A task-parameterized probabilistic model with minimal intervention control
Robotics and Automation(2014)
摘要
We present a task-parameterized probabilistic model encoding movements in the form of virtual spring-damper systems acting in multiple frames of reference. Each candidate coordinate system observes a set of demonstrations from its own perspective, by extracting an attractor path whose variations depend on the relevance of the frame at each step of the task. This information is exploited to generate new attractor paths in new situations (new position and orientation of the frames), with the predicted covariances used to estimate the varying stiffness and damping of the spring-damper systems, resulting in a minimal intervention control strategy. The approach is tested with a 7-DOFs Barrett WAM manipulator whose movement and impedance behavior need to be modulated in regard to the position and orientation of two external objects varying during demonstration and reproduction.
更多查看译文
关键词
covariance matrices,manipulator dynamics,motion control,path planning,position control,probability,shock absorbers,springs (mechanical),vibration control,7-DOF Barrett WAM manipulator,attractor path extraction,candidate coordinate system,covariance prediction,damping estimation,frame orientation,frame position,impedance behavior,minimal intervention control strategy,movement behavior,task-parameterized probabilistic model,task-parameterized probabilistic model encoding movements,varying stiffness estimation,virtual spring-damper systems
AI 理解论文
溯源树
样例
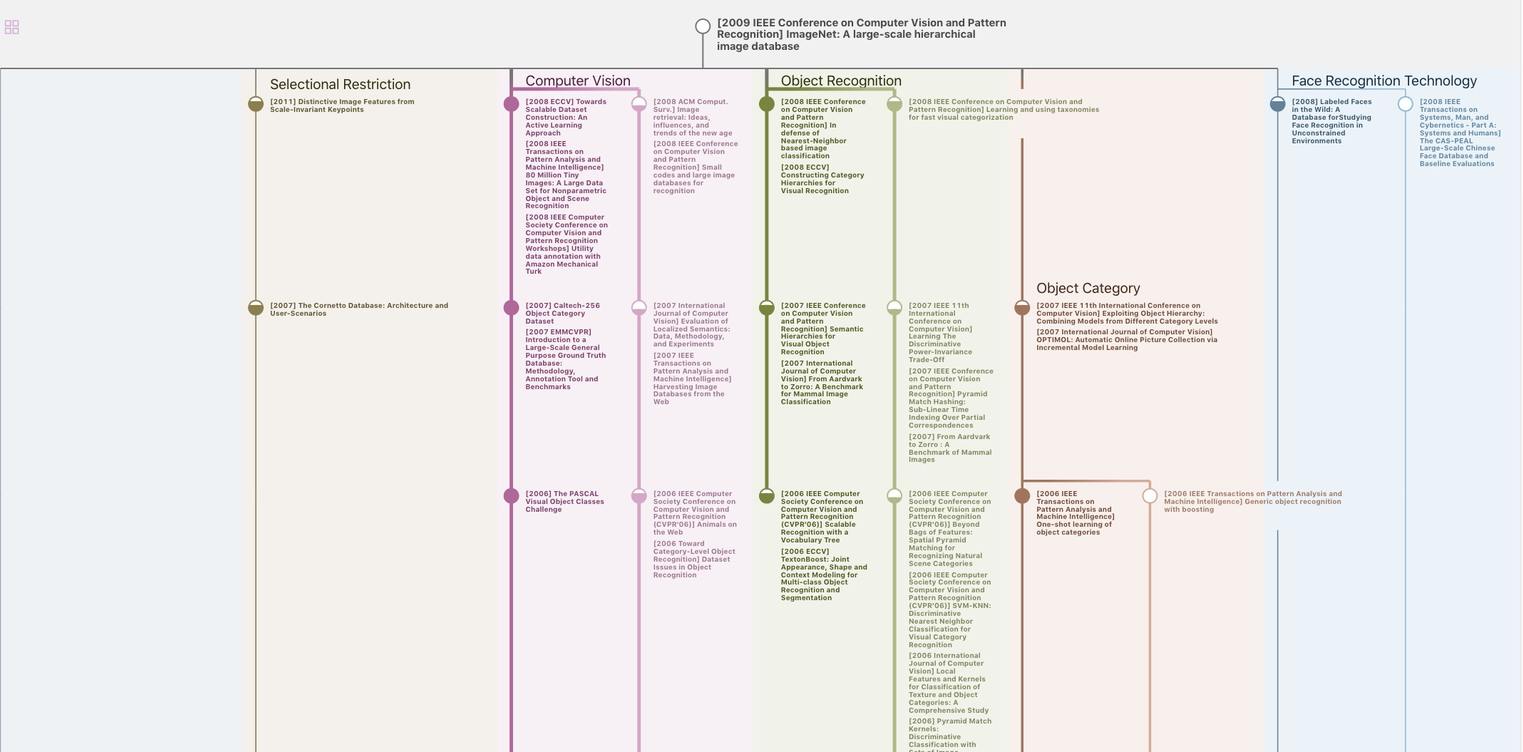
生成溯源树,研究论文发展脉络
Chat Paper
正在生成论文摘要