Joint Estimation of Multiple Undirected Graphical Models
International Workshop on Machine Learning for Signal Processing(2014)
Key words
Gaussian processes,covariance matrices,graph theory,learning (artificial intelligence),matrix inversion,maximum likelihood estimation,Gaussian graphical model,alternating direction method,conditional independence,convex maximum likelihood problem,joint estimation,network substructure,sparse inverse covariance matrix,statistical learning,undirected graphical models,zero entry
AI Read Science
Must-Reading Tree
Example
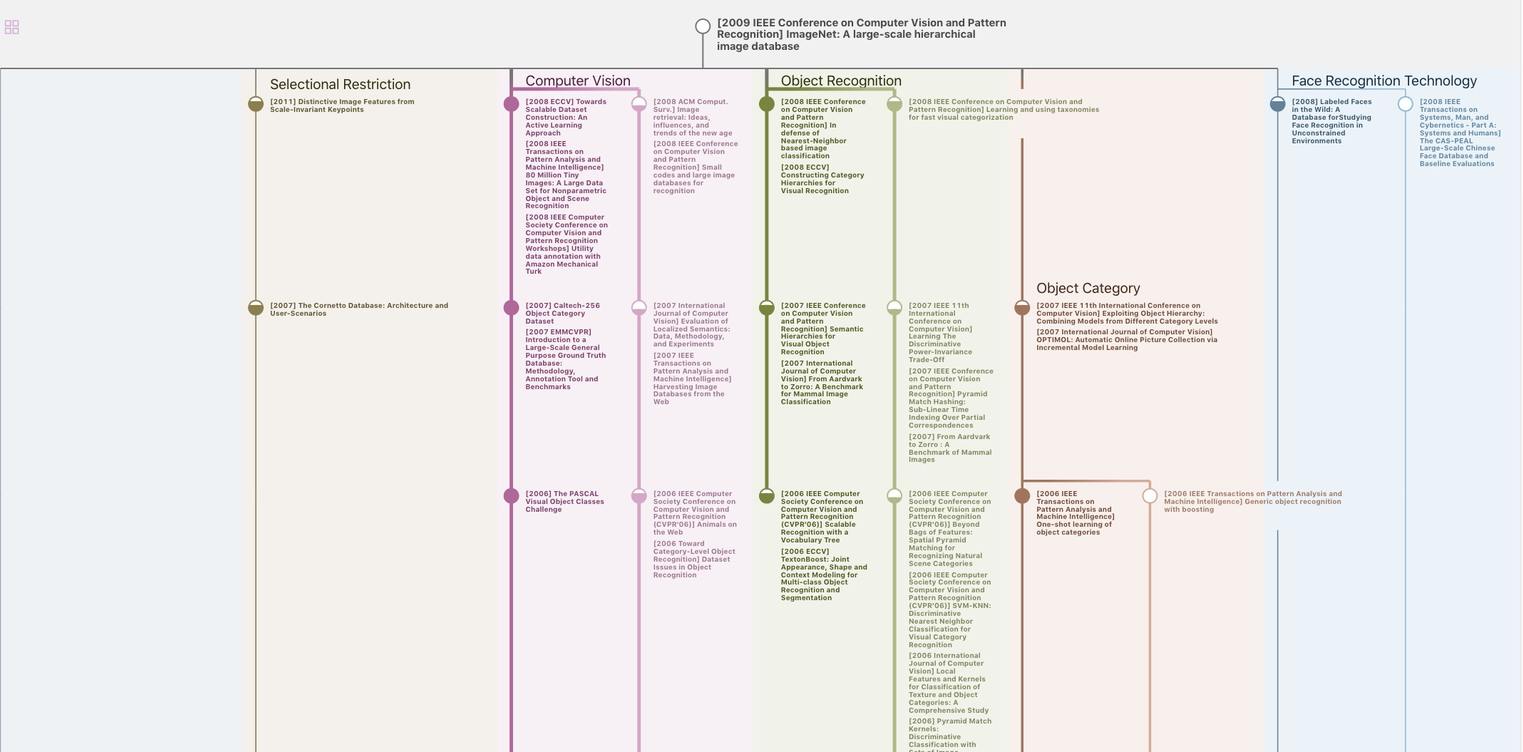
Generate MRT to find the research sequence of this paper
Chat Paper
Summary is being generated by the instructions you defined