Fast Computation of Wasserstein Barycenters
ICML'14: Proceedings of the 31st International Conference on International Conference on Machine Learning - Volume 32(2014)
摘要
We present new algorithms to compute the mean of a set of empirical probability measures under the optimal transport metric. This mean, known as the Wasserstein barycenter, is the measure that minimizes the sum of its Wasserstein distances to each element in that set. We propose two original algorithms to compute Wasserstein barycenters that build upon the subgradient method. A direct implementation of these algorithms is, however, too costly because it would require the repeated resolution of large primal and dual optimal transport problems to compute subgradients. Extending the work of Cuturi (2013), we propose to smooth the Wasserstein distance used in the definition of Wasserstein barycenters with an entropic regularizer and recover in doing so a strictly convex objective whose gradients can be computed for a considerably cheaper computational cost using matrix scaling algorithms. We use these algorithms to visualize a large family of images and to solve a constrained clustering problem.
更多查看译文
AI 理解论文
溯源树
样例
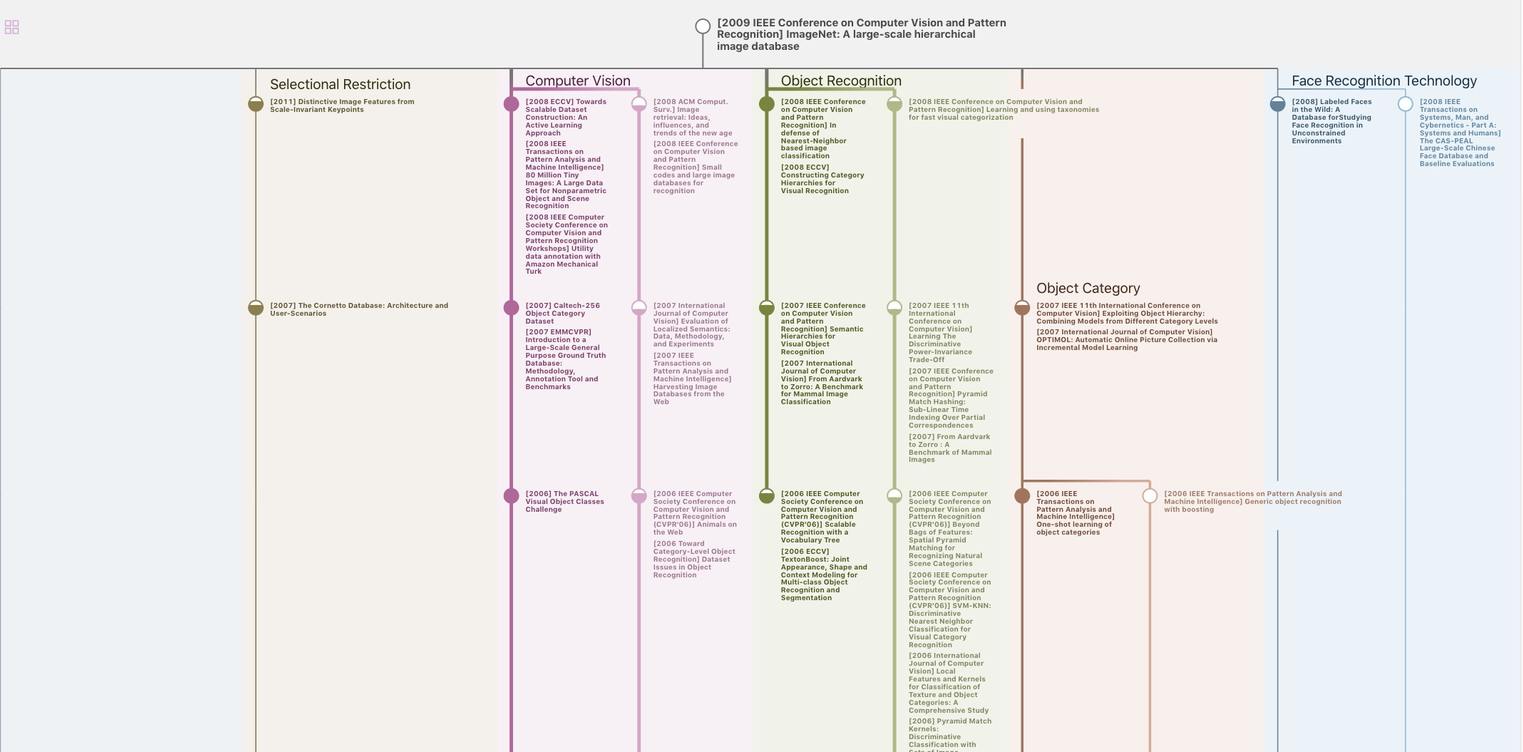
生成溯源树,研究论文发展脉络
Chat Paper
正在生成论文摘要