On the use of classifiers for text-independent speaker identification
Automation, Control, Energy and Systems(2014)
Abstract
In this paper we have presented the comparative study of different modelling techniques (classifiers) for the text independent speaker identification. Four classifiers, namely, Gaussian mixture models, Fuzzy min-max neural network, Self organizing map, and Vector Quantization based Probabilistic Neural Network (VQ-PNN) have been used for the study. The database containing speech utterances recorded from forty two speakers in Hindi language was used for experimentation. Mel frequency cepstral coefficients that represent short time spectrum are used as features for identification. The performance of four classifiers is analysed under clean- and noisy-speech environment for different signal to noise ratios. All the four classifiers have almost similar performance for 10 second test speech utterances under clean environment. However, GMM outperforms other three classifiers under noisy test conditions.
MoreTranslated text
Key words
gaussian processes,audio databases,cepstral analysis,fuzzy neural nets,mixture models,natural language processing,probability,self-organising feature maps,signal classification,speaker recognition,text analysis,vector quantisation,gmm,gaussian mixture models,hindi language,mel frequency cepstral coefficients,vq-pnn,classifiers,clean-and noisy-speech environment,fuzzy min-max neural network,self organizing map,signal to noise ratios,speech utterance database,text-independent speaker identification,vector quantization based probabilistic neural network,som,vq based probabilistic neural network,fuzzy neural networks,speaker identification
AI Read Science
Must-Reading Tree
Example
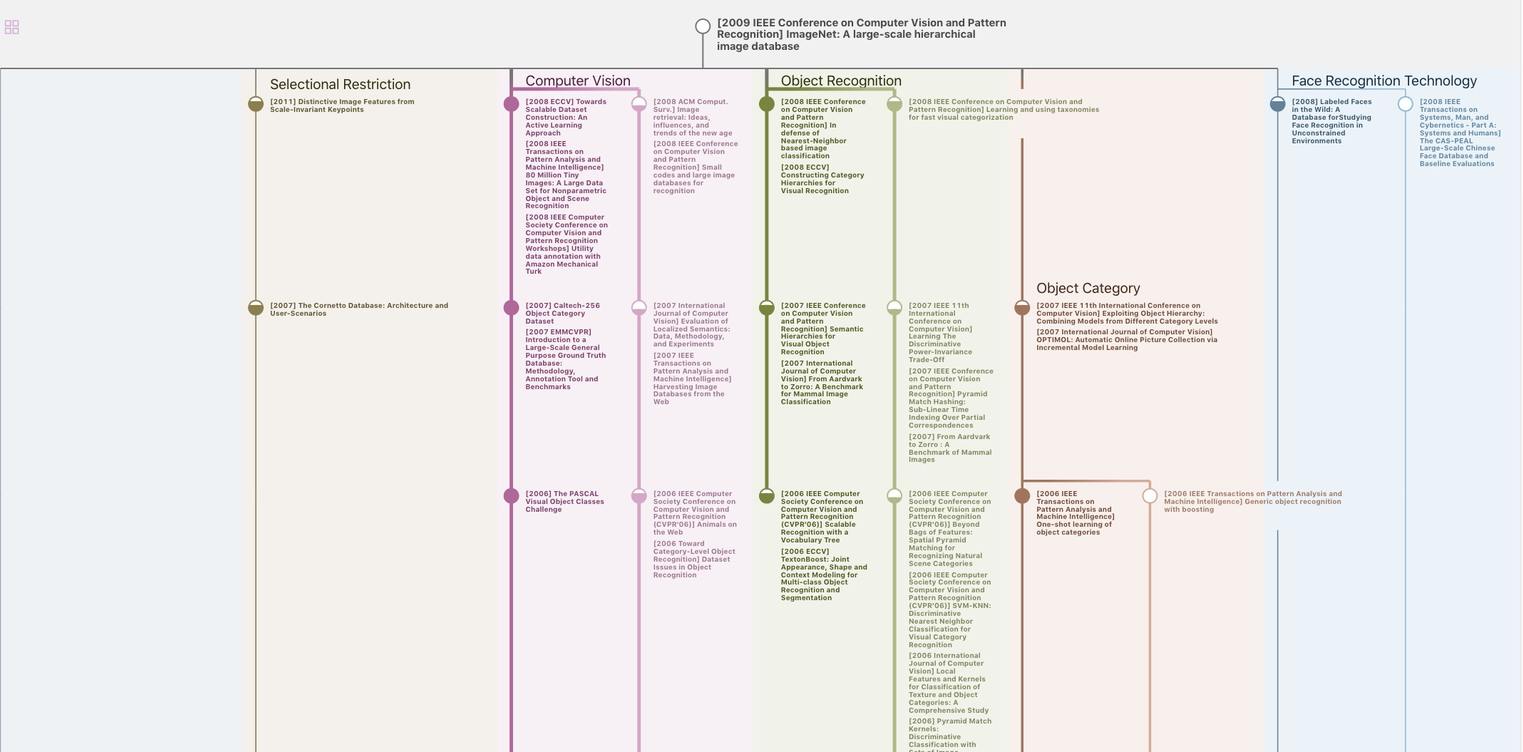
Generate MRT to find the research sequence of this paper
Chat Paper
Summary is being generated by the instructions you defined