Pedestrian Detection in Low-Resolution Imagery by Learning Multi-scale Intrinsic Motion Structures (MIMS)
CVPR(2014)
Abstract
Detecting pedestrians at a distance from large-format wide-area imagery is a challenging problem because of low ground sampling distance (GSD) and low frame rate of the imagery. In such a scenario, the approaches based on appearance cues alone mostly fail because pedestrians are only a few pixels in size. Frame-differencing and optical flow based approaches also give poor detection results due to noise, camera jitter and parallax in aerial videos. To overcome these challenges, we propose a novel approach to extract Multi-scale Intrinsic Motion Structure features from pedestrian's motion patterns for pedestrian detection. The MIMS feature encodes the intrinsic motion properties of an object, which are location, velocity and trajectory-shape invariant. The extracted MIMS representation is robust to noisy flow estimates. In this paper, we give a comparative evaluation of the proposed method and demonstrate that MIMS outperforms the state of the art approaches in identifying pedestrians from low resolution airborne videos.
MoreTranslated text
Key words
low resolution imagery,pedestrians,video signal processing,pedestrian detection,image resolution,aerial videos,pedestrian motion patterns,low ground sampling distance,large format wide area imagery,multiscale intrinsic motion structure features,intrinsic motion properties,trajectory shape invariant,mims feature,low frame rate,optical flow,parallax,gsd,low resolution airborne videos,mims representation,comparative evaluation,frame differencing,noisy flow estimates,camera jitter,feature extraction,noise,shape,trajectory
AI Read Science
Must-Reading Tree
Example
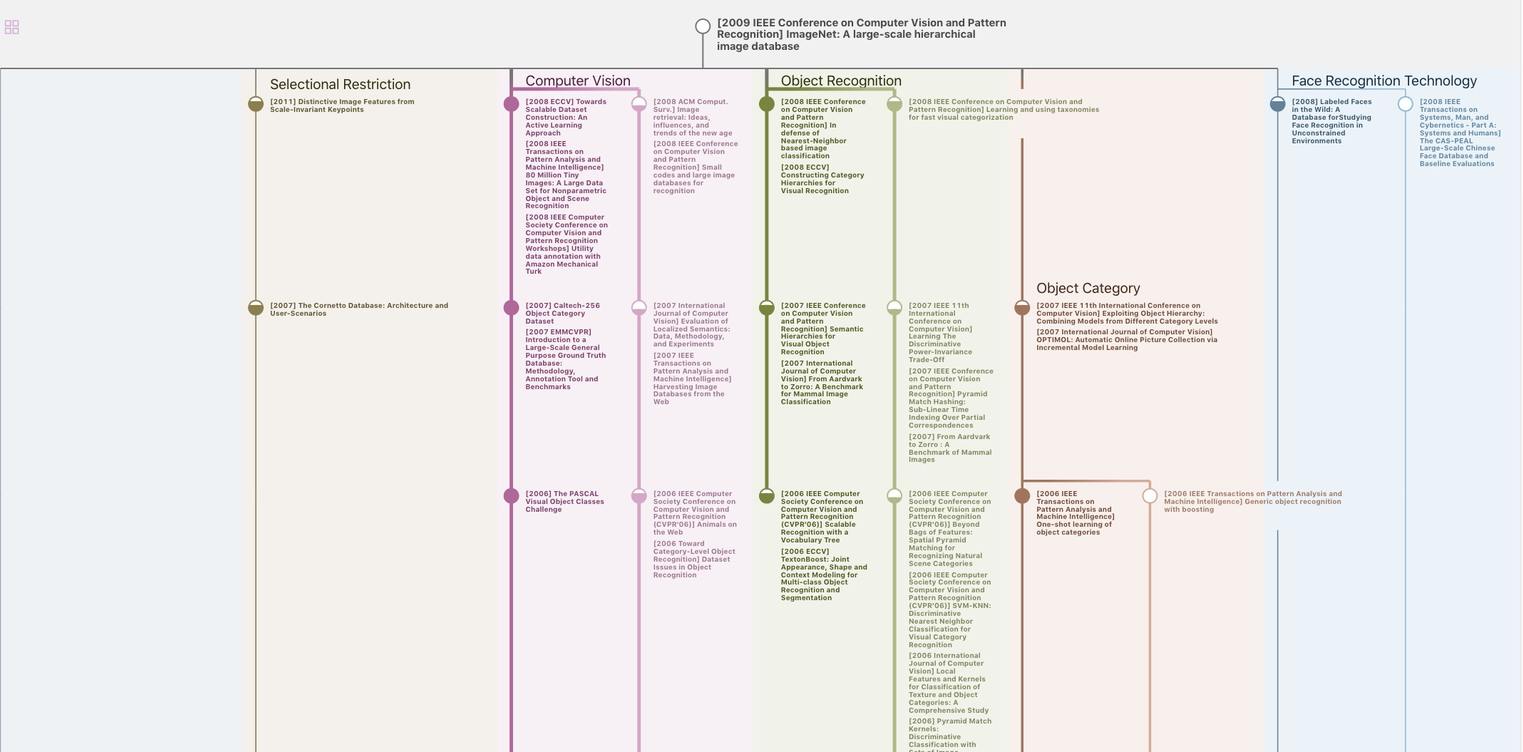
Generate MRT to find the research sequence of this paper
Chat Paper
Summary is being generated by the instructions you defined