An improved artificial immune network for multimodal function optimization
Control and Decision Conference(2014)
Abstract
Multimodal optimization problems are typical those problems where both a global optimum and one or more local optima are included, which can be tackled with by evolutionary algorithms. This paper proposes an improved artificial immune network for multimodal function optimization. In the antibody population, antibodies are allocated to three spaces, i.e., the elitist space, the common space and the poor space. To be specific, the antibodies in the elitist space undergo self-learning mutation, those in the common space undergo elitist-learning mutation and those in the poor space undergo the random hypermutation. At each generation, the mutated antibodies with higher affinity are regrouped into a new elitist space while those with medium affinity are regrouped into a new common space. On the other hand, the old poor space is removed; instead, a group of randomly generated antibodies are grouped into a new poor space. Six benchmark functions in both 10D and 30D are used to evaluate the optimization performance of the proposed artificial immune network and the other existing artificial immune networks, such as opt-aiNet, IA-AIS and AINet-SL. The simulation results indicate that the proposed artificial immune network is an effective and relative efficient method in optimizing both the unimodal and multimodal functions.
MoreTranslated text
Key words
artificial immune systems,AINet-SL network,IA-AIS network,antibody population,artificial immune network,common space,elitist space,elitist-learning mutation,evolutionary algorithms,multimodal function optimization,opt-aiNet network,poor space,random hypermutation,self-learning mutation,Artificial immune network,dynamic updating,elitist learning,multimodal optimization
AI Read Science
Must-Reading Tree
Example
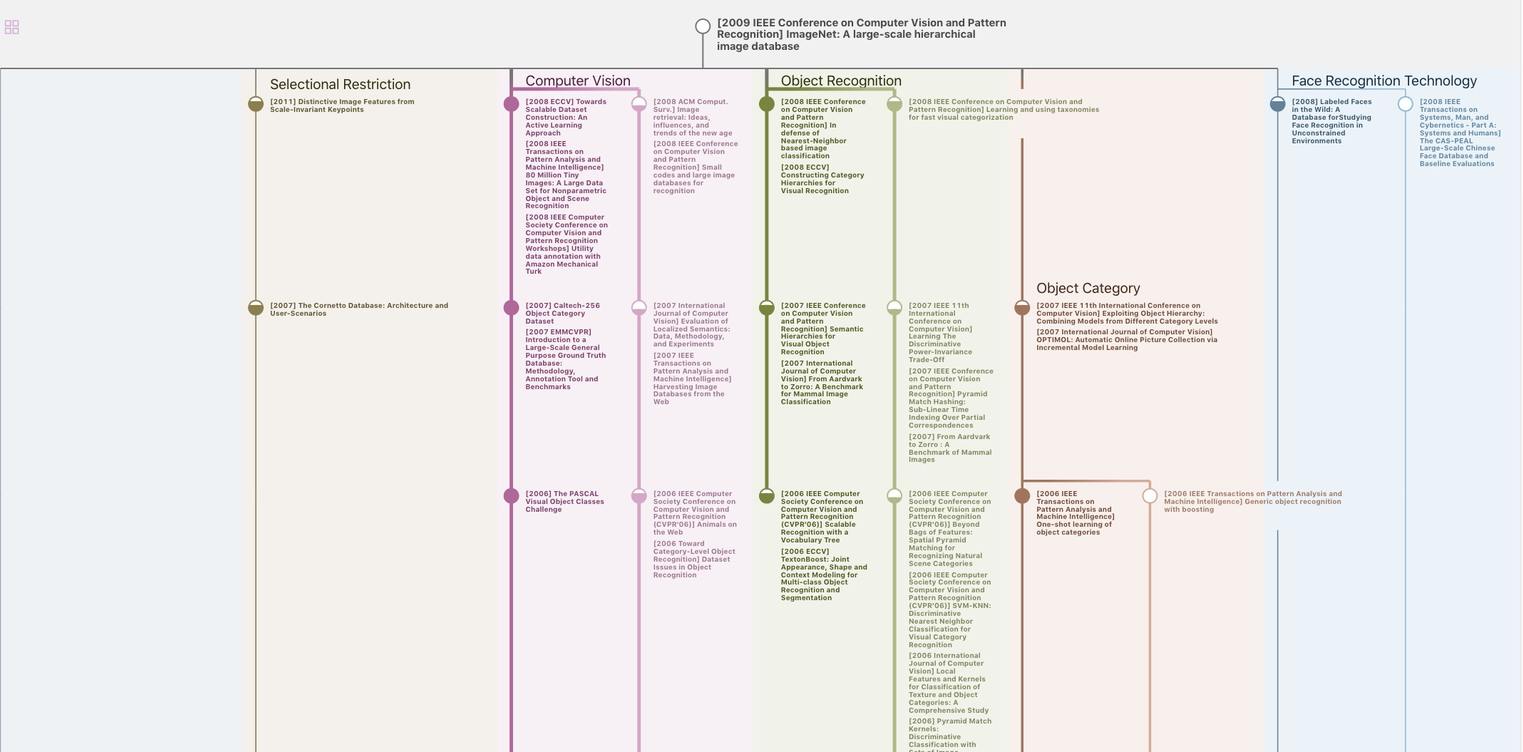
Generate MRT to find the research sequence of this paper
Chat Paper
Summary is being generated by the instructions you defined