Learning New Parts for Landmark Localization in Whole-Body CT Scans
Medical Imaging, IEEE Transactions (2014)
摘要
The goal of this work is to reliably and accurately localize anatomical landmarks in 3-D computed tomography scans, particularly for the deformable registration of whole-body scans, which show huge variation in posture, and the spatial distribution of anatomical features. Parts-based graphical models (GM) have shown attractive properties for this task because they capture naturally anatomical relationships between landmarks. Unfortunately, standard GMs are learned from manually annotated training images and the quantity of landmarks is limited by the high cost of expert annotation. We propose a novel method that automatically learns new corresponding landmarks from a database of 3-D whole-body CT scans, using a limited initial set of expert-labeled ground-truth landmarks. The newly learned landmarks, called B-landmarks, are used to build enriched GMs. We compare our method of deformable registration based on such GM landmarks to a conventional deformable registration method and to a “baseline” state-of-the-art GM. The results show our method finds new relevant anatomical correspondences and improves by up to 35% the matching accuracy of highly variable skeletal and soft-tissue landmarks of clinical interest.
更多查看译文
关键词
computerised tomography,image registration,learning (artificial intelligence),medical image processing,3D computed tomography scans,3D whole-body CT scans,B-landmarks,GM,annotated training images,clinical interest,deformable registration,expert-labeled ground-truth landmarks,landmark localization,learning,matching accuracy,part-based graphical models,soft-tissue landmarks,spatial distribution,Computed tomography (CT),graphical models,image registration,oncology,semi-supervised learning
AI 理解论文
溯源树
样例
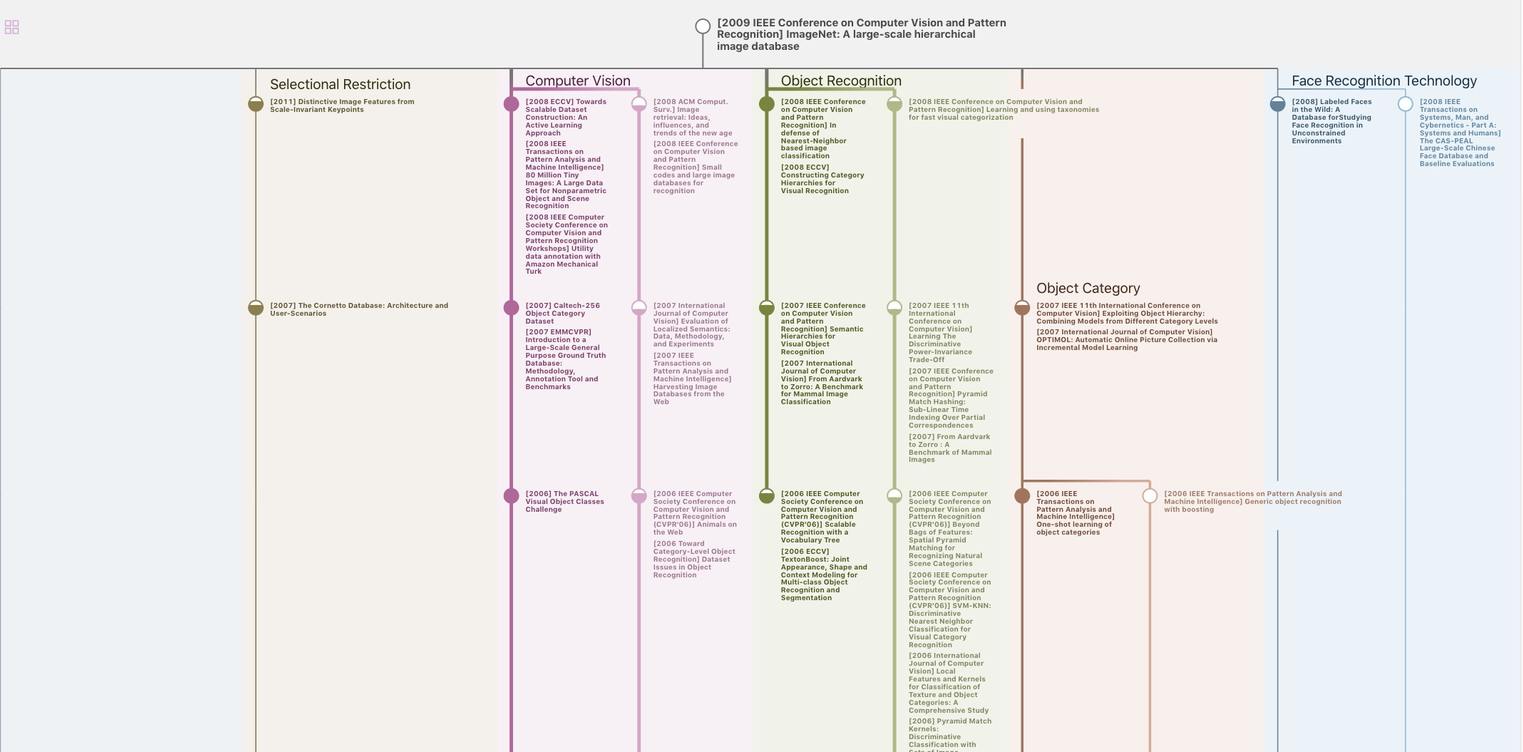
生成溯源树,研究论文发展脉络
Chat Paper
正在生成论文摘要