A multi-model reverse-engineering algorithm for large gene regulation networks
Bioinformatics and Biomedicine(2014)
摘要
Modeling and simulation of gene-regulatory networks (GRNs) has become an important aspect of modern systems biology investigations into mechanisms underlying gene regulation. An important and unsolved problem in this area is the automated inference (reverse-engineering) of dynamic, mechanistic GRN models from time-course gene expression data. The conventional one-stage model inference algorithm determines the values of all model parameters simultaneously. Recently, two-stage algorithms have been proposed to improve the accuracy of the inferred models and the efficiency of the reverse-engineering process. The main objective of this study is to compare the performance of the conventional one-stage and the modern two-stage algorithm, with emphasis on the computational complexity. We explored data generated from artificial and real GRN systems under different experimental conditions and regulatory structure constraints. Our results suggest that the 2-stage approach outperforms the one-stage methods by far in terms of model inference speed without a loss of accuracy.
更多查看译文
关键词
biological techniques,computational complexity,genomics,computational complexity,gene-regulatory networks,large gene regulation networks,model inference speed,multimodel reverse-engineering algorithm,one-stage algorithm,regulatory structure constraints,time-course gene expression data,two-stage algorithm
AI 理解论文
溯源树
样例
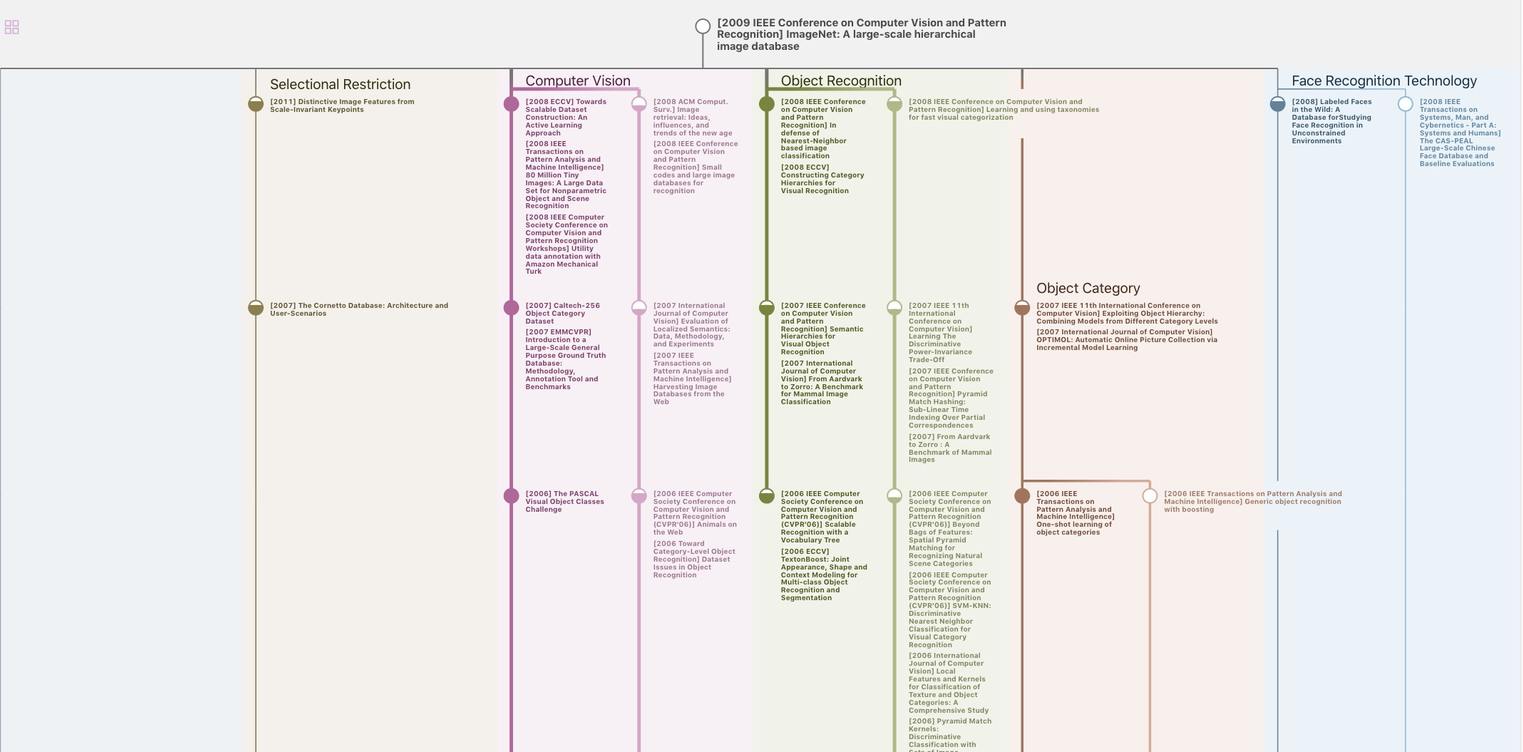
生成溯源树,研究论文发展脉络
Chat Paper
正在生成论文摘要