Supervised Semantic Indexing Using Sub-spacing.
ICCBR(2014)
摘要
Indexing of textual cases is commonly affected by the problem of variation in vocabulary. Semantic indexing is commonly used to address this problem by discovering semantic or conceptual relatedness between individual terms and using this to improve textual case representation. However, representations produced using this approach are not optimal for supervised tasks because standard semantic indexing approaches do not take into account class membership of these textual cases. Supervised semantic indexing approaches e.g. sprinkled Latent Semantic Indexing (SpLSI) and supervised Latent Dirichlet Allocation (sLDA) have been proposed for addressing this limitation. However, both SpLSI and sLDA are computationally expensive and require parameter tuning. In this work, we present an approach called Supervised Sub-Spacing (S3) for supervised semantic indexing of documents. S3 works by creating a separate sub-space for each class within which class-specific term relations and term weights are extracted. The power of S3 lies in its ability to modify document representations such that documents that belong to the same class are made more similar to one another while, at the same time, reducing their similarity to documents of other classes. In addition, S3 is flexible enough to work with a variety of semantic relatedness metrics and yet, powerful enough that it leads to significant improvements in text classification accuracy. We evaluate our approach on a number of supervised datasets and results show classification performance on S3-based representations to significantly outperform both a supervised version of Latent Semantic Indexing (LSI) called Sprinkled LSI, and supervised LDA.
更多查看译文
关键词
Textual case-based reasoning,textual case representation,semantic indexing,supervised semantic indexing
AI 理解论文
溯源树
样例
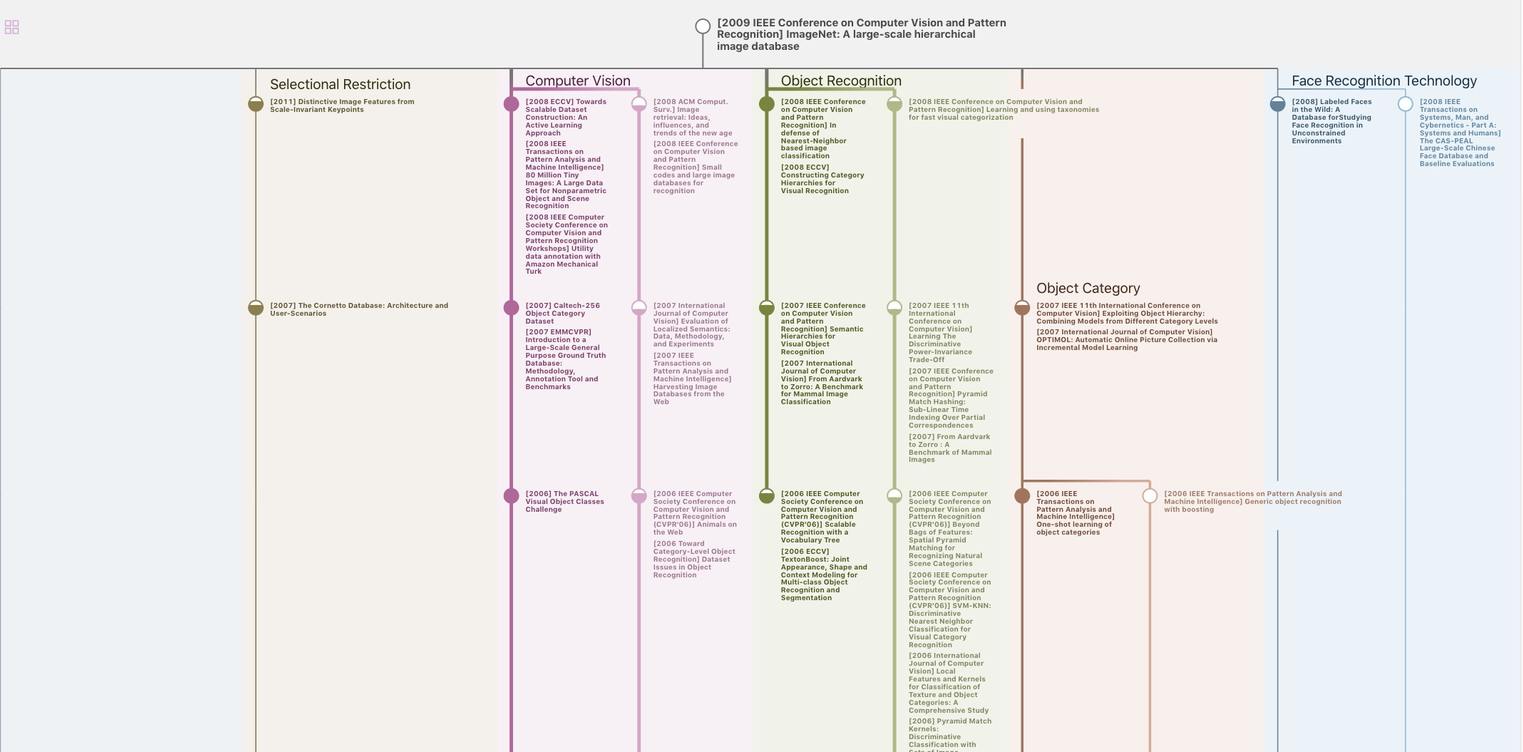
生成溯源树,研究论文发展脉络
Chat Paper
正在生成论文摘要