An Entropic Perturbation Approach To Tv-Minimization For Limited-Data Tomography
DISCRETE GEOMETRY FOR COMPUTER IMAGERY, DGCI 2014(2014)
摘要
The reconstruction problem of discrete tomography is studied using novel techniques from compressive sensing. Recent theoretical results of the authors enable to predict the number of measurements required for the unique reconstruction of a class of cosparse dense 2D and 3D signals in severely undersampled scenarios by convex programming. These results extend established l(1)-related theory based on sparsity of the signal itself to novel scenarios not covered so far, including tomographic projections of 3D solid bodies composed of few different materials. As a consequence, the large-scale optimization task based on total-variation minimization subject to tomographic projection constraints is considerably more complex than basic l(1)-programming for sparse regularization. We propose an entropic perturbation of the objective that enables to apply efficient methodologies from unconstrained optimization to the perturbed dual program. Numerical results validate the theory for large-scale recovery problems of integer-valued functions that exceed the capacity of the commercial MOSEK software.
更多查看译文
关键词
discrete tomography, compressed sensing, underdetermined systems of linear equations, cosparsity, phase transitions, total variation, entropic perturbation, convex duality, convex programming
AI 理解论文
溯源树
样例
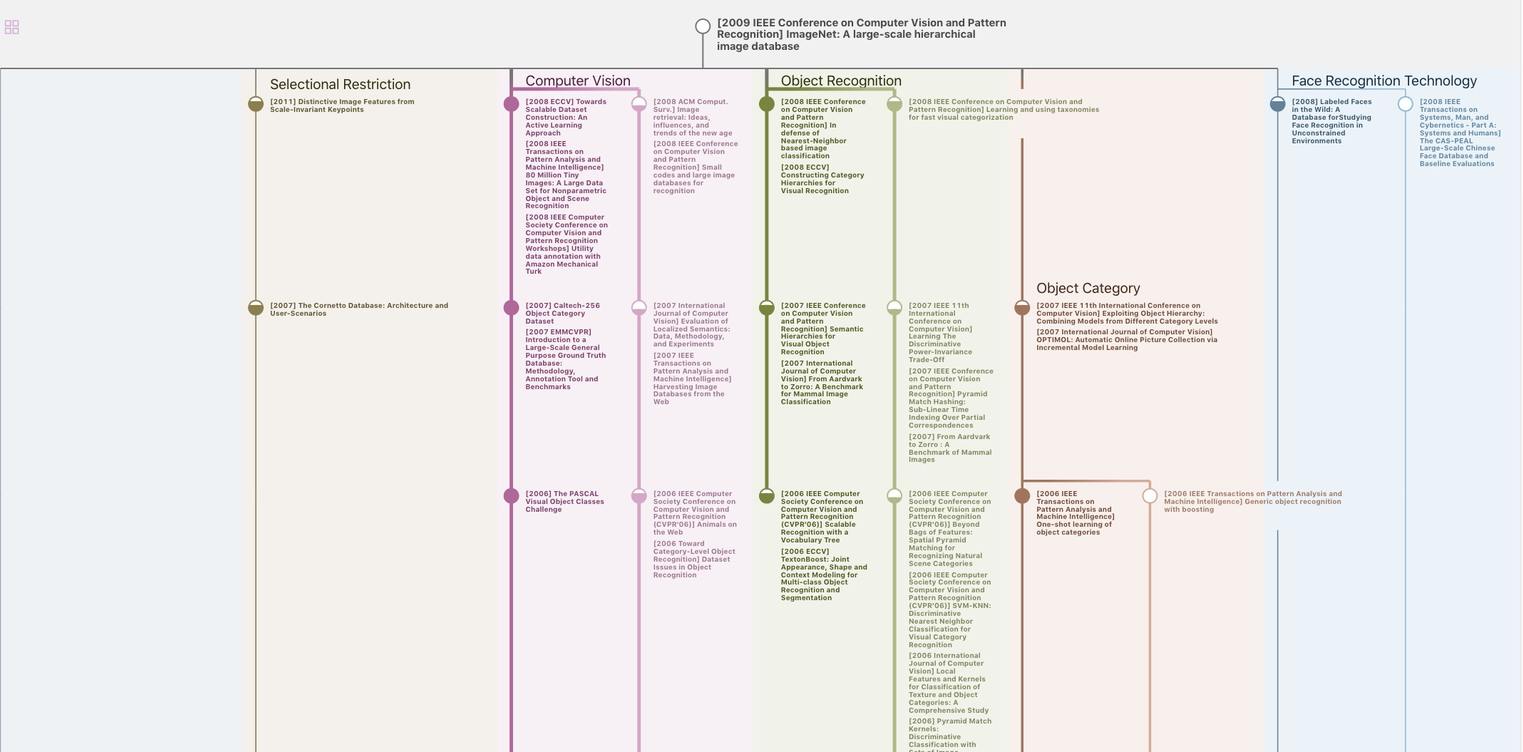
生成溯源树,研究论文发展脉络
Chat Paper
正在生成论文摘要