MiL testing of highly configurable continuous controllers: scalable search using surrogate models.
ASE(2014)
摘要
ABSTRACTContinuous controllers have been widely used in automotive domain to monitor and control physical components. These controllers are subject to three rounds of testing: Model-in-the-Loop (MiL), Software-in-the-Loop and Hardware-in-the-Loop. In our earlier work, we used meta-heuristic search to automate MiL testing of fixed configurations of continuous controllers. In this paper, we extend our work to support MiL testing of all feasible configurations of continuous controllers. Specifically, we use a combination of dimensionality reduction and surrogate modeling techniques to scale our earlier MiL testing approach to large, multi-dimensional input spaces formed by configuration parameters. We evaluated our approach by applying it to a complex, industrial continuous controller. Our experiment shows that our approach identifies test cases indicating requirements violations. Further, we demonstrate that dimensionally reduction helps generate surrogate models with higher prediction accuracy. Finally, we show that combining our search algorithm with surrogate modelling improves its efficiency for two out of three requirements.
更多查看译文
AI 理解论文
溯源树
样例
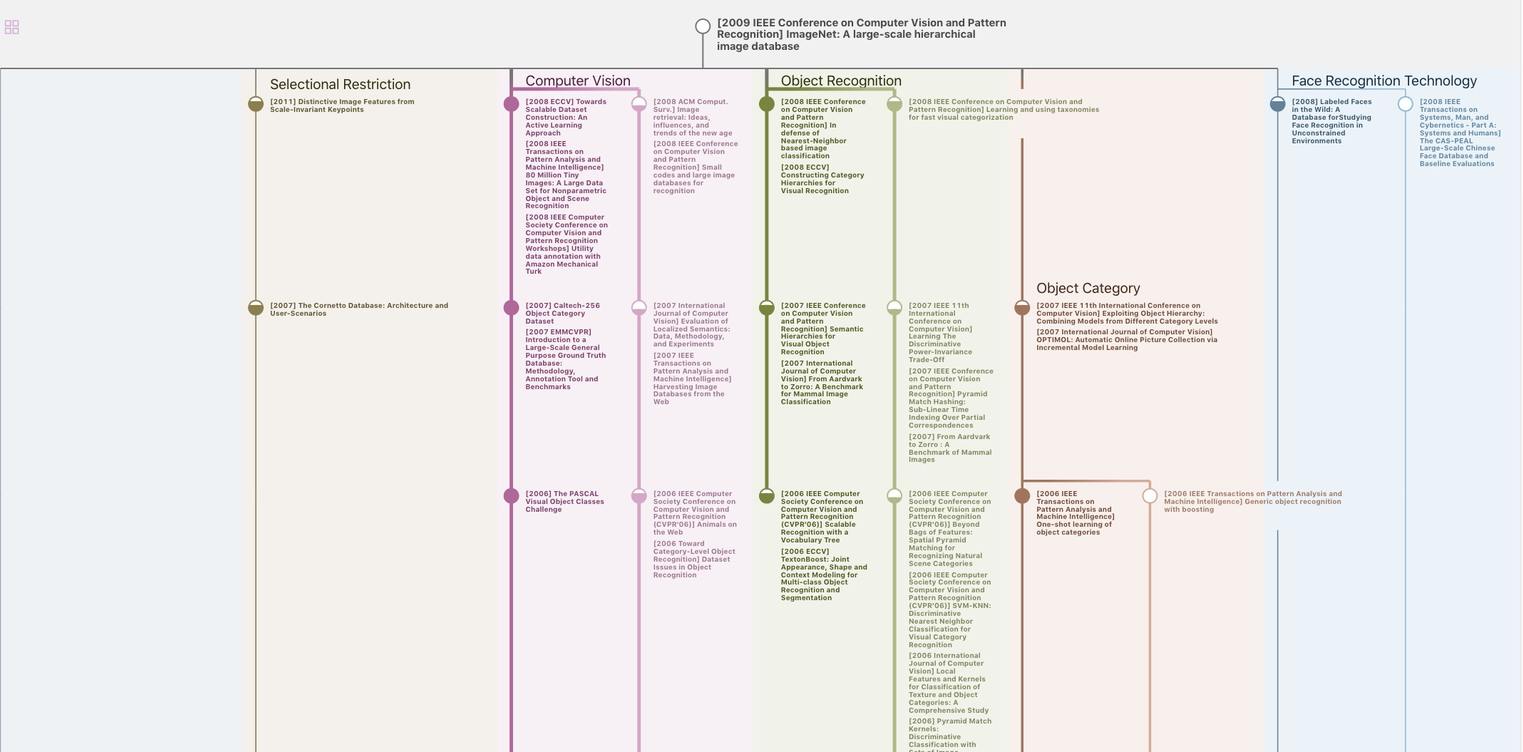
生成溯源树,研究论文发展脉络
Chat Paper
正在生成论文摘要