Integrating Motivated Learning and k-Winner-Take-All to Coordinate Multi-agent Reinforcement Learning.
WI-IAT (3)(2014)
摘要
This work addresses the coordination issue in distributed optimization problem (DOP) where multiple distinct and time-critical tasks are performed to satisfy a global objective function. The performance of these tasks has to be coordinated due to the sharing of consumable resources and the dependency on non-consumable resources. Knowing that it can be suboptimal to predefine the performance of the tasks for large DOPs, the multi-agent reinforcement learning (MARL) framework is adopted wherein an agent is used to learn the performance of each distinct task using reinforcement learning. To coordinate MARL, we propose a novel coordination strategy integrating Motivated Learning (ML) and the k-Winner-Take-All (k-WTA) approach. The priority of the agents to the shared resources is determined using Motivated Learning in real time. Due to the finite amount of the shared resources, the k-WTA approach is used to allow for the maximum number of the most urgent tasks to execute. Agents performing tasks dependent on resources produced by other agents are coordinated using domain knowledge. Comparing our proposed contribution to the existing approaches, results from our experiments based on a 16-task DOP and a 68-task DOP show our proposed approach to be most effective in coordinating multi-agent reinforcement learning.
更多查看译文
关键词
learning artificial intelligence,games,multi agent system,reinforcement learning,linear programming,optimization,resource management
AI 理解论文
溯源树
样例
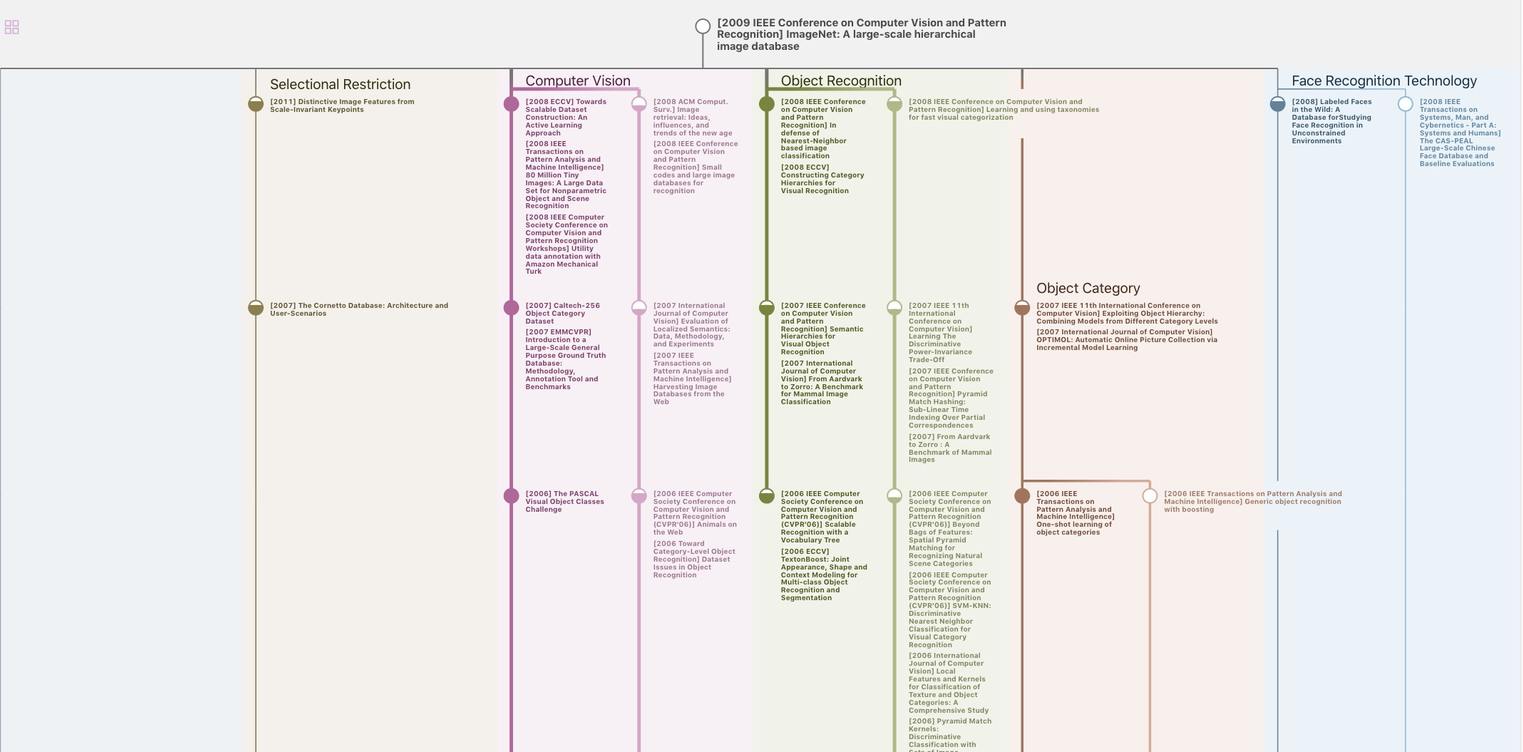
生成溯源树,研究论文发展脉络
Chat Paper
正在生成论文摘要