Propensity Score Matching for Causal Inference with Relational Data.
CI'14: Proceedings of the UAI 2014 Conference on Causal Inference: Learning and Prediction - Volume 1274(2014)
摘要
Propensity score matching (PSM) is a widely used method for performing causal inference with observational data. PSM requires fully specifying the set of confounding variables of treatment and outcome. In the case of relational data, this set may include non-intuitive relational variables, i.e., variables derived from the relational structure of the data. In this work, we provide an automated method to derive these relational variables based on the relational structure and a set of naive confounders. This automatic construction includes two unusual classes of variables: relational degree and entity identifiers. We provide experimental evidence that demonstrates the utility of these variables in accounting for certain latent confounders. Finally, through a set of synthetic experiments, we show that our method improves the performance of PSM for causal inference with relational data.
更多查看译文
AI 理解论文
溯源树
样例
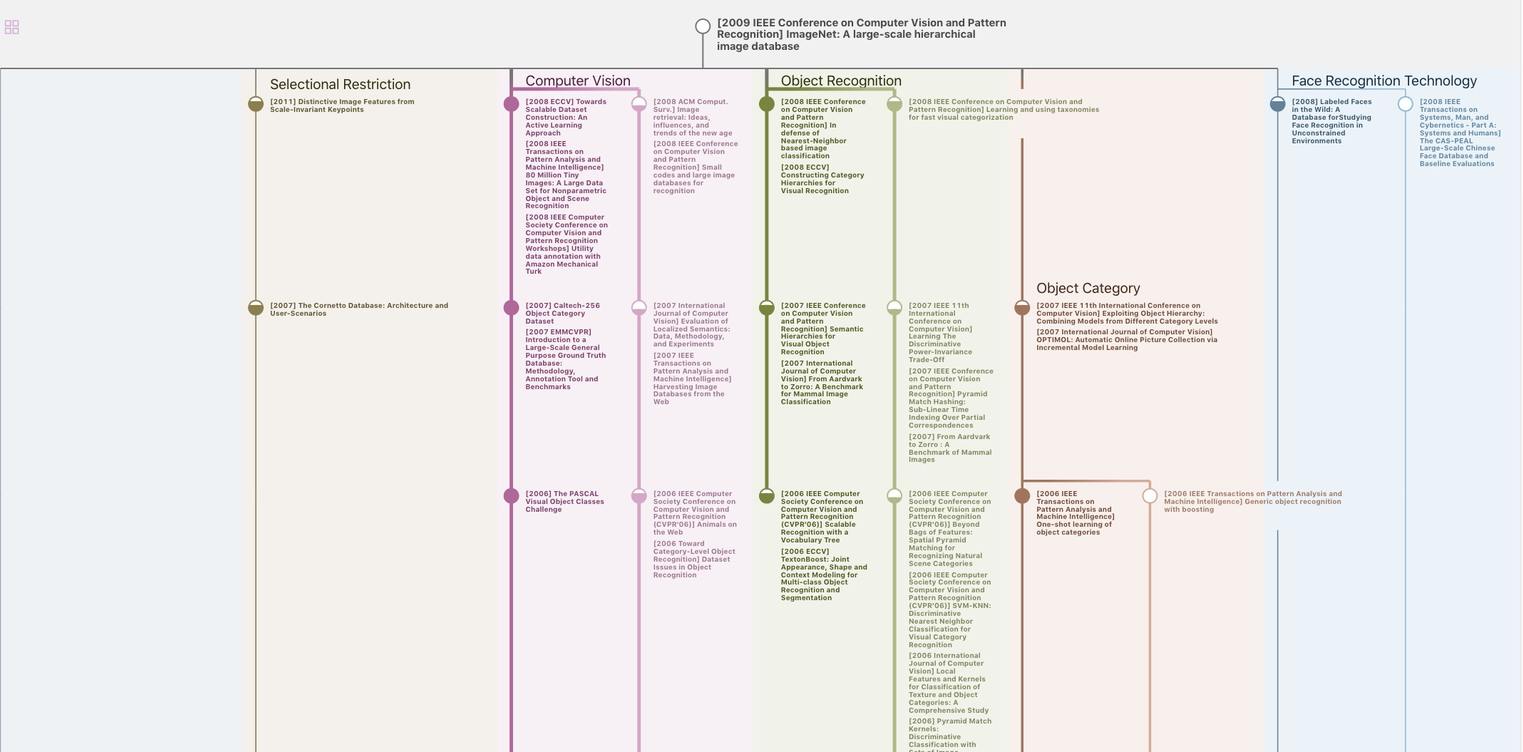
生成溯源树,研究论文发展脉络
Chat Paper
正在生成论文摘要