An Adaptive Subdivision Scheme For Quadratic Programming In Multi-Label Image Segmentation
PROCEEDINGS OF THE BRITISH MACHINE VISION CONFERENCE 2013(2013)
摘要
We address the problem of efficient, globally optimal multi-label image segmentation. Transforming the discrete labeling problem into a [0, 1]-relaxed binary quadratic program we are able to solve arbitrary convex quadratic labeling tasks in polynomial time. Although this guarantees efficiency in a theoretical sense, large-scale quadratic programs that arise from relaxation can rarely be used for image segmentation directly. We treat this issue by an adaptive domain subdivision scheme, reducing the problem to a short sequence of spatially smoothed medium-scale programs, which subsequently better approximate the large-scale program. Our scheme is globally optimal in terms of the approximated problem. It allows for near-interactive multi-label segmentation and is highly accurate even in the presence of strong noise.
更多查看译文
AI 理解论文
溯源树
样例
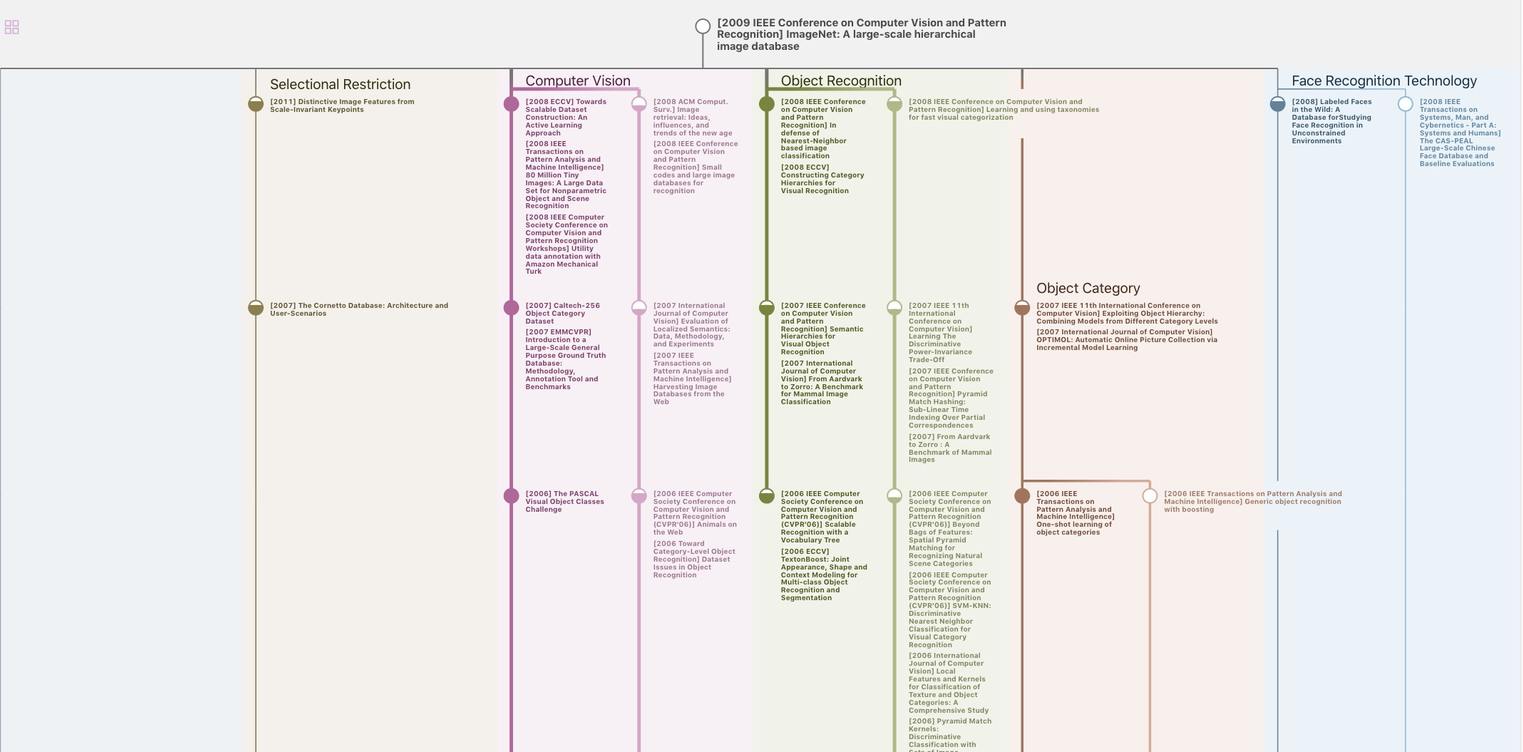
生成溯源树,研究论文发展脉络
Chat Paper
正在生成论文摘要