Multi-Label Classification For Tree And Directed Acyclic Graphs Hierarchies
PROBABILISTIC GRAPHICAL MODELS(2014)
摘要
Hierarchical Multi-label Classification (HMC) is the task of assigning a set of classes to a single instance with the peculiarity that the classes are ordered in a predefined structure. We propose a novel HMC method for tree and Directed Acyclic Graphs (DAG) hierarchies. Using the combined predictions of locals classifiers and a weighting scheme according to the level in the hierarchy, we select the "best" single path for tree hierarchies, and multiple paths for DAG hierarchies. We developed a method that returns paths from the root down to a leaf node ( Mandatory Leaf Node Prediction or MLNP) and an extension for Non Mandatory Leaf Node Prediction (NMLNP). For NMLNP we compared several pruning approaches varying the pruning direction, pruning time and pruning condition. Additionally, we propose a new evaluation metric for hierarchical classifiers, that avoids the bias of current measures which favor conservative approaches when using NMLNP. The proposed approach was experimentally evaluated with 10 tree and 8 DAG hierarchical datasets in the domain of protein function prediction. We concluded that our method works better for deep, DAG hierarchies and in general NMLNP improves MLNP.
更多查看译文
关键词
Leaf Node, Direct Acyclic Graph, Parent Node, Pruning Strategy, Music Genre
AI 理解论文
溯源树
样例
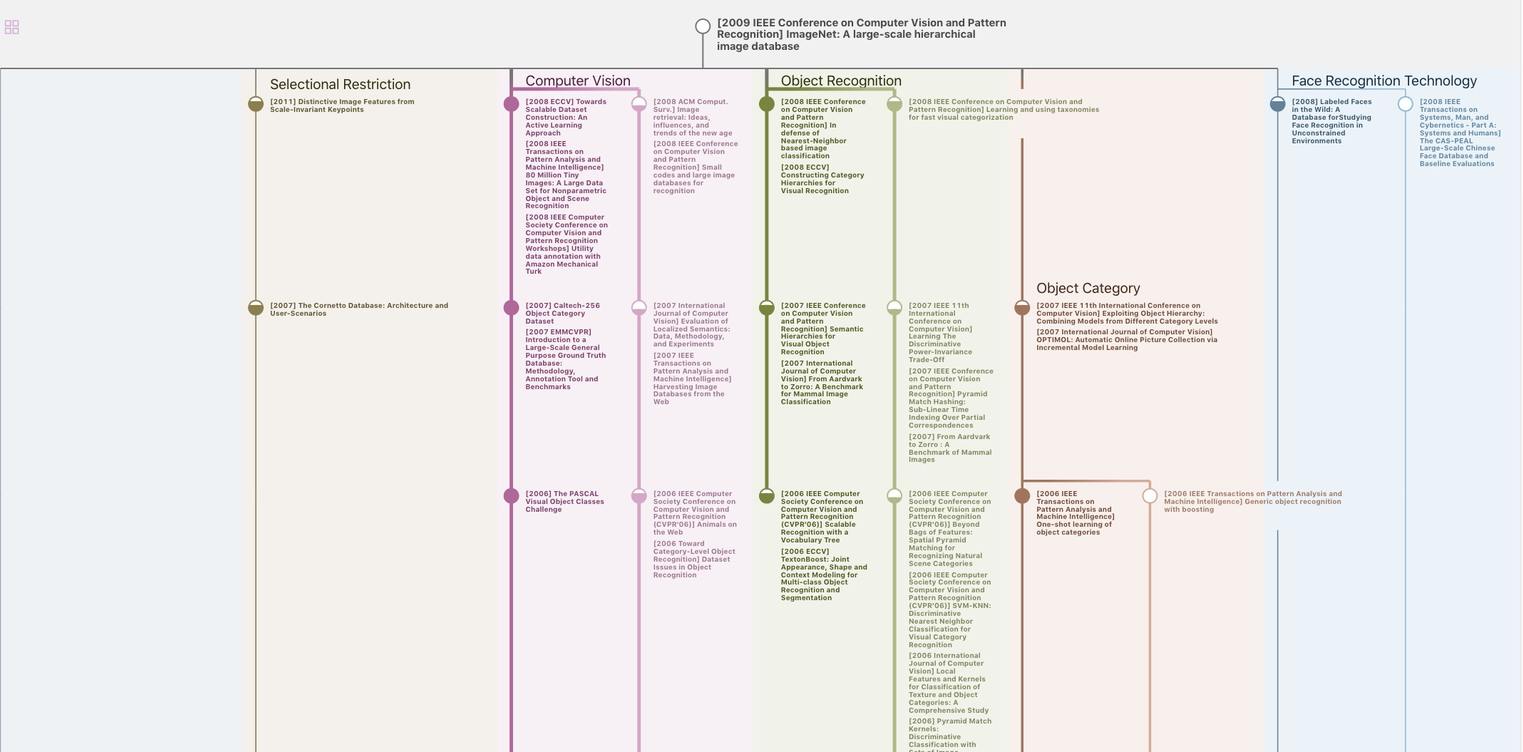
生成溯源树,研究论文发展脉络
Chat Paper
正在生成论文摘要