Preprocessing For Classification Of Sparse Data: Application To Trajectory Recognition
2012 IEEE STATISTICAL SIGNAL PROCESSING WORKSHOP (SSP)(2012)
摘要
On one hand, sparse coding, which is widely used in signal processing, consists of representing signals as linear combinations of few elementary patterns selected from a dedicated dictionary. The output is a sparse vector containing few coding coefficients and is called sparse code. On the other hand, Multilayer Perceptron (MLP) is a neural network classification method that learns non linear borders between classes using labeled data examples. The MLP input data are vectors, usually normalized and preprocessed to minimize the inter-class correlation. This article acts as a link between sparse coding and MLP by converting sparse code into convenient vectors for MLP input. This original association assures in this way the classification of any sparse signals. Experimental results obtained by the whole process on trajectories data and comparisons to other methods show that this approach is efficient for signals classification.
更多查看译文
关键词
Sparse coding,classification,multilayer perceptron,trajectories data
AI 理解论文
溯源树
样例
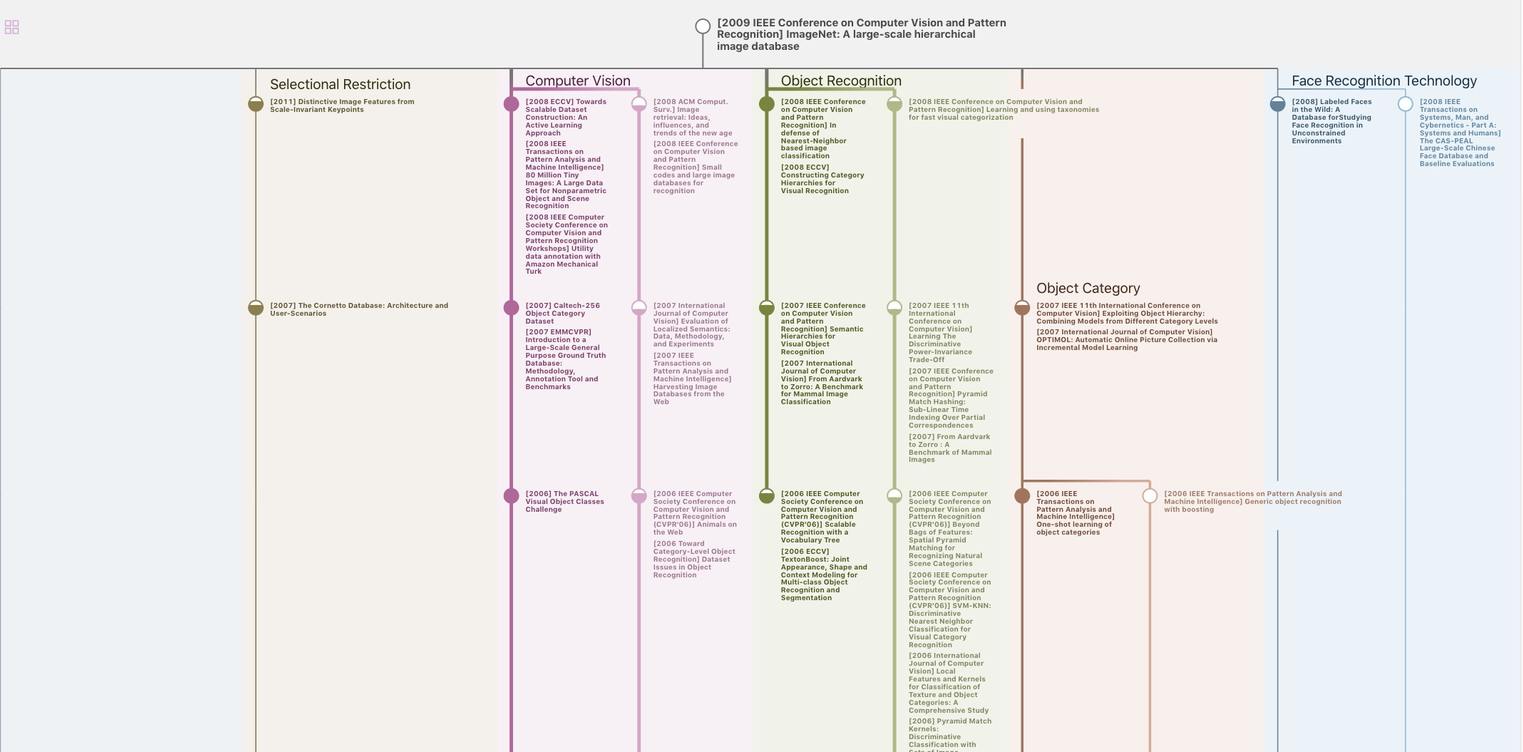
生成溯源树,研究论文发展脉络
Chat Paper
正在生成论文摘要