A Unified Framework for Outlier Detection in Trace Data Analysis
Semiconductor Manufacturing, IEEE Transactions (2014)
摘要
Process trace data (PTD) is an important data type in semiconductor manufacturing and has a very large aggregate volume. While data mining and statistical analysis play a key role in the quality control of wafers, the existence of outliers adversely affects the applications benefiting from PTD analysis. Due to the complexities of PTD and the resultant outlier patterns, this paper proposes a unified outlier detection framework which takes advantages of data complexity reduction using entropy and abrupt change detection using cumulative sum (CUSUM) method. To meet the practical needs of PTD analysis, a two-step algorithm taking into account of the related domain knowledge is developed, and its effectiveness is validated by using real PTD sets and a production example. The experimental results show that the proposed method outperforms the Fast Greedy Algorithm (FGA) and the Grubb's test, two commonly used outlier detection techniques for univariate data.
更多查看译文
关键词
data analysis,greedy algorithms,semiconductor process modelling,statistical analysis,cusum method,fga,grubb test,ptd analysis,cumulative sum method,data complexity reduction,data mining,entropy,fast greedy algorithm,outlier detection technique,process trace data analysis,quality control,semiconductor manufacturing,unified outlier detection framework,cusum,outlier detection,information content,process trace data
AI 理解论文
溯源树
样例
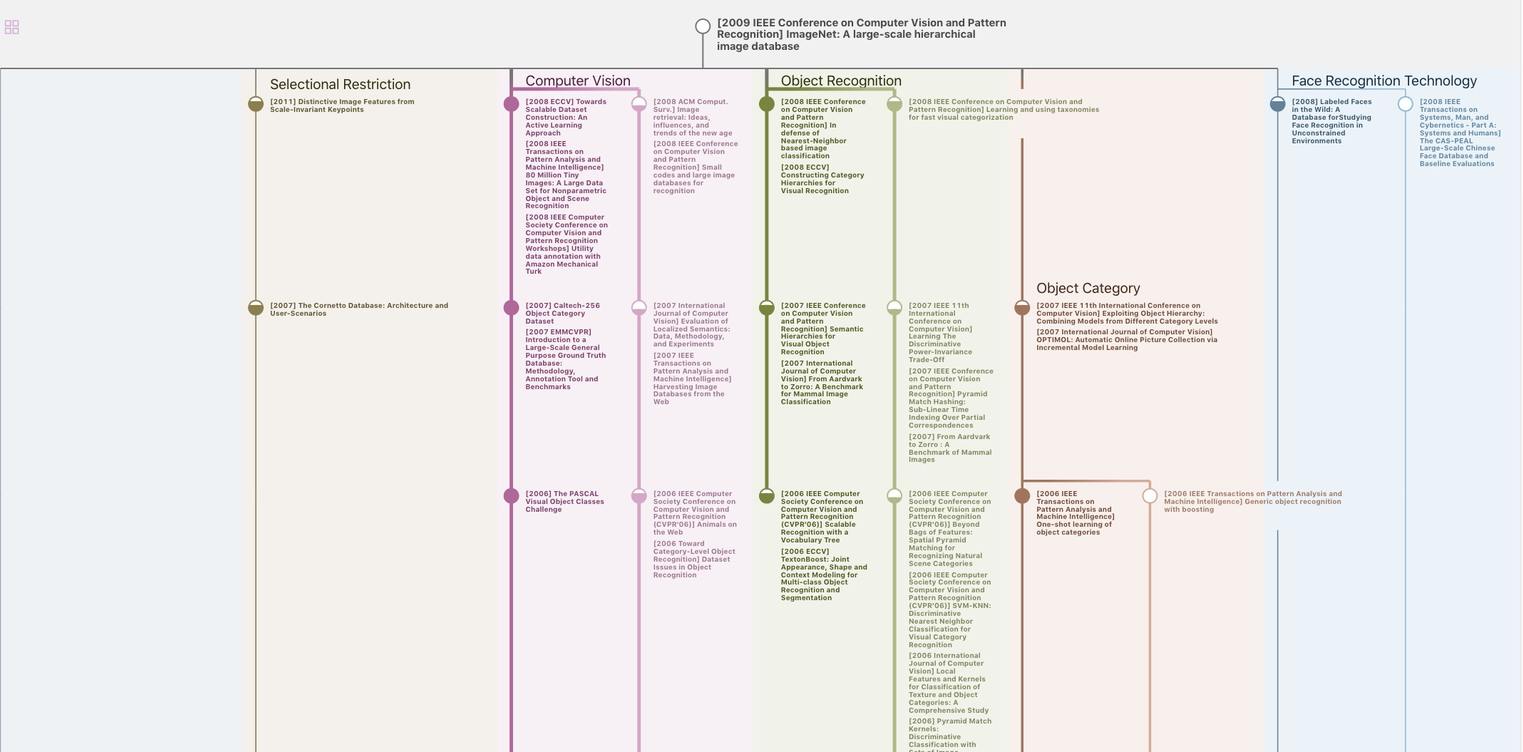
生成溯源树,研究论文发展脉络
Chat Paper
正在生成论文摘要