Bayesian analysis of similarity matrices for speaker diarization
Acoustics, Speech and Signal Processing(2014)
摘要
Inspired by recent success of speaker clustering in Total Variability space we propose a new probabilistic model for speaker diarization based on Bayesian modeling of pairwise similarity scores. The recordings are represented by symmetric similarity matrices of likelihood ratio scores from probabilistic linear discriminant analysis (PLDA) trained on short-term i-vectors. We employ Bayesian approach to address the problem of unknown number of speakers in conversation. Diarization error rates on the NIST 2008 SRE telephone data indicate comparable performance with state-of-the-art eigenvoice-based diarization. But unlike the eigenvoice approach, our method finds the number of speakers automatically, making the proposed model more viable for practical applications.
更多查看译文
关键词
Bayes methods,pattern clustering,speaker recognition,vectors,Bayesian analysis,Bayesian modeling,NIST 2008 SRE telephone data,PLDA,diarization error rates,likelihood ratio scores,pairwise similarity scores,probabilistic linear discriminant analysis,probabilistic model,recording representation,short-term i-vectors,speaker clustering,speaker diarization,symmetric similarity matrices,total variability space,Speaker diarization,similarity matrix,variational Bayesian inference
AI 理解论文
溯源树
样例
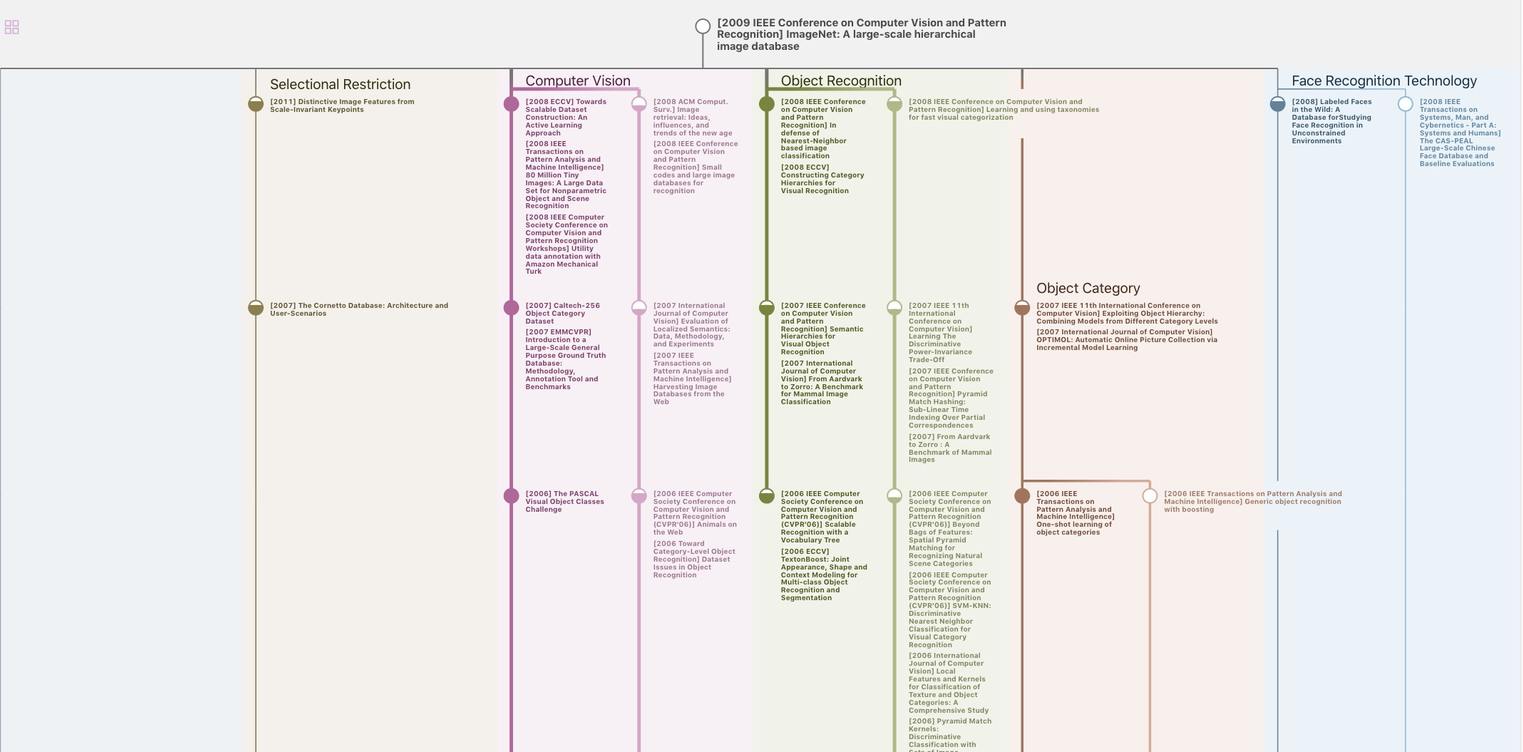
生成溯源树,研究论文发展脉络
Chat Paper
正在生成论文摘要