Evil Twins: Modeling Power Users In Attacks On Recommender Systems
USER MODELING, ADAPTATION, AND PERSONALIZATION, UMAP 2014(2014)
摘要
Attacks on Collaborative Filtering Recommender Systems (RS) can bias recommendations, potentially causing users to distrust results and the overall system. Attackers constantly innovate, and understanding the implications of novel attack vectors on system robustness is important for designers and operators. Foundational research on attacks in RSs studied attack user profiles based on straightforward models such as random or average ratings data. We are studying a novel category of attack based explicitly on measures of influence, in particular the potential impact of high-influence power users. This paper describes our approach to generate synthetic attack profiles that emulate influence characteristics of real power users, and it studies the impact of attack vectors that use synthetic power user profiles. We evaluate both the quality of synthetic power user profiles and the effectiveness of the attack, on both user-based and matrix-factorization-based recommender systems. Results show that synthetic user profiles that model real power users are an effective way of attacking collaborative recommender systems.
更多查看译文
关键词
Recommender Systems,Power User,Attacks,Evaluation
AI 理解论文
溯源树
样例
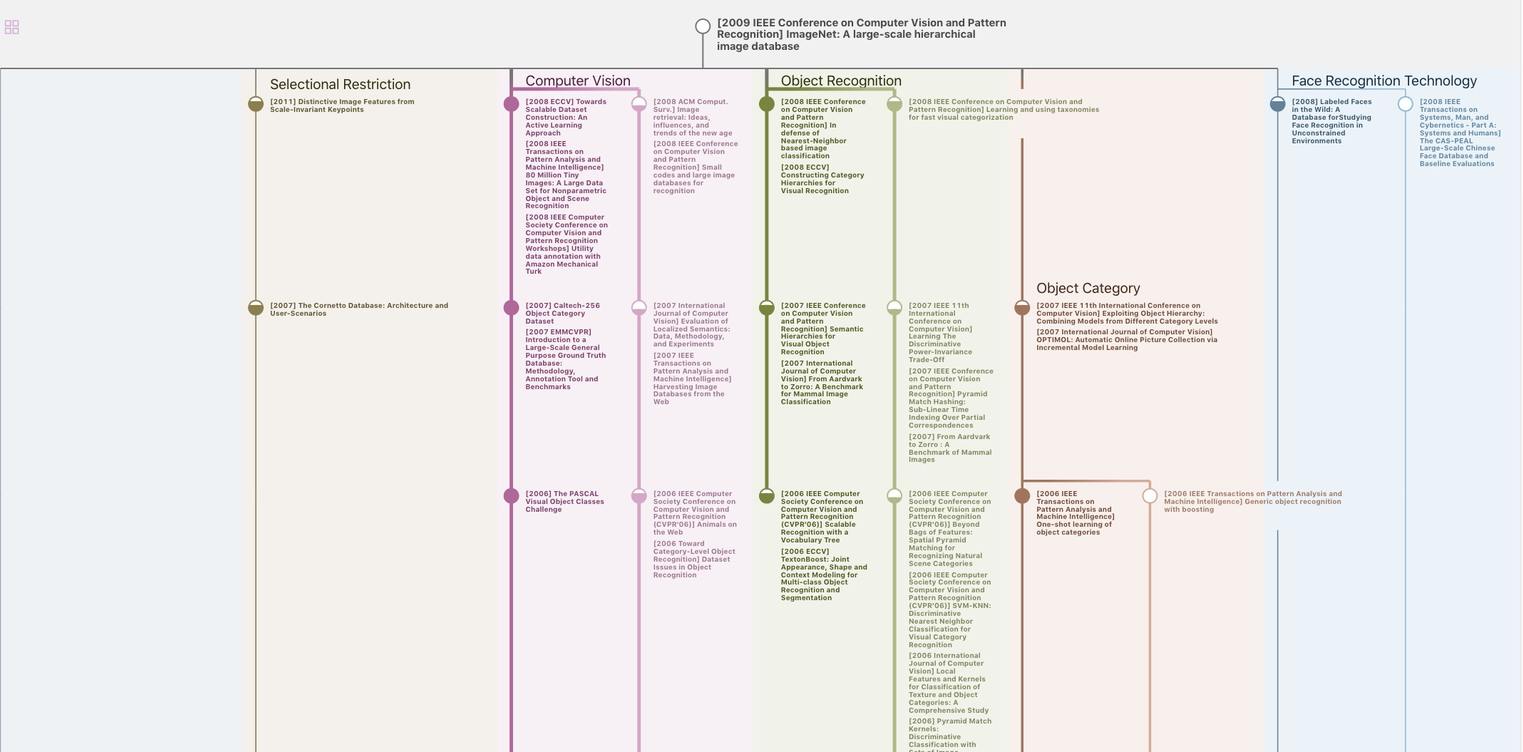
生成溯源树,研究论文发展脉络
Chat Paper
正在生成论文摘要