paper
正在生成论文摘要