Simulation of tomographic medical image data for training of generic segmentation models utilizing multivariate feature classification.
SummerSim '14: Proceedings of the 2014 Summer Simulation Multiconference(2014)
摘要
Whenever generic segmentation strategies utilizing multivariate feature classification are developed in the medical domain, testing and training solely on real world data is insufficient to validate all possible feature aspects. Instead, utilization of a simulator is highly recommended to test against different feature distributions with varying levels of correlation. In this work a simulator is presented, not only generating correlated feature values but also providing geometric representations of the regions to classify, as well as simulated intensity volumes. Geometric representations of predefined shape primitives are simulated utilizing randomized dilation for steering surface characteristics. For intensity volume generation, gradient magnitude level, intra region homogeneity and border layout can be parameterized. The adjustable inter dataset variability allows for generation of entire training and validation datasets for model-based segmentation approaches.
更多查看译文
关键词
["correlated feature simulation","intensity volume simulation","mathematical morphology","randomized dilation"]
AI 理解论文
溯源树
样例
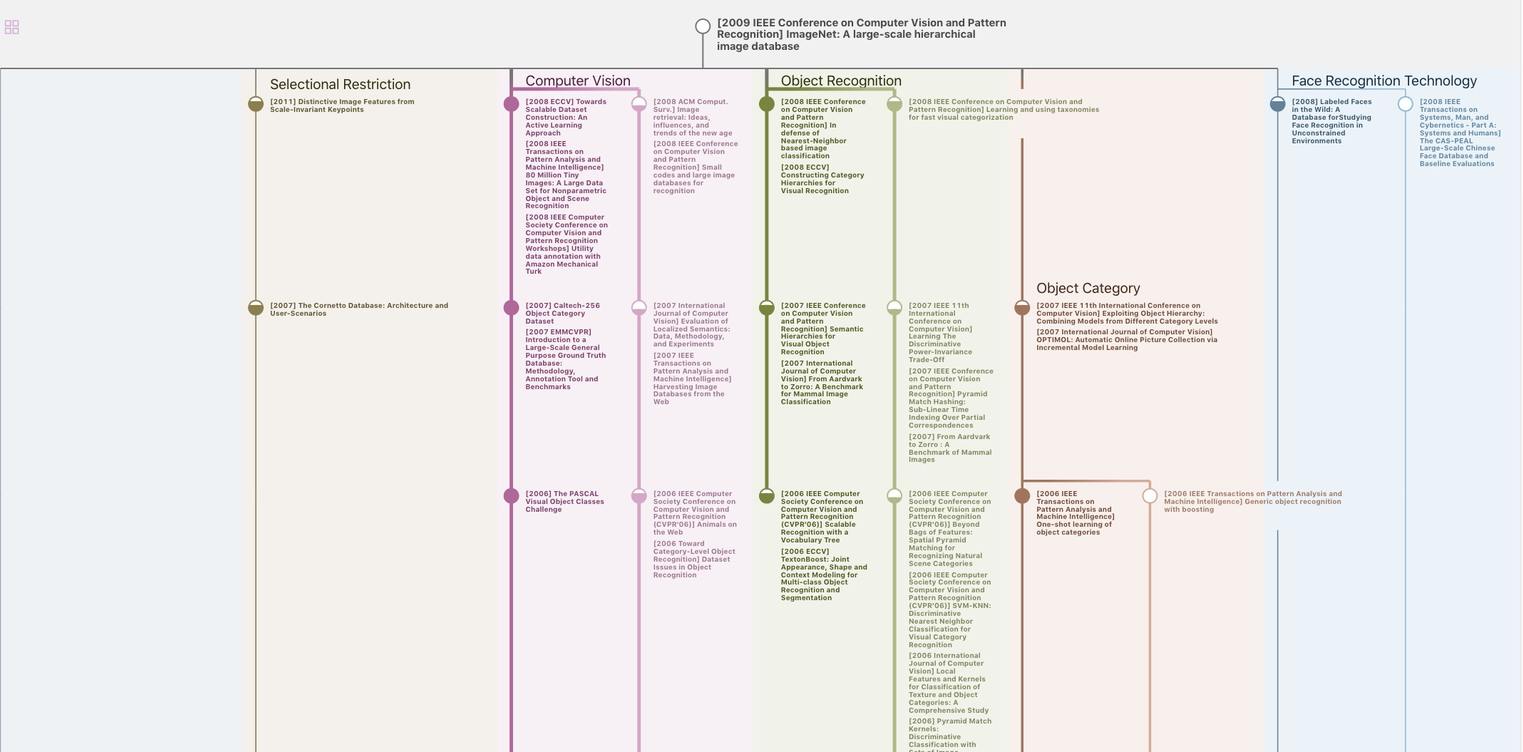
生成溯源树,研究论文发展脉络
Chat Paper
正在生成论文摘要