Contextual modeling content-based approaches for new-item recommendation
IIiX(2014)
摘要
The new-item cold-start problem is a well-known limitation of context-free and context-aware Collaborative Filtering (CF) prediction models. In such situations, only Content-based (CB) approaches can produce meaningful recommendations. In this paper, we propose three Context-Aware Content-Based (CACB) models that extend a linear CB prediction model with context-awareness by including additional parameters that represent the influence of context with respect to the users' interests and rating behaviour. The precision of the proposed models has been evaluated using a contextually-tagged rating data set for journey plans in the city of Barcelona (Spain), which has a high number of new items. We demonstrate that, in this data set, the most sophisticated CACB model, which exploits the contextual information at different granularities and also the distributional similarities between contextual conditions during user modeling, significantly outperforms a context-free CB model as well as a state-of-the-art context-aware approach.
更多查看译文
关键词
algorithms,cold-start problem,content-based filtering,context-aware recommender systems,contextual modelling,experimentation,information filtering,performance
AI 理解论文
溯源树
样例
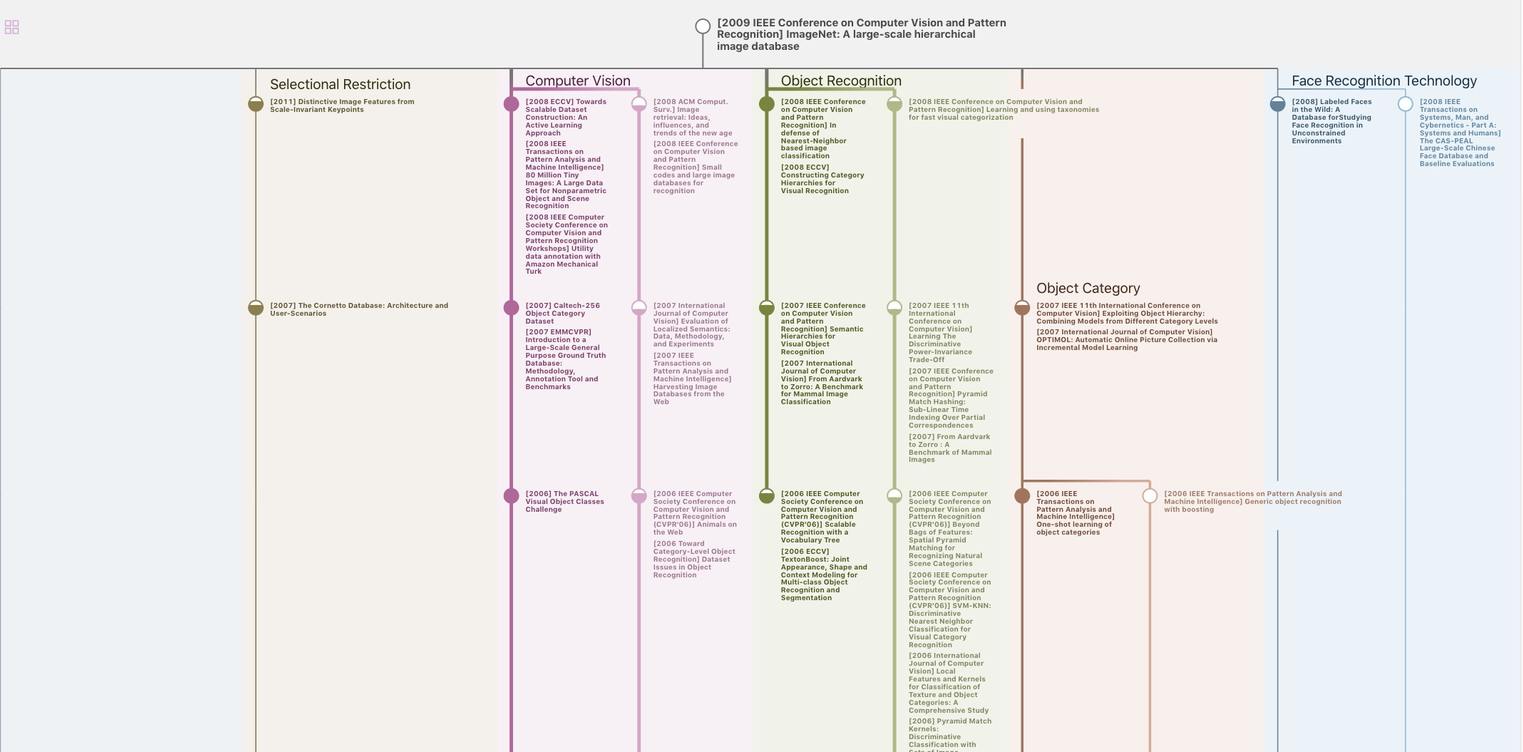
生成溯源树,研究论文发展脉络
Chat Paper
正在生成论文摘要