Deciding when to stop: efficient experimentation to learn to predict drug-target interactions
BMC Bioinformatics(2015)
摘要
Active learning is a powerful tool for guiding an experimentation process. Instead of doing all possible experiments in a given domain, active learning can be used to pick the experiments that will add the most knowledge to the current model. Especially, for drug discovery and development, active learning has been shown to reduce the number of experiments needed to obtain high-confidence predictions. However, in practice, it is crucial to have a method to evaluate the quality of the current predictions and decide when to stop the experimentation process. Only by applying reliable stopping criteria to active learning can time and costs in the experimental process actually be saved.
更多查看译文
关键词
Active learning,Drug-target prediction,Simulation,Matrix factorization,Regression
AI 理解论文
溯源树
样例
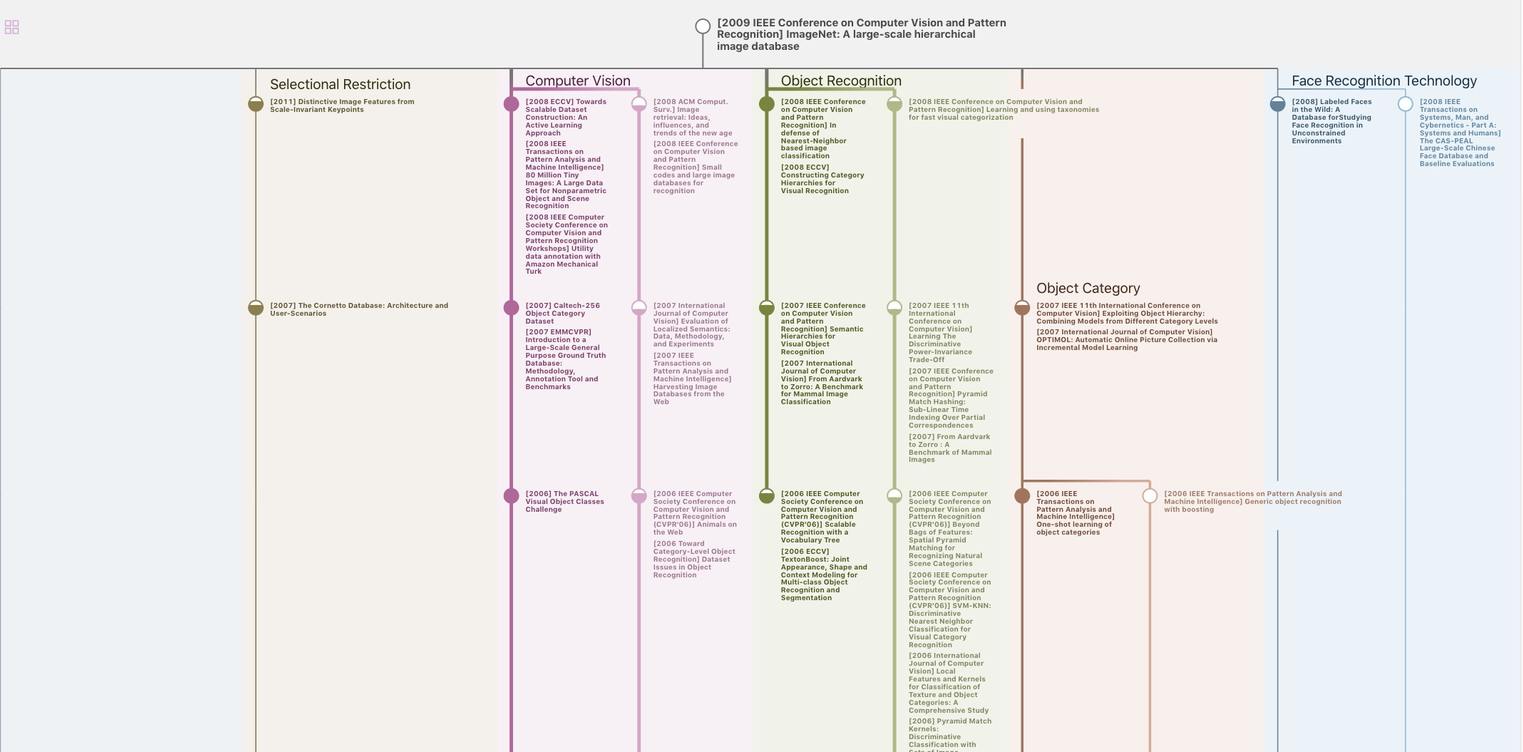
生成溯源树,研究论文发展脉络
Chat Paper
正在生成论文摘要