A constrained-based optimization approach for seismic data recovery problems
ICASSP(2014)
摘要
Random and structured noise both affect seismic data, hiding the reflections of interest (primaries) that carry meaningful geophysical interpretation. When the structured noise is composed of multiple reflections, its adaptive cancellation is obtained through time-varying filtering, compensating inaccuracies in given approximate templates. The under-determined problem can then be formulated as a convex optimization one, providing estimates of both filters and primaries. Within this framework, the criterion to be minimized mainly consists of two parts: a data fidelity term and hard constraints modeling a priori information. This formulation may avoid, or at least facilitate, some parameter determination tasks, usually difficult to perform in inverse problems. Not only classical constraints, such as sparsity, are considered here, but also constraints expressed through hyperplanes, onto which the projection is easy to compute. The latter constraints lead to improved performance by further constraining the space of geophysically sound solutions.
更多查看译文
关键词
optimisation,seismic data recovery problems,optimization methods,data fidelity term,seismology,adaptive filters,inverse problems,hard constraints modeling,adaptive filtering,geophysical signal processing,time-varying filtering,filtering theory,constrained-based optimization approach,time-varying filters,wavelet transforms,signal restoration,convex optimization,noise,indexes,estimation,geophysics,convex functions
AI 理解论文
溯源树
样例
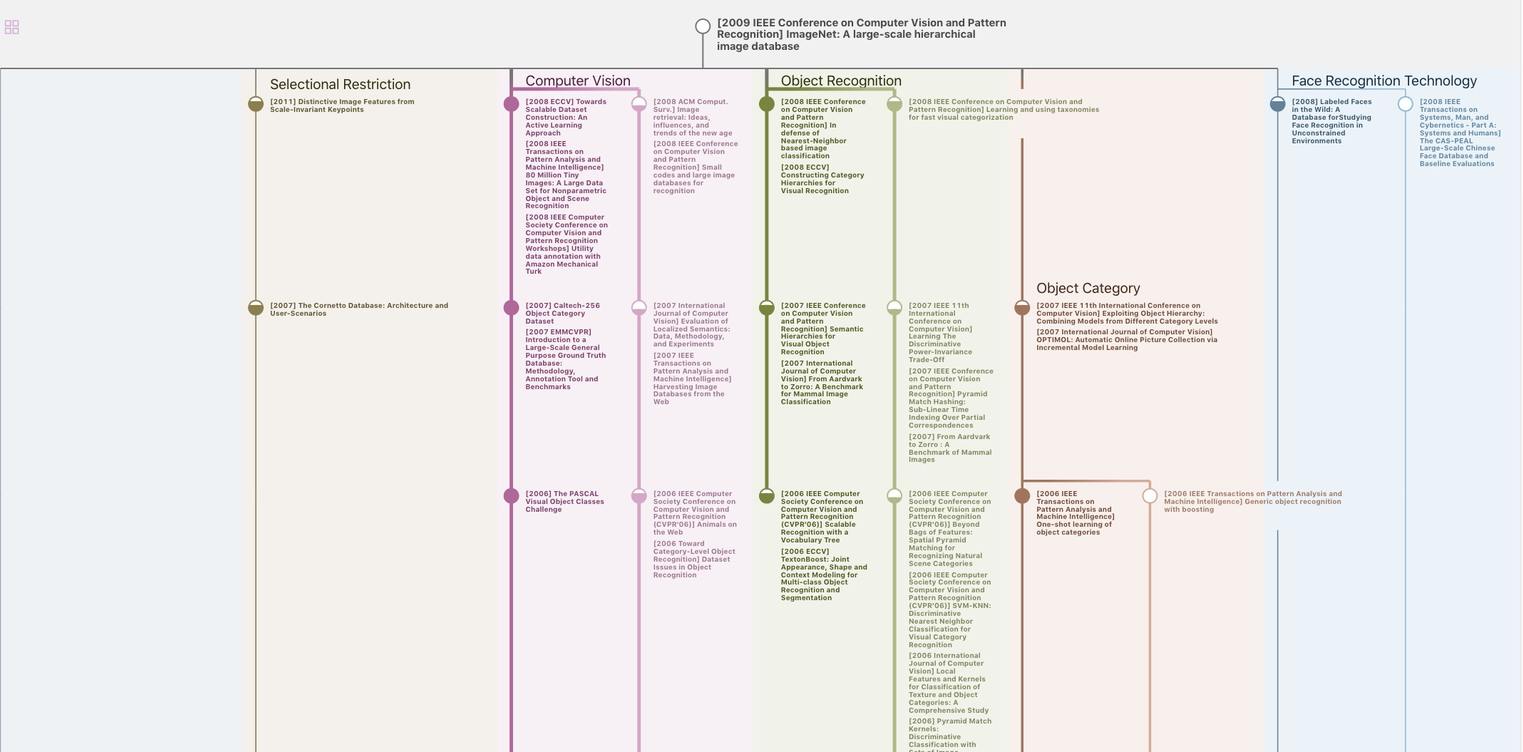
生成溯源树,研究论文发展脉络
Chat Paper
正在生成论文摘要