An energy-efficient VLSI architecture for pattern recognition via deep embedding of computation in SRAM
ICASSP(2014)
摘要
In this paper, we propose the concept of compute memory, where computation is deeply embedded into the memory (SRAM). This deep embedding enables multi-row read access and analog signal processing. Compute memory exploits the relaxed precision and linearity requirements of pattern recognition applications. System-level simulations incorporating various deterministic errors from analog signal chain demonstrates the limited accuracy of analog processing does not significantly degrade the system performance, which means the probability of pattern detection is minimally impacted. The estimated energy saving is 63 % as compared to the conventional system with standard embedded memory and parallel processing architecture, for 256×256 target image.
更多查看译文
关键词
machine learning,linearity requirements,deterministic errors,signal processing,pattern recognition,deep embedding,compute memory,analog processing,relaxed precision,system level simulations,sram chips,energy-efficient vlsi architecture,associative memory,analog signal processing,analog signal chain,vlsi,sram,pattern detection,multirow read access,probability
AI 理解论文
溯源树
样例
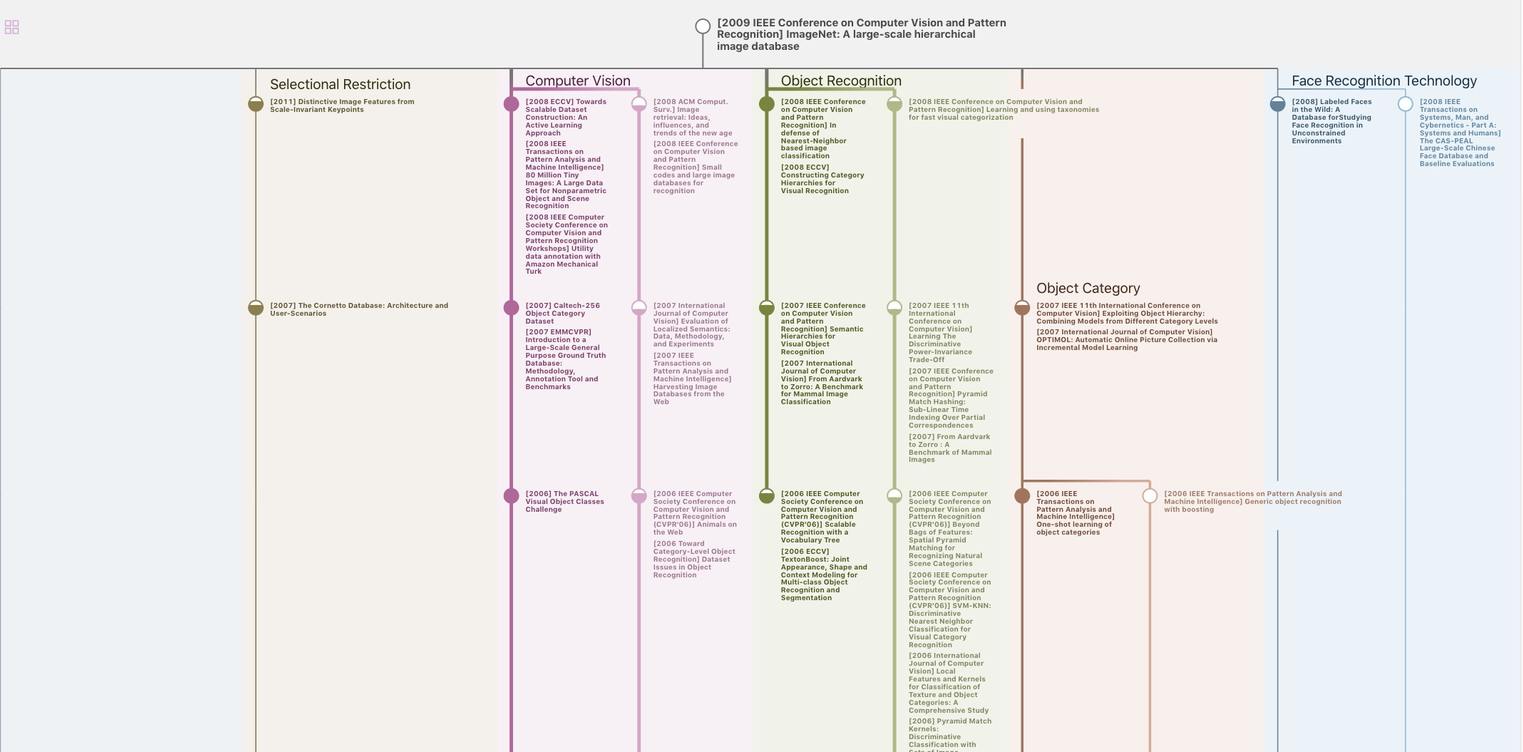
生成溯源树,研究论文发展脉络
Chat Paper
正在生成论文摘要