Mptm: A Topic Model For Multi-Part Documents
DATABASE SYSTEMS FOR ADVANCED APPLICATIONS, DASFAA 2015, PT II(2015)
摘要
Topic models have been successfully applied to uncover hidden probabilistic structures in collections of documents, where documents are treated as unstructured texts. However, it is not uncommon that some documents, which we call multi-part documents, are composed of multiple named parts. To exploit the information buried in the document-part relationships in the process of topic modeling, this paper adopts two assumptions: the first is that all parts in a given document should have similar topic distributions, and the second is that the multiple versions (corresponding to multiple named parts) of a given topic should have similar word distributions. Based on these two underlying assumptions, we propose a novel topic model for multi-part documents, called Multi-Part Topic Model (or MPTM in short), and develop its construction and inference method with the aid of the techniques of collapsed Gibbs sampling and maximum likelihood estimation. Experimental results on real datasets demonstrate that our approach has not only achieved significant improvement on the qualities of discovered topics, but also boosted the performance in information retrieval and document classification.
更多查看译文
关键词
Topic models, Gibbs sampling, Maximum likelihood estimation
AI 理解论文
溯源树
样例
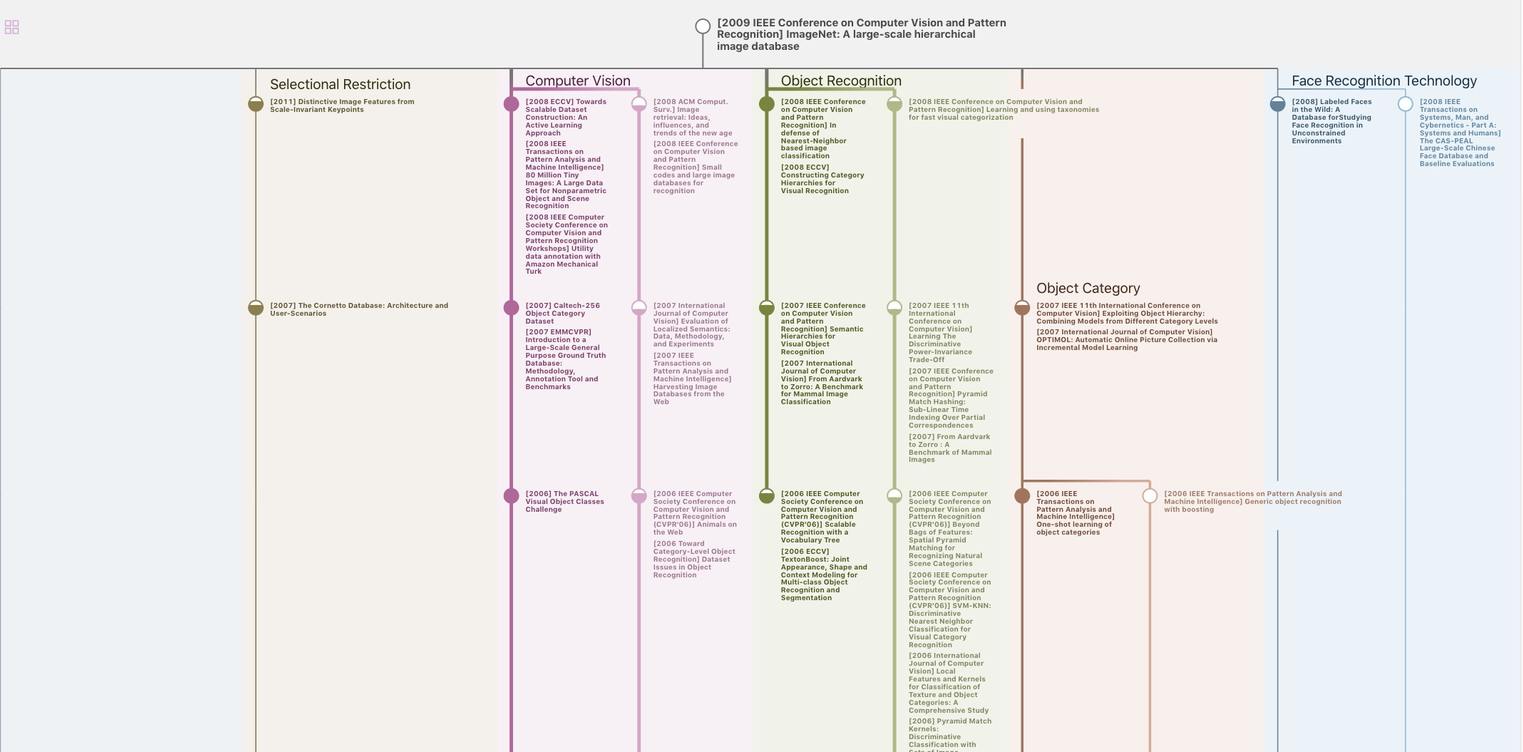
生成溯源树,研究论文发展脉络
Chat Paper
正在生成论文摘要