Query difficulty estimation via pseudo relevance feedback for image search
ICME(2014)
摘要
Query difficulty estimation (QDE) attempts to automatically predict the performance of the search results returned for a given query. QDE has been widely investigated in text document retrieval for many years. However, few research works have been explored in image retrieval. State-of-the-art QDE methods in image retrieval mainly investigate the statistical characteristics (coherence, robustness, etc.) of the returned images to derive a value for indicating the query difficulty degree. To the best of our knowledge, little research has been done to directly estimate the real retrieval performance of the search results, such as average precision, instead of only an indicator. In this paper, we propose a novel query difficulty estimation approach which automatically estimate the average precision of the image search results. Specifically, we first select a set of query relevant and query irrelevant images for each query via pseudo relevance feedback. Then an efficient and effective voting scheme is proposed to estimate the relevance label of each image in the search results. Based on the images' relevance labels, the average precision of the search results returned for the given query is derived. The experimental results on a benchmark image search dataset demonstrate the effectiveness of the proposed method.
更多查看译文
关键词
query relevant images,image search dataset,statistical analysis,qde method,text document retrieval,query irrelevant images,pseudo relevance feedback (prf),image retrieval,statistical characteristics,voting scheme,average precision (ap),relevance feedback,query difficulty estimation (qde),query difficulty estimation approach,pseudo relevance feedback,visualization,correlation,search engines,mathematical model,estimation
AI 理解论文
溯源树
样例
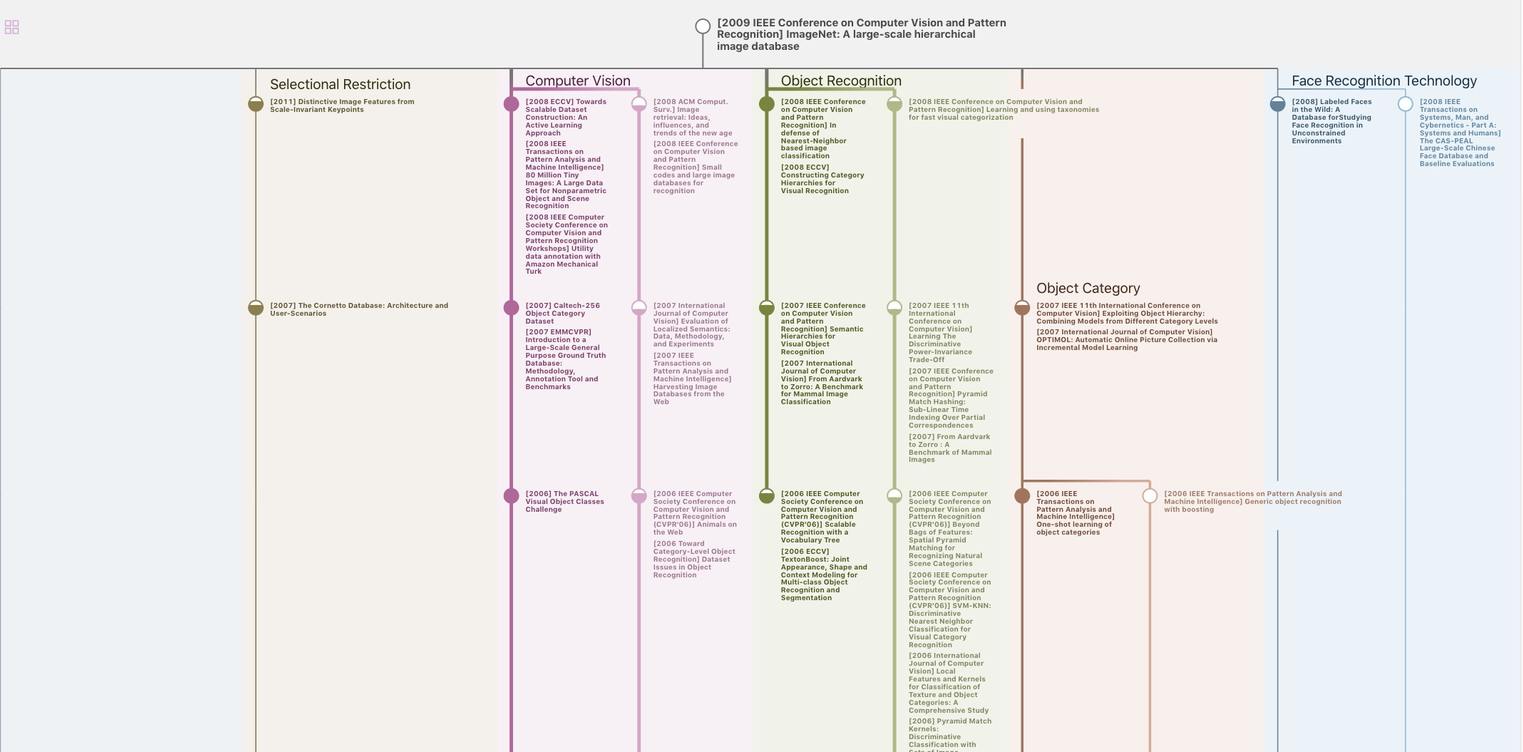
生成溯源树,研究论文发展脉络
Chat Paper
正在生成论文摘要