Learning Spatio-Temporal Features For Action Recognition With Modified Hidden Conditional Random Field
COMPUTER VISION - ECCV 2014 WORKSHOPS, PT I(2014)
摘要
Previous work on human action analysis mainly focuses on designing hand-crafted local features and combining their context information. In this paper, we propose using supervised feature learning as a way to learn spatio-temporal features. More specifically, a modified hidden conditional random field is applied to learn two high-level features conditioned on a certain action label. Among them, the individual features can describe the appearance of local parts and the interaction features can capture their spatial constraints. In order to make the best of what have been learned, a new categorization model is proposed for action matching. It is inspired by the Deformable Part Model and the intuition is that actions can be modeled by local features in a changeable spatial and temporal dependency. Experimental result shows that our algorithm can successfully recognize human actions with high accuracies both on the simple atomic action database (KTH and Weizmann) and complex interaction activity database (CASIA).
更多查看译文
关键词
Human action recognition, Spatio-temporal features, HCRF, Changeable spatial-temporal constraint model (CSTCM), Feature learning
AI 理解论文
溯源树
样例
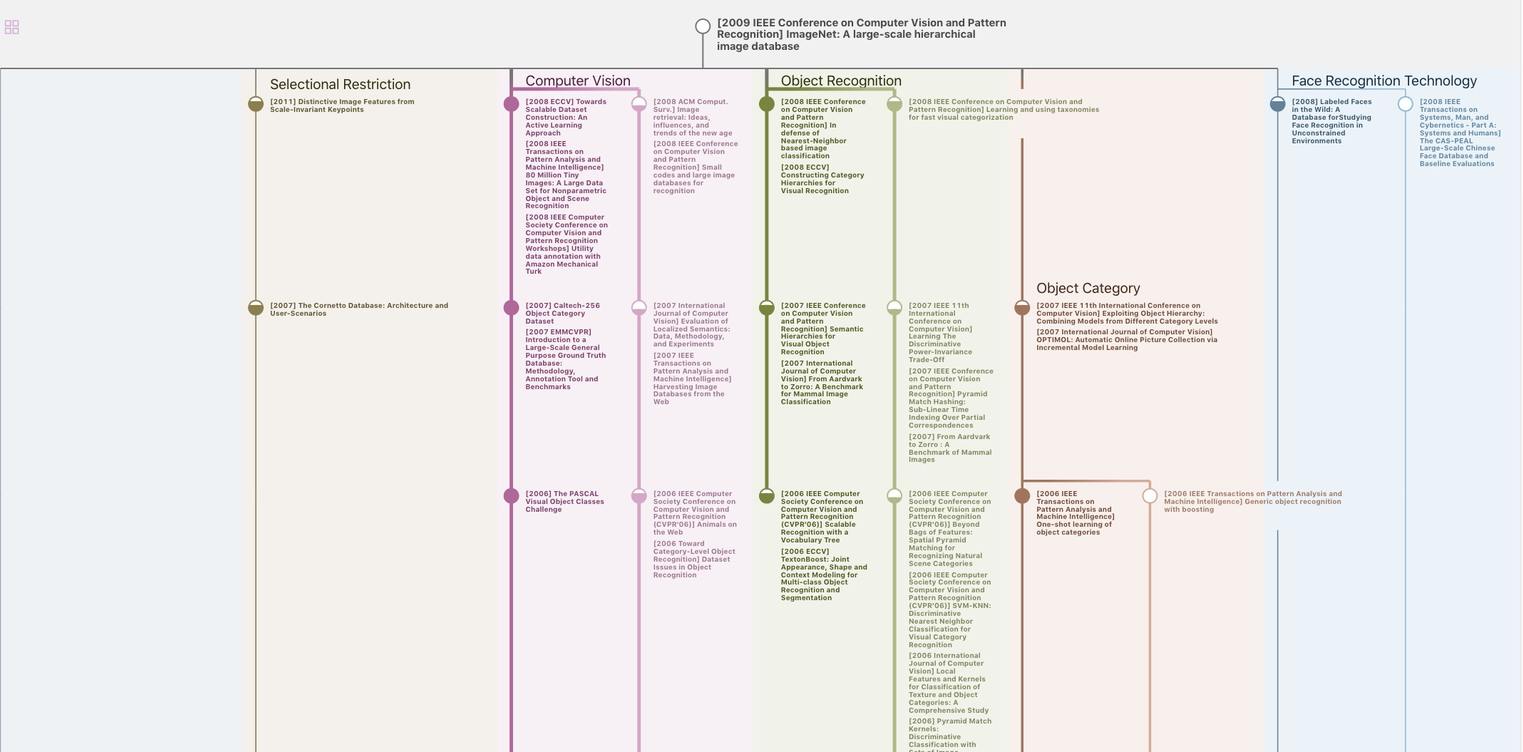
生成溯源树,研究论文发展脉络
Chat Paper
正在生成论文摘要