Content Matching For Short Duration Speaker Recognition
15TH ANNUAL CONFERENCE OF THE INTERNATIONAL SPEECH COMMUNICATION ASSOCIATION (INTERSPEECH 2014), VOLS 1-4(2014)
摘要
This work attempts to tackle the problem of content mismatch for short duration speaker verification. Experiments are run on both text-dependent and text-independent protocols, where a larger amount of enrollment data is available in the latter. We recently proposed a framework based on a deep neural network that explicitly utilizes phonetic information, and showed increased performance on long duration utterances. We show how this new framework can also yield significant improvements for short duration. We then propose an innovative approach to perform content matching, i.e. transforming a text independent trial into a text-dependent one by mining content from a speaker's enrollment data to match the test utterance. We show how content matching can be effectively done at the statistics level to enable the use of standard verification backends. Experiments run on the RSR2015 and NIST SRE 2010 data sets show relative improvements of 50% for cases where the content has been said during enrollment. While no significant improvements were observed for the general text-independent case, we believe that this work might pave the way for new research for speaker verification with very short utterances.
更多查看译文
AI 理解论文
溯源树
样例
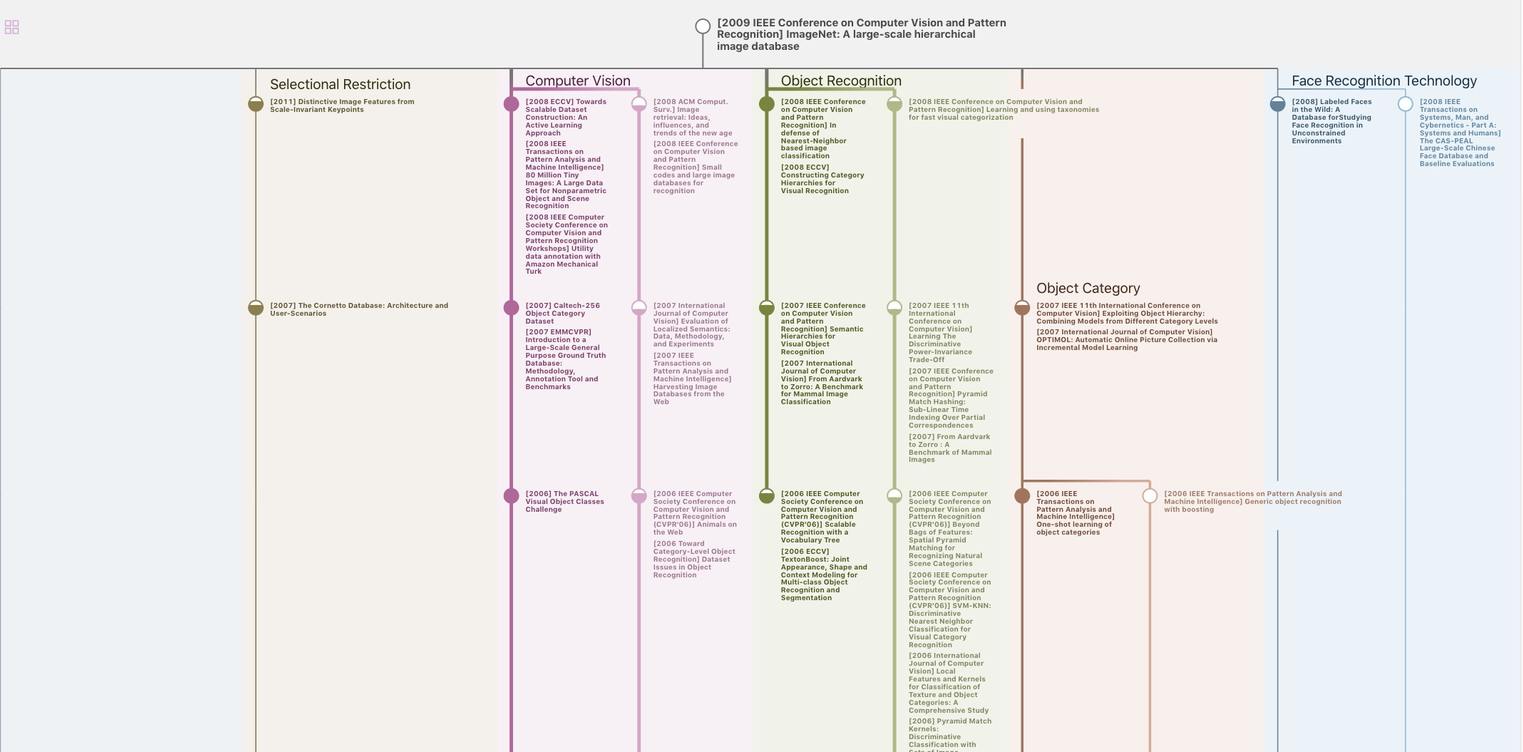
生成溯源树,研究论文发展脉络
Chat Paper
正在生成论文摘要