Interrogative-guided re-ranking for question-oriented software text retrieval.
ASE(2014)
摘要
ABSTRACTIn many software engineering tasks, question-oriented text retrieval is often used to help developers search for software artifacts. In this paper, we propose an interrogative-guided re-ranking approach for question-oriented software text retrieval. Since different interrogatives usually indicate users' different search focuses, we firstly label 9 kinds of question-answer pairs according to the common interrogatives. Then, we train document classifiers by using 1,826 questions along with 2,460 answers from StackOverflow, apply the classifiers to our document repository and present a re-ranking approach to improve the retrieval precision. In software document classification, our classifiers achieve the average precision, recall and F-measure of 56.2%, 90.9% and 69.4% respectively. Our re-ranking approach presents 9.6% improvement in [email protected] upon the baseline, and we also obtain 8.1% improvement in [email protected] when more candidates are included.
更多查看译文
AI 理解论文
溯源树
样例
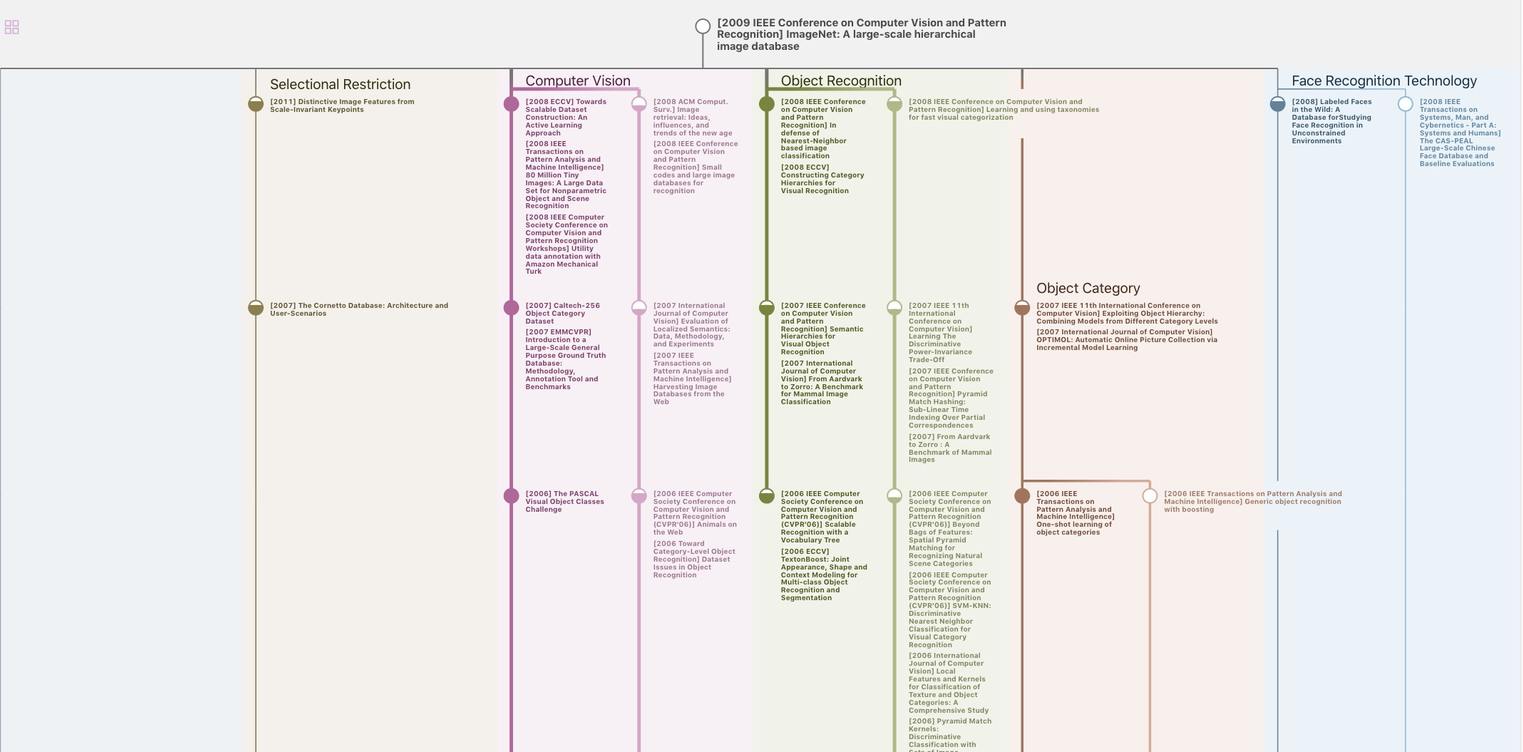
生成溯源树,研究论文发展脉络
Chat Paper
正在生成论文摘要