Gender identification in unconstrained scenarios using Self-Similarity of Gradients features
2014 IEEE International Conference on Image Processing, ICIP 2014(2014)
摘要
Gender identification has been a hot research topic with wide application requirements from social life. In general, effective feature representation is the key to solving this problem. In this paper, a new feature named Self-Similarity of Gradients (GSS) is proposed, which captures pairwise statistics of localized gradient distributions. There are three contributions made by us to practical gender identification. First, GSS features are proposed for gender identification in the wild, which achieve good performance compared with baseline approaches. Second, we originally utilize 31-dimensional HOG for practical gender identification and its excellent results demonstrates that HOG with both contrast sensitive and insensitive information is a better fit for this topic than that with only contrast insensitive information. Last, feature combination and multi-classifier combination strategies are adopted and the best gender identification performance is achieved. Experimental results show that the combination of GSS, HOG and LBP using a linear SVM outperforms state-of-the-art on the LFW database, which meets the 'wild' condition. © 2014 IEEE.
更多查看译文
关键词
AdaBoost,Histogram of Oriented Gradients,Labeled Faces in the Wild,Self-Similarity of Gradients,SVM
AI 理解论文
溯源树
样例
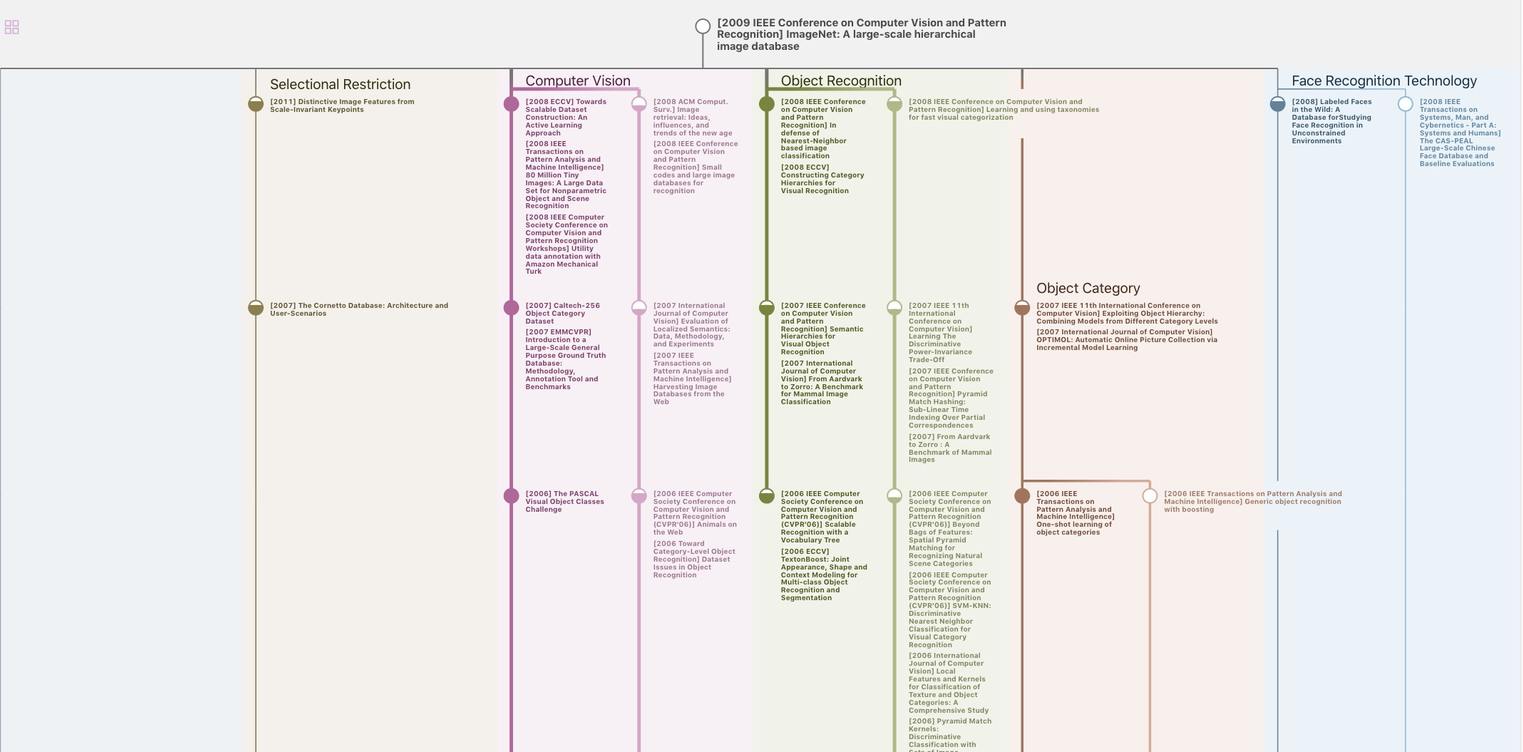
生成溯源树,研究论文发展脉络
Chat Paper
正在生成论文摘要