What Videos Are Similar With You? Learning A Common Attributed Representation For Video Recommendation
MM '14: 2014 ACM Multimedia Conference Orlando Florida USA November, 2014(2014)
摘要
Although video recommender systems have become the predominant way for people to obtain video information, their performances are far from satisfactory in that (1) the recommended videos are often mismatched with the users' interests and (2) the recommendation results are, in most cases, hardly understandable for users and therefore cannot persuade them to engage. In this paper, we attempt to address the above problems in data representation level, and aim to learn a common attributed representation for users and videos in social media with good interpretability, stability and an appropriate level of granularity. The basic idea is to represent videos with users' social attributes, and represent users with content attributes of videos, such that both users and videos can be represented in a common space concatenated by social attributes and content attributes. The video recommendation problem can then be converted into a similarity matching problem in the common space. However, it is still challenging to balance the roles of social attributes and content attributes, learn such a common representation in sparse user-video interactions and deal with the cold-start problem. In this paper, we propose a REgularized Dual-fActor Regression (REDAR) method based on matrix factorization. In this method, social attributes and content attributes are flexibly combined, and social and content information are effectively exploited to alleviate the sparsity problem. An incremental version of REDAR is designed to solve the cold-start problem. We extensively evaluate the proposed method for video recommendation application in real social network dataset, and the results show that, in most cases, the proposed method can achieve a relative improvement of more than 20% compared to state-of-the-art baseline methods.
更多查看译文
关键词
video recommendation,attributed representation,social attributes
AI 理解论文
溯源树
样例
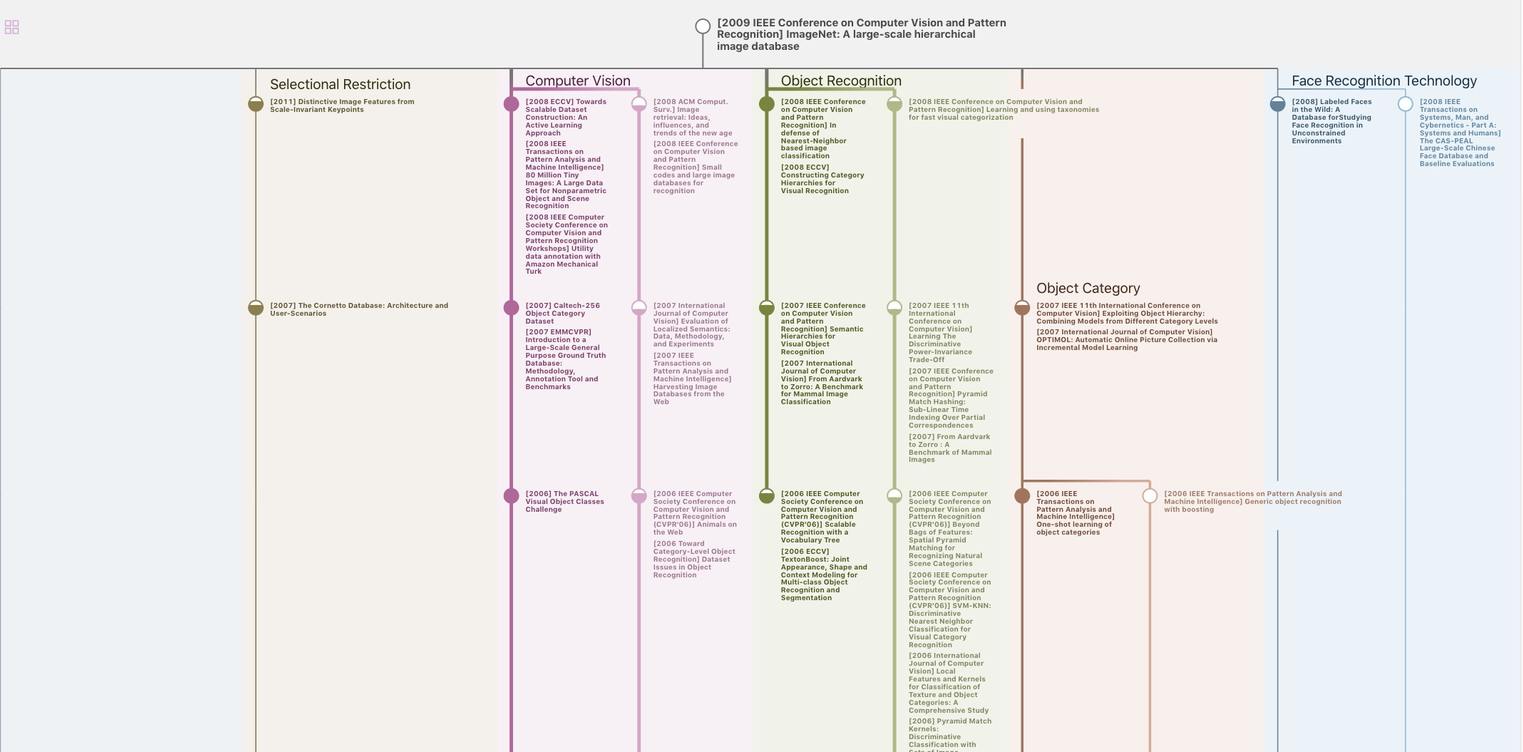
生成溯源树,研究论文发展脉络
Chat Paper
正在生成论文摘要