A design and implementation framework for unsupervised high-resolution recursive filters in neuromotor prosthesis applications
Pacific Grove, CA(2014)
摘要
Neuromotor prostheses have the potential of restoring movement ability in patients with severe motor dysfuncion. In cortically-controlled neuromotor prostheses, the design of neural decoders for motor impaired patients requires initialization using a concurrently measured set of neural and motor imagery or observation data. In addition, the decoder implementation poses a scalability challenge with an increasing number of decoded neurons. Consequently, most neural decoder implementations resort to sub-sampling the neural firing rates, which results in noisy decoded outputs. In this work, we propose a new decoder design and implementation framework in which (i) the decoder initialization is unsupervised, (ii) the decoder is implemented using computationally-inexpensive recursive filters that can operate at high-resolution sampling of the neural data thereby minimizing the delay introduced in the system, and (iii) the decoder gives a smooth real-time control signal expressed by the span of neural data projections onto a low-dimensional latent space that possesses desirable features for the control task.
更多查看译文
关键词
brain-computer interfaces,decoding,patient rehabilitation,prosthetics,recursive filters,unsupervised learning,cortically-controlled neuromotor prostheses,decoded neurons,low-dimensional latent space,motor dysfuncion,motor imagery,motor impaired patients,neural data projections,neural decoder implementations,neural decoders,neural firing rates,neural imagery,observation data,real-time control signal,unsupervised high-resolution recursive filters
AI 理解论文
溯源树
样例
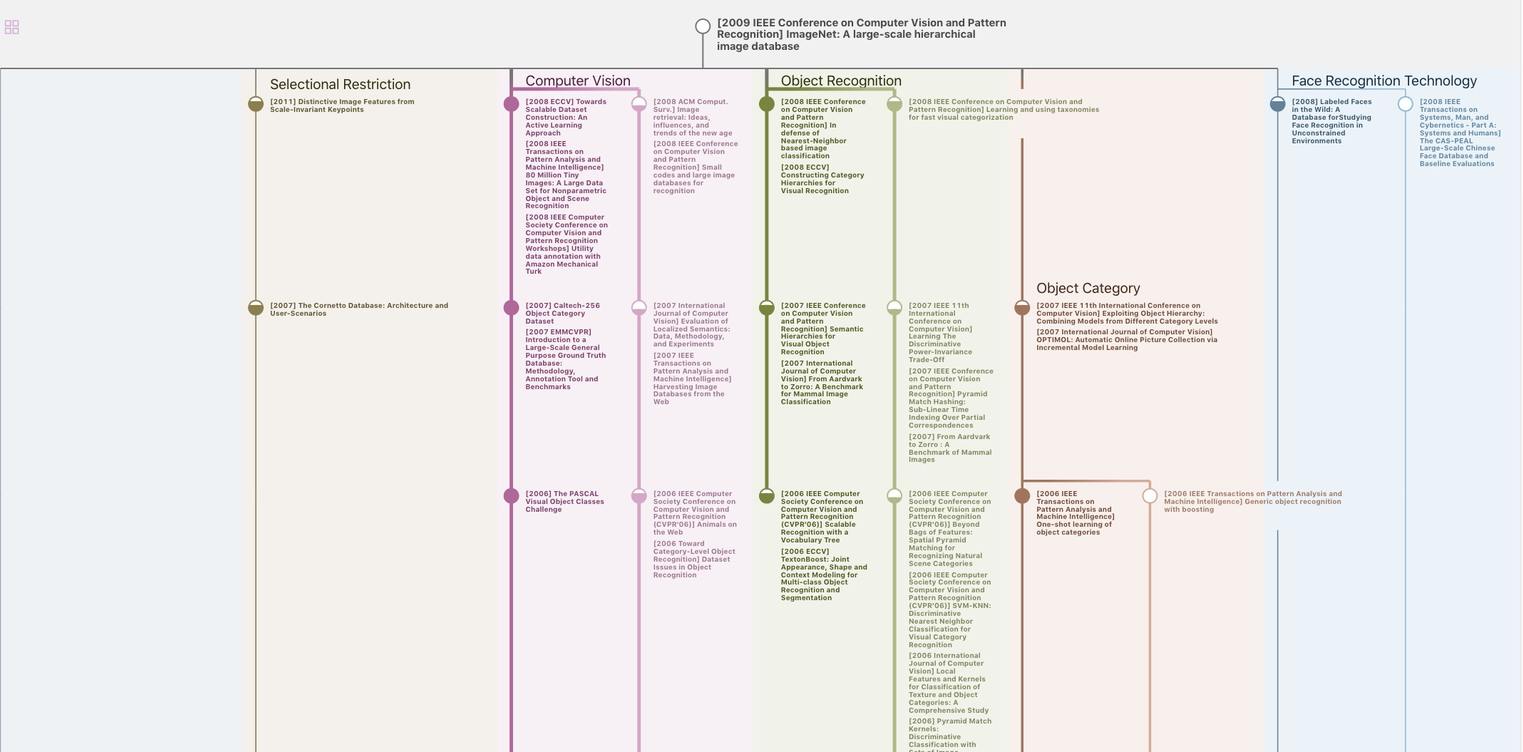
生成溯源树,研究论文发展脉络
Chat Paper
正在生成论文摘要