Super Normal Vector for Activity Recognition Using Depth Sequences
CVPR(2014)
摘要
This paper presents a new framework for human activity recognition from video sequences captured by a depth camera. We cluster hypersurface normals in a depth sequence to form the polynormal which is used to jointly characterize the local motion and shape information. In order to globally capture the spatial and temporal orders, an adaptive spatio-temporal pyramid is introduced to subdivide a depth video into a set of space-time grids. We then propose a novel scheme of aggregating the low-level polynormals into the super normal vector (SNV) which can be seen as a simplified version of the Fisher kernel representation. In the extensive experiments, we achieve classification results superior to all previous published results on the four public benchmark datasets, i.e., MSRAction3D, MSRDailyActivity3D, MSRGesture3D, and MSRActionPairs3D.
更多查看译文
关键词
video signal processing,motion information,video sequences,shape information,super normal vector,adaptive spatio-temporal pyramid,depth sequences,spatiotemporal phenomena,snv,human activity recognition,image sequences,space-time grids,vectors,encoding,trajectory,visualization,dictionaries
AI 理解论文
溯源树
样例
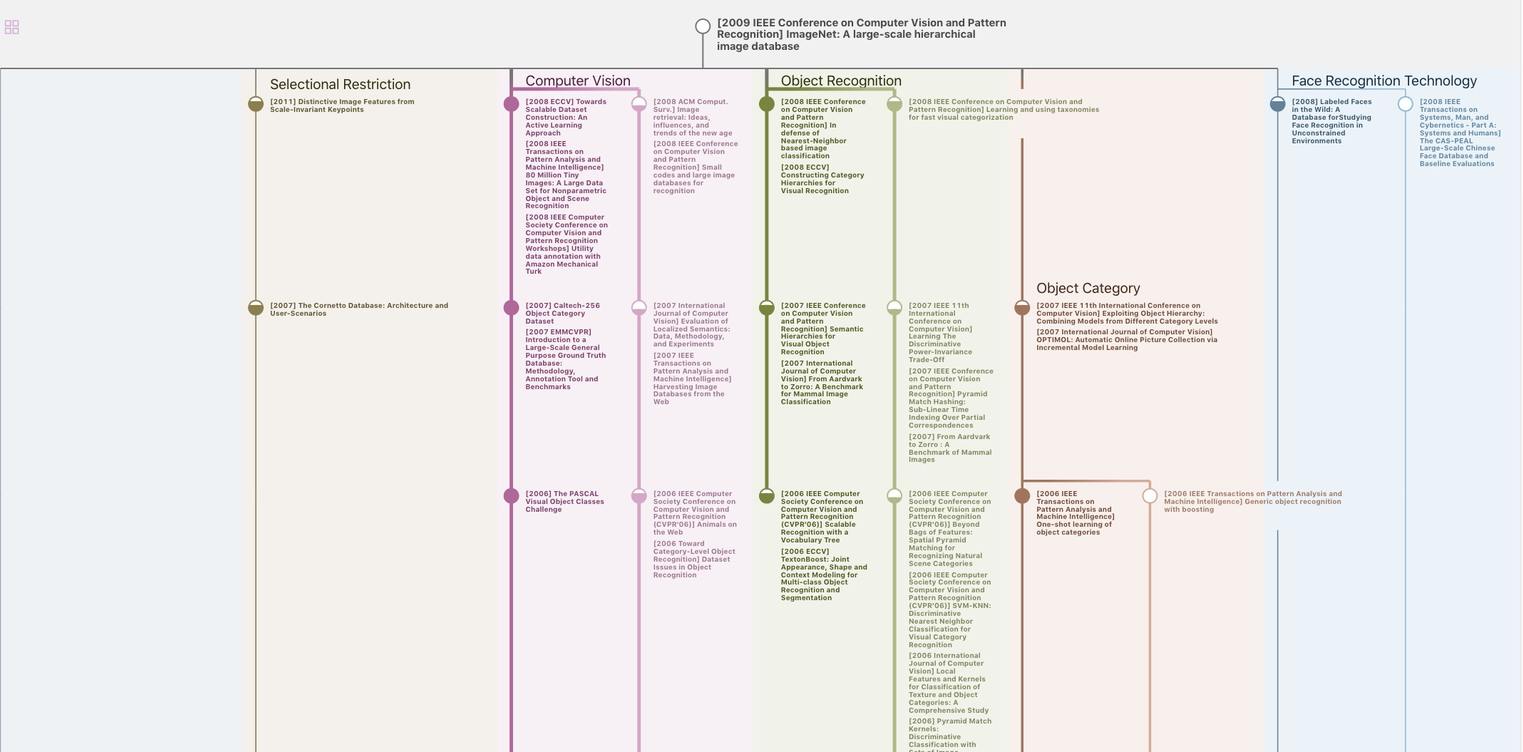
生成溯源树,研究论文发展脉络
Chat Paper
正在生成论文摘要