Classifier-Adjusted Density Estimation for Anomaly Detection and One-Class Classification.
SDM(2014)
摘要
Previous chapter Next chapter Full AccessProceedings Proceedings of the 2014 SIAM International Conference on Data Mining (SDM)Classifier-Adjusted Density Estimation for Anomaly Detection and One-Class ClassificationLisa Friedland, Amanda Gentzel, and David JensenLisa FriedlandSchool of Computer Science, University of Massachusetts Amherst.School of Computer Science, University of Massachusetts Amherst.School of Computer Science, University of Massachusetts Amherst.Search for more papers by this author, Amanda GentzelSchool of Computer Science, University of Massachusetts Amherst.School of Computer Science, University of Massachusetts Amherst.School of Computer Science, University of Massachusetts Amherst.Search for more papers by this author, and David JensenSchool of Computer Science, University of Massachusetts Amherst.School of Computer Science, University of Massachusetts Amherst.School of Computer Science, University of Massachusetts Amherst.Search for more papers by this authorpp.578 - 586Chapter DOI:https://doi.org/10.1137/1.9781611973440.67PDFBibTexSections ToolsAdd to favoritesDownload CitationsTrack CitationsEmail SectionsAboutAbstract Density estimation methods are often regarded as unsuitable for anomaly detection in high-dimensional data due to the difficulty of estimating multivariate probability distributions. Instead, the scores from popular distance- and local-density-based methods, such as local outlier factor (LOF), are used as surrogates for probability densities. We question this infeasibility assumption and explore a family of simple statistically-based density estimates constructed by combining a probabilistic classifier with a naive density estimate. Across a number of semi-supervised and unsupervised problems formed from real-world data sets, we show that these methods are competitive with LOF and that even simple density estimates that assume attribute independence can perform strongly. We show that these density estimation methods scale well to data with high dimensionality and that they are robust to the problem of irrelevant attributes that plagues methods based on local estimates. Previous chapter Next chapter RelatedDetails Published:2014eISBN:978-1-61197-344-0 https://doi.org/10.1137/1.9781611973440Book Series Name:ProceedingsBook Code:PRDT14Book Pages:1-1086
更多查看译文
关键词
anomaly detection,classification,density,classifier-adjusted,one-class
AI 理解论文
溯源树
样例
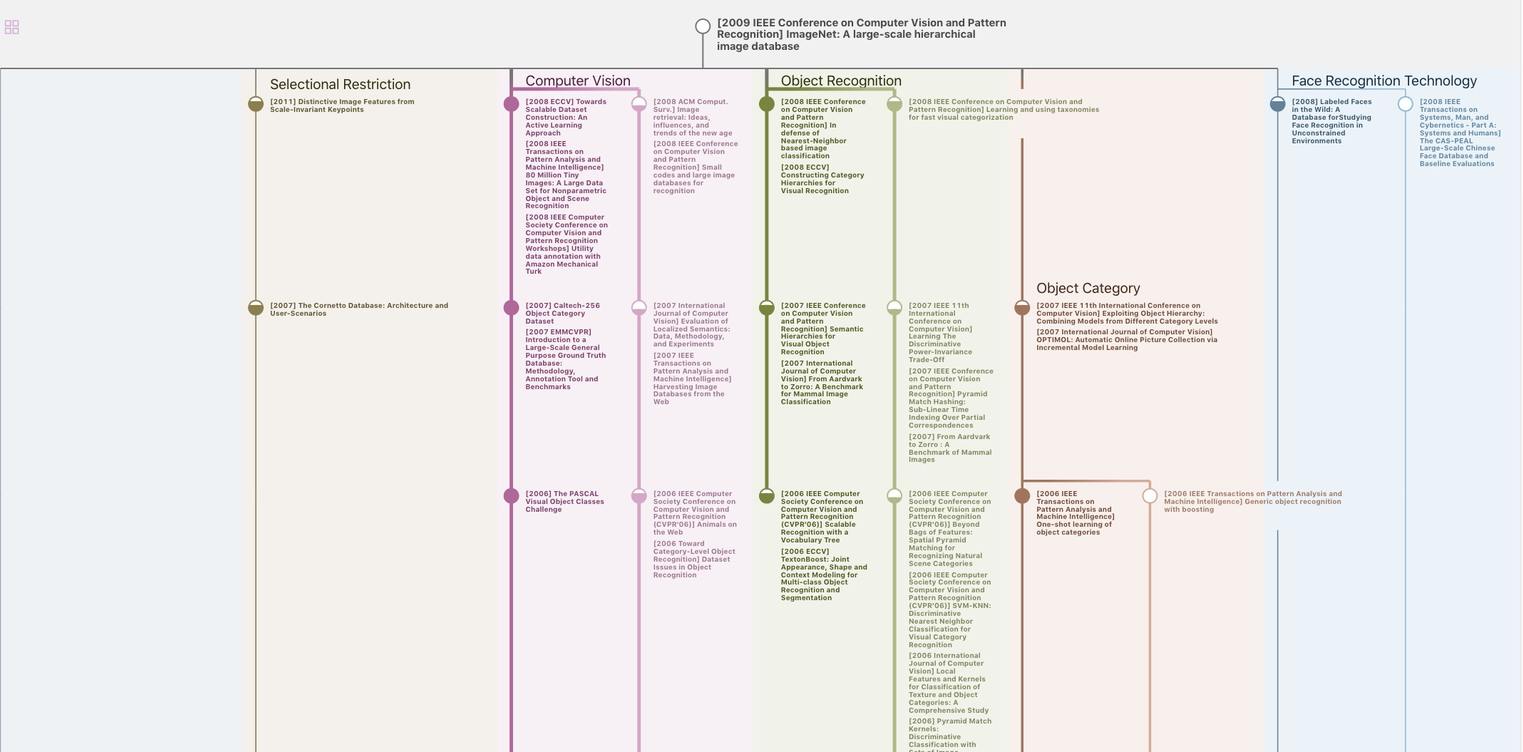
生成溯源树,研究论文发展脉络
Chat Paper
正在生成论文摘要