Embedded Unsupervised Feature Selection
PROCEEDINGS OF THE TWENTY-NINTH AAAI CONFERENCE ON ARTIFICIAL INTELLIGENCE(2015)
摘要
Sparse learning has been proven to be a powerful technique in supervised feature selection, which allows to embed feature selection into the classification (or regression) problem. In recent years, increasing attention has been on applying spare learning in unsupervised feature selection. Due to the lack of label information, the vast majority of these algorithms usually generate cluster labels via clustering algorithms and then formulate unsupervised feature selection as sparse learning based supervised feature selection with these generated cluster labels. In this paper, we propose a novel unsupervised feature selection algorithm EUFS, which directly embeds feature selection into a clustering algorithm via sparse learning without the transformation. The Alternating Direction Method of Multipliers is used to address the optimization problem of EUFS. Experimental results on various benchmark datasets demonstrate the effectiveness of the proposed framework EUFS.
更多查看译文
关键词
clustering
AI 理解论文
溯源树
样例
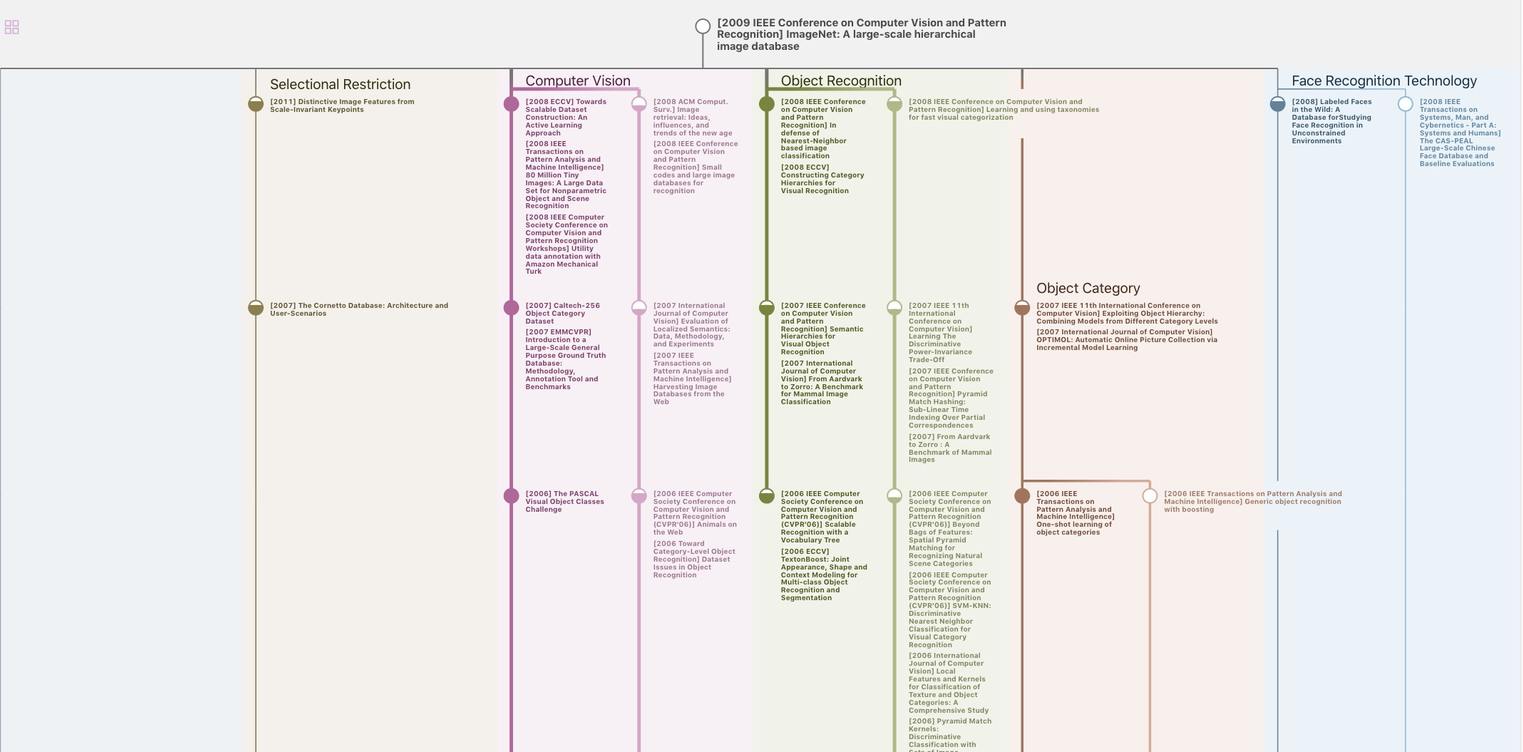
生成溯源树,研究论文发展脉络
Chat Paper
正在生成论文摘要