Patient-specific semi-supervised learning for postoperative brain tumor segmentation.
Lecture Notes in Computer Science(2014)
Abstract
In contrast to preoperative brain tumor segmentation, the problem of postoperative brain tumor segmentation has been rarely approached so far. We present a fully-automatic segmentation method using multimodal magnetic resonance image data and patient-specific semisupervised learning. The idea behind our semi-supervised approach is to effectively fuse information from both pre-and postoperative image data of the same patient to improve segmentation of the postoperative image. We pose image segmentation as a classification problem and solve it by adopting a semi-supervised decision forest. The method is evaluated on a cohort of 10 high-grade glioma patients, with segmentation performance and computation time comparable or superior to a state-of-the-art brain tumor segmentation method. Moreover, our results confirm that the inclusion of preoperative MR images lead to a better performance regarding postoperative brain tumor segmentation.
MoreTranslated text
AI Read Science
Must-Reading Tree
Example
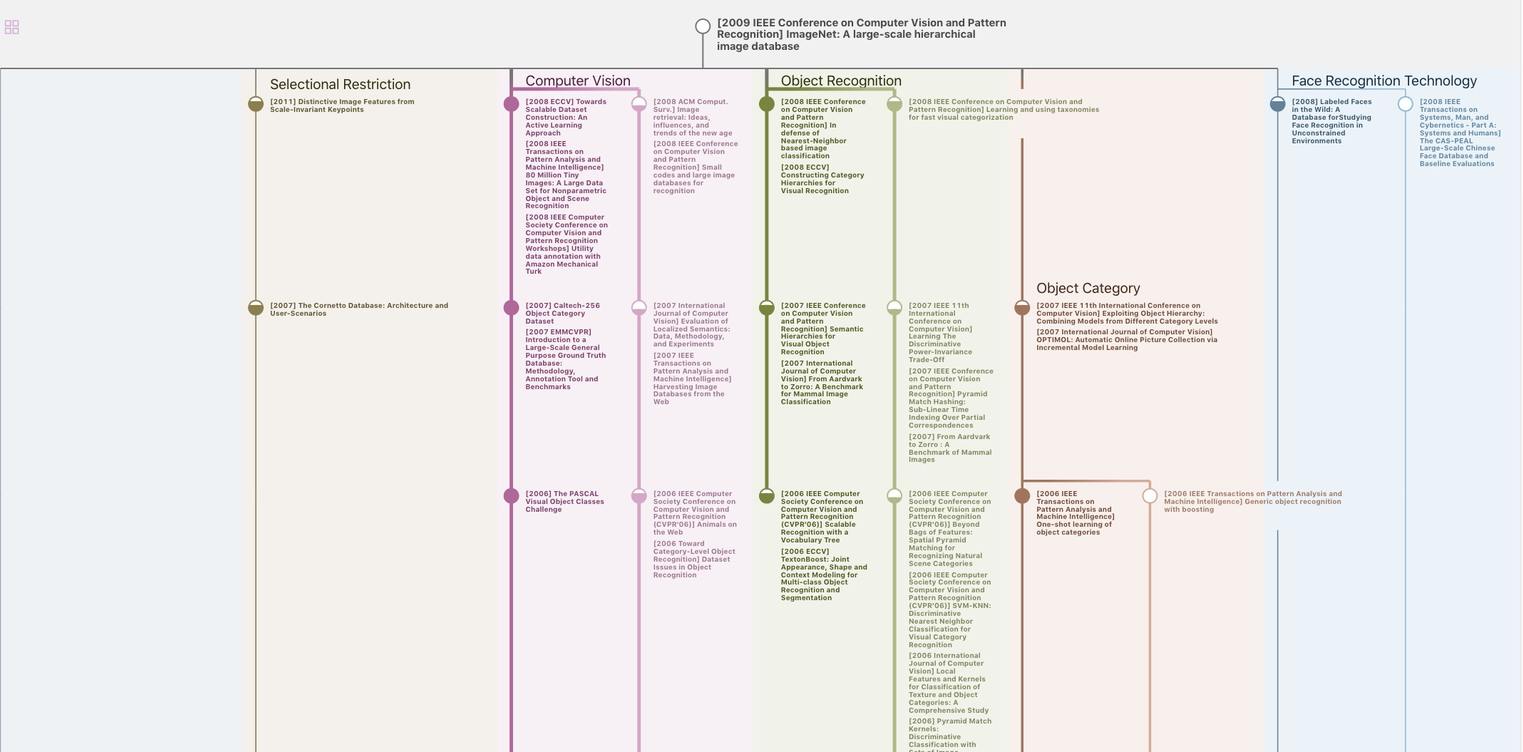
Generate MRT to find the research sequence of this paper
Chat Paper
Summary is being generated by the instructions you defined