Overcoming far-end congestion in large-scale networks
HPCA(2015)
Abstract
Accurately estimating congestion for proper global adaptive routing decisions (i.e., determine whether a packet should be routed minimally or non-minimally) has a significant impact on overall performance for high-radix topologies, such as the Dragonfly topology. Prior work have focused on understanding near-end congestion - i.e., congestion that occurs at the current router - or downstream congestion - i.e., congestion that occurs in downstream routers. However, most prior work do not evaluate the impact of far-end congestion or the congestion from the high channel latency between the routers. In this work, we refer to far-end congestion as phantom congestion as the congestion is not "real" congestion. Because of the long inter-router latency, the in-flight packets (and credits) result in inaccurate congestion information and can lead to inaccurate adaptive routing decisions. In addition, we show how transient congestion occurs as the occupancy of network queues fluctuate due to random traffic variation, even in steady-state conditions. This also results in inaccurate adaptive routing decisions that degrade network performance with lower throughput and higher latency. To overcome these limitations, we propose a history-window based approach to remove the impact of phantom congestion. We also show how using the average of local queue occupancies and adding an offset significantly remove the impact of transient congestion. Our evaluations of the adaptive routing in a large-scale Dragonfly network show that the combination of these techniques results in an adaptive routing that nearly matches the performance of an ideal adaptive routing algorithm.
MoreTranslated text
Key words
multiprocessor interconnection networks,network routing,Dragonfly network,adaptive routing,far-end congestion,history-window based approach,in-flight packets,inter-router latency,interconnection networks,large-scale networks,network queues,phantom congestion,random traffic variation,steady-state conditions,transient congestion,
AI Read Science
Must-Reading Tree
Example
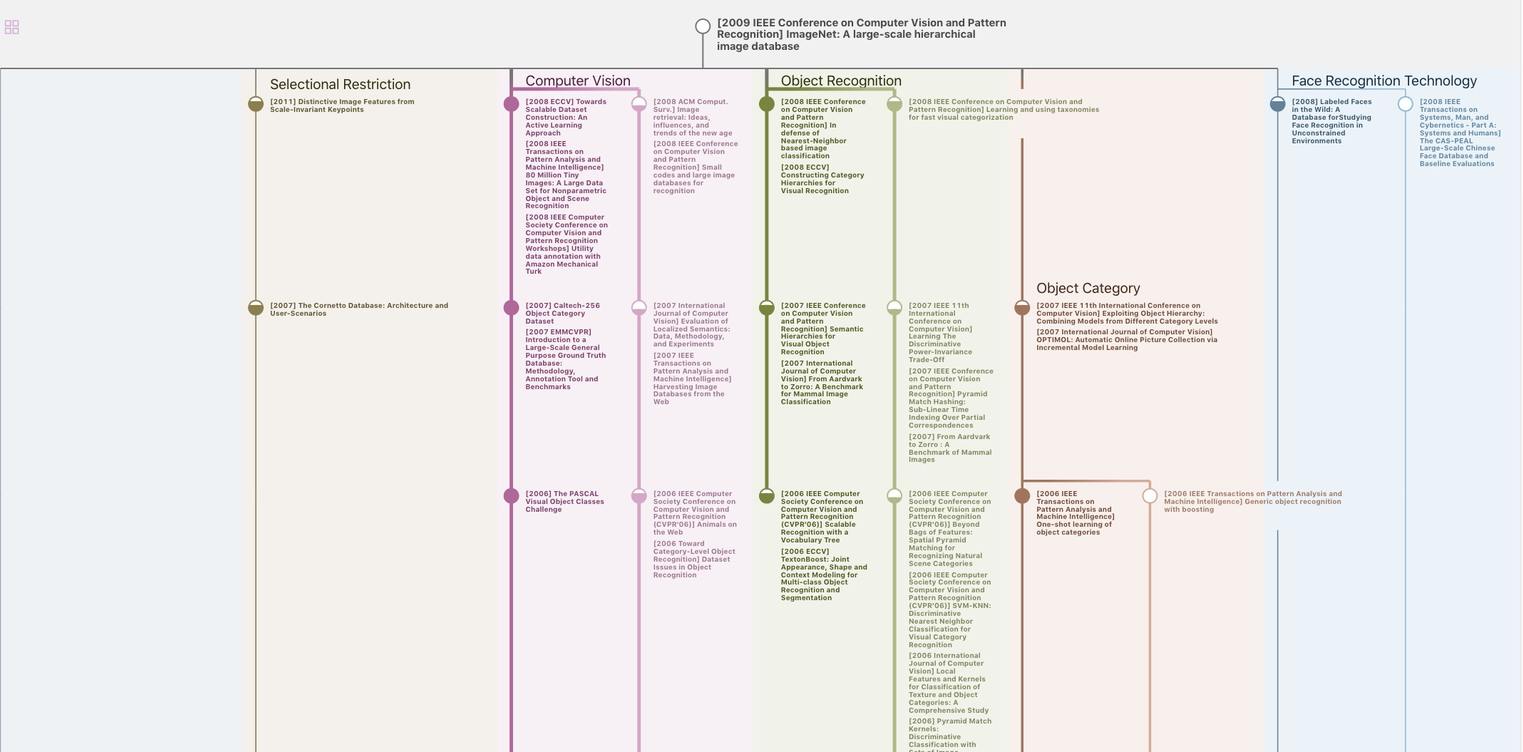
Generate MRT to find the research sequence of this paper
Chat Paper
Summary is being generated by the instructions you defined