SFP-Rank: significant frequent pattern analysis for effective ranking
Knowledge and Information Systems(2014)
摘要
Ranking documents in terms of their relevance to a given query is fundamental to many real-life applications such as information retrieval and recommendation systems. Extensive study in these application domains has given rise to the development of many efficient ranking models. While most existing research focuses on developing learning to rank (LTR) models, the quality of the training features, which plays an important role in ranking performance, has not been fully studied. Thus, we propose a new approach that discovers effective features for the LTR problem. In this paper, we present a theoretical analysis on which frequent patterns are potentially effective for improving the performance of LTR and then propose an efficient method that selects frequent patterns for LTR. First, we define a new criterion, namely feature significance (or simply significance ). Specifically, we use each feature’s value to rank the training instances and define the ranking effectiveness in terms of a performance measure as the significance of the feature. We show that the significance of an infrequent pattern is limited by using formal connection between pattern support and its significance. Then, we propose a methodology that sets the support value when performing frequent pattern mining. Finally, since frequent patterns are not equally effective for LTR, we further provide a coverage-based significant pattern generation algorithm to discover effective patterns and propose a new ranking approach called S ignificant F requent P attern-based Rank ing ( SFP-Rank ), in which the ranking model is built upon the original features as well as the significant frequent patterns. Our experiments confirm that, by incorporating significant frequent patterns to train the ranking model, the performance of the ranking model can be substantially improved.
更多查看译文
关键词
Learning to rank,Frequent patterns,Feature selection,Combined features,Ranking performance
AI 理解论文
溯源树
样例
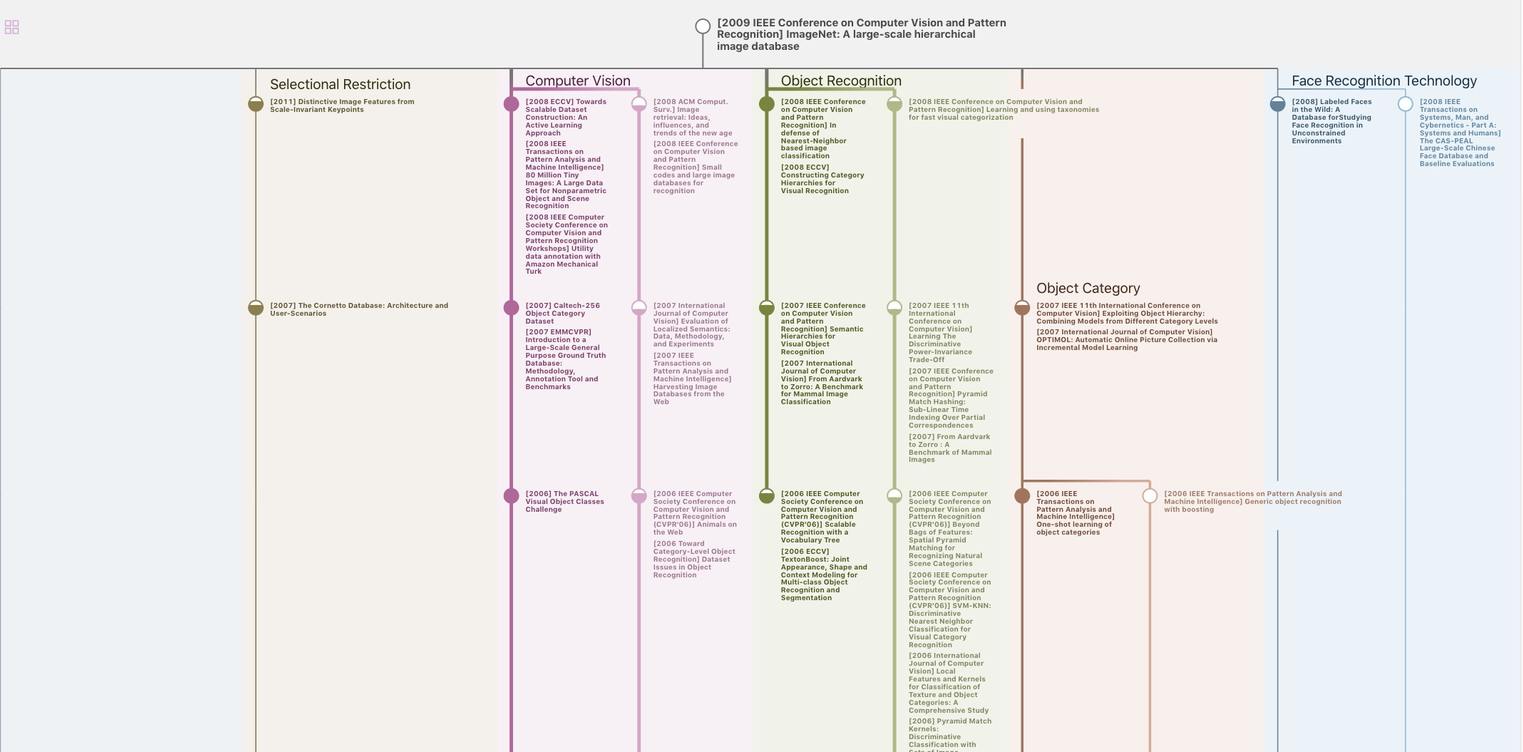
生成溯源树,研究论文发展脉络
Chat Paper
正在生成论文摘要