Parameter-free discovery and recommendation of areas-of-interest.
GIS(2014)
摘要
ABSTRACTThe task of discovering places of interest is a key step for many location-based recommendation tasks. In this paper we propose a fully unsupervised and parameter-free approach to deal with this problem based on the collection of geotagged photos. While previous papers are mostly devoted to discovering points (POI), we focus on areas of interest (AOI). Recommendation of better matches the traditional tourist goals and allows to robustly incorporate the interests of many users resulting in less subjective recommendations. The typical question that can be answered with the algorithm is formulated as "Where can one spend T minutes/hours walking around to observe as many attractive places as possible?" The proposed method starts with estimating multiple density hypotheses and then partitions these densities with the watershed segmentation algorithm into regions. The implicit parameters are tuned automatically to fit tourist goals and constraints resulting in a parameter-free algorithm. In spite of the parameter optimization overhead, the method is computationally efficient as it employs fast Fourier transforms for convolutions. We test our approach on 7 different cities and quantitatively show that the proposed method consistently outperforms the state-of-the-art DBSCAN algorithm and its modern modification P-DBSCAN, providing up to several times better recommendations in terms of time required for city exploration.
更多查看译文
AI 理解论文
溯源树
样例
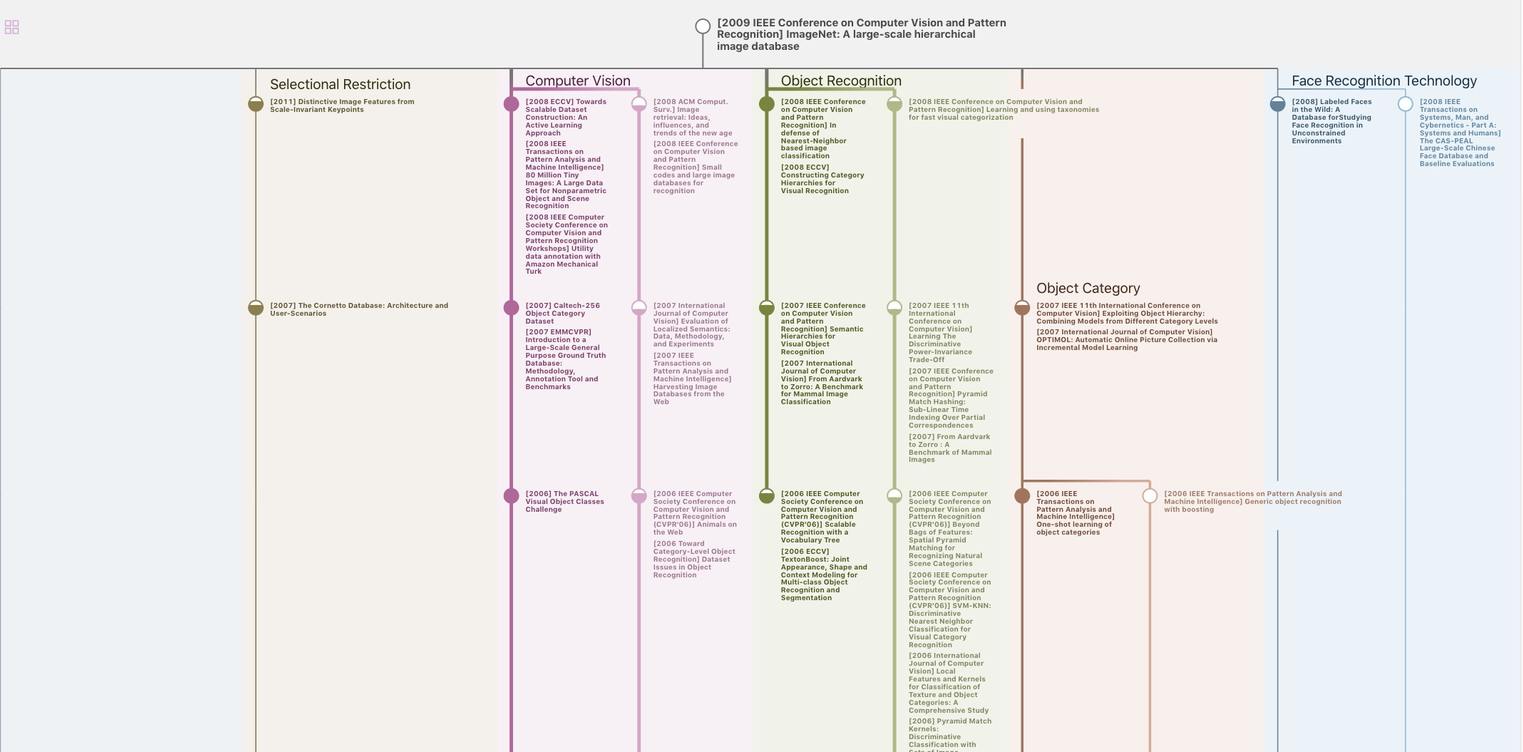
生成溯源树,研究论文发展脉络
Chat Paper
正在生成论文摘要