Efficient Anomaly Detection by Isolation Using Nearest Neighbour Ensemble
Data Mining Workshop(2014)
摘要
This paper presents iNNE (isolation using Nearest Neighbour Ensemble), an efficient nearest neighbour-based anomaly detection method by isolation. Inne runs significantly faster than existing nearest neighbour-based methods such as Local Outlier Factor, especially in data sets having thousands of dimensions or millions of instances. This is because the proposed method has linear time complexity and constant space complexity. Compared with the existing tree-based isolation method iForest, the proposed isolation method overcomes three weaknesses of iForest that we have identified, i.e., Its inability to detect local anomalies, anomalies with a low number of relevant attributes, and anomalies that are surrounded by normal instances.
更多查看译文
关键词
computational complexity,data mining,pattern recognition,constant space complexity,iForest,iNNE,isolation using nearest neighbour ensemble,linear time complexity,local outlier factor,nearest neighbour-based anomaly detection method,tree-based isolation method,anomaly detection,ensemble-based,nearest-neighbour
AI 理解论文
溯源树
样例
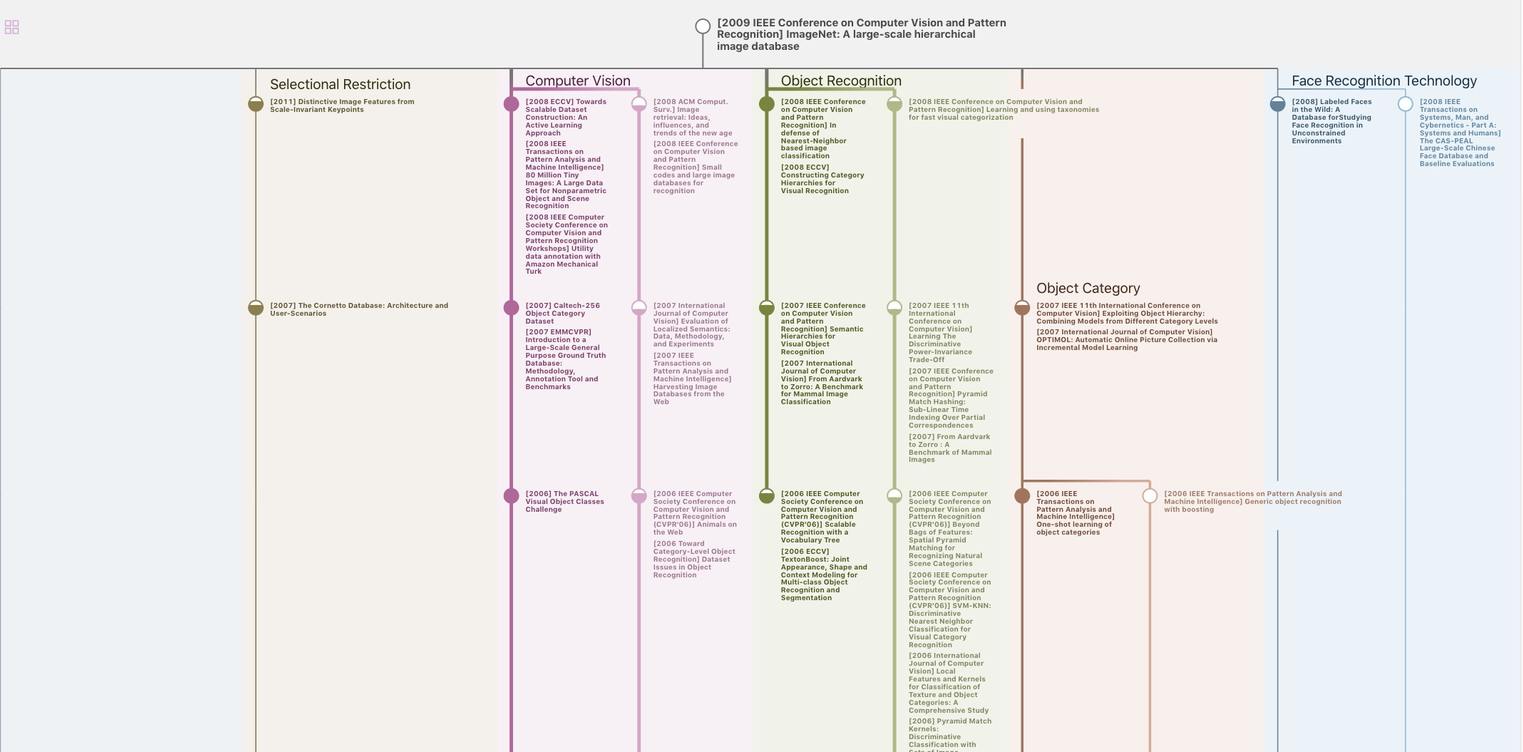
生成溯源树,研究论文发展脉络
Chat Paper
正在生成论文摘要