GMM-based saliency aggregation for calibration-free gaze estimation
ICIP(2014)
摘要
A typical gaze estimator needs an explicit personal calibration stage with many discrete fixation points. This limitation can be resolved by mapping multiple eye images and corresponding saliency maps of a video clip during an implicit calibration stage. Compared to previous calibration-free methods, our approach clusters eye images by using Gaussian Mixture Model (GMM) in order to increase calibration accuracy and reduce training redundancy. Eye feature vectors representing eye images undergo soft clustering with GMM as well as the corresponding saliency maps for aggregation. The GMM based soft-clustering boosts the accuracy of Gaussian process regression which maps between eye feature vectors and gaze directions given this constructed data. The experimental results show an increase in gaze estimation accuracy compared to previous works on calibration-free method.
更多查看译文
关键词
discrete fixation points,video signal processing,calibration,saliency,soft clusters eye images,eye feature vectors,training redundancy reduction,data construction,video clip,gaze tracking,estimation theory,mixture models,gaze estimation,gaussian process regression,real-time free head pose gaze tracking system,gmm-based saliency aggregation,gaze directions,gaussian processes,multiple eye image mapping,calibration-free gaze estimation,saliency maps,gaussian mixture model,personal implicit calibration stage accuracy
AI 理解论文
溯源树
样例
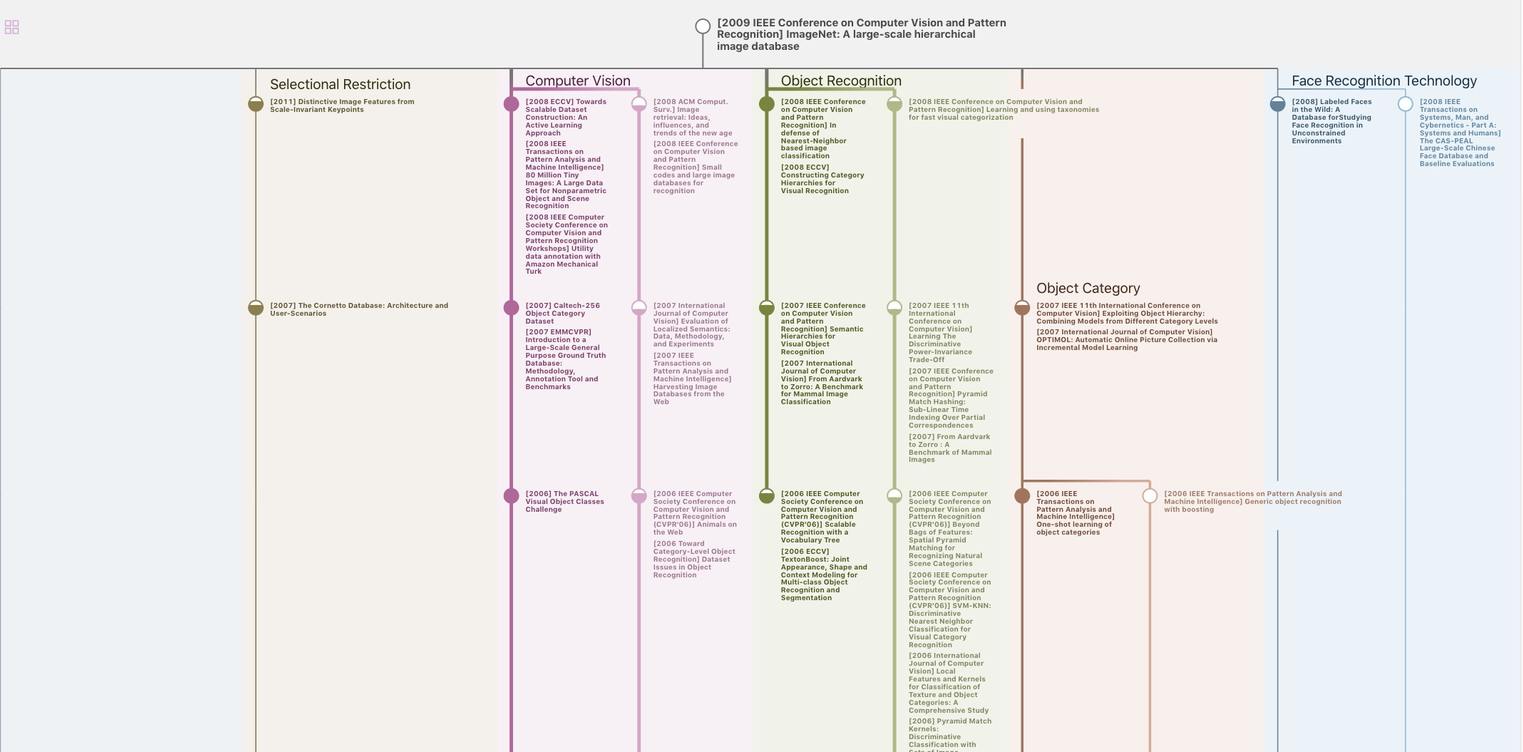
生成溯源树,研究论文发展脉络
Chat Paper
正在生成论文摘要