Priview: Practical Differentially Private Release Of Marginal Contingency Tables
MOD(2014)
摘要
We consider the problem of publishing a differentially private synopsis of a d-dimensional dataset so that one can reconstruct any k-way marginal contingency tables from the synopsis. Marginal tables are the workhorses of categorical data analysis. Thus, the private release of such tables has attracted a lot of attention from the research community. However, for situations where d is moderate to large and k is beyond 3, no accurate and practical method exists. We introduce PriView, which computes marginal tables for a number of strategically chosen sets of attributes that we call views, and then use these view marginal tables to reconstruct any desired k-way marginal. In PriView, we apply maximum entropy optimization to reconstruct k-way marginals from views. We also develop a novel method to efficiently making all view marginals consistent while correcting negative entries to improve accuracy. For view selection, we borrow the concept of covering design from combinatorics theory. We conduct extensive experiments on real and synthetic datasets, and show that PriView reduces the error over existing approaches by 2 to 3 orders of magnitude.
更多查看译文
关键词
Differential Privacy,Marginal Table,Contingency Table
AI 理解论文
溯源树
样例
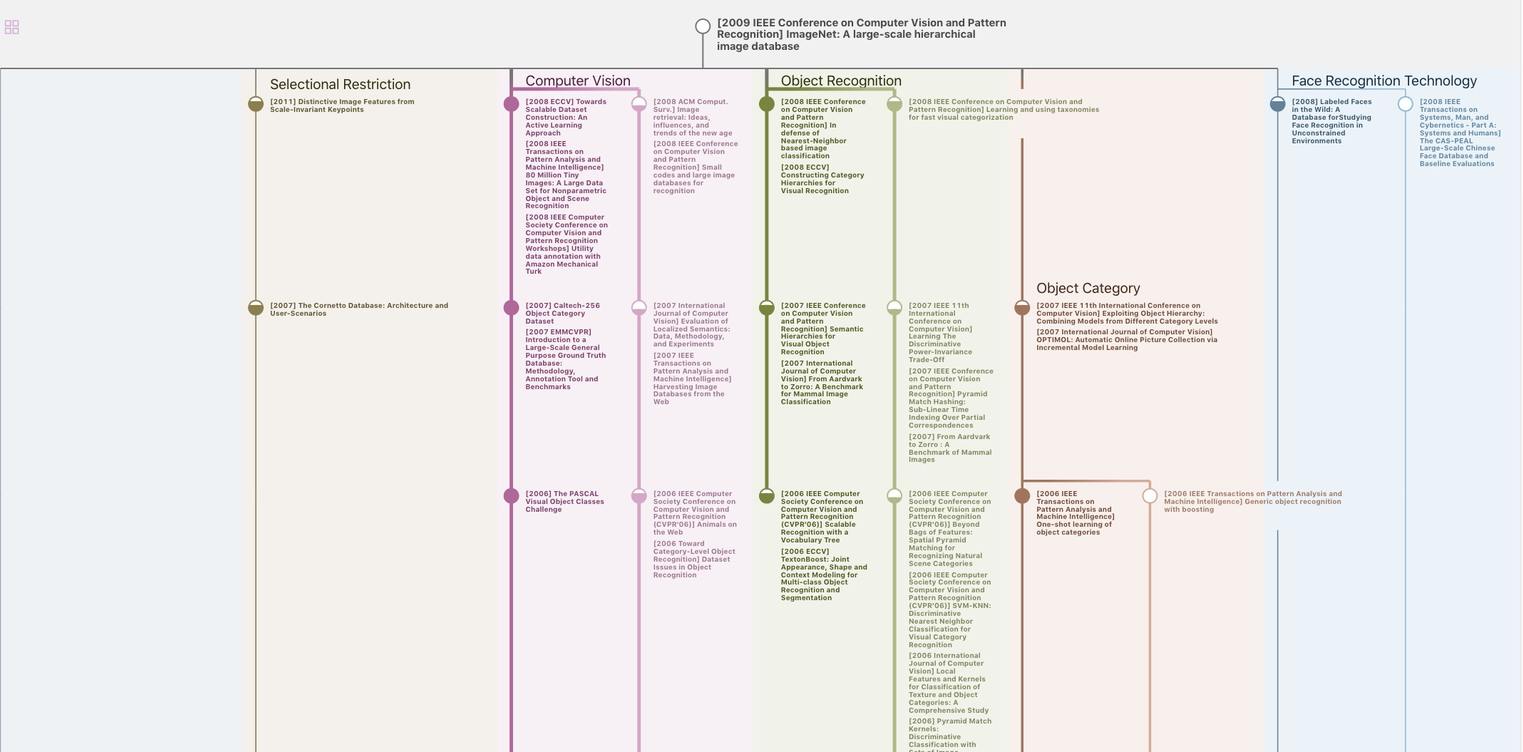
生成溯源树,研究论文发展脉络
Chat Paper
正在生成论文摘要