Visual Salience Learning Via Low Rank Matrix Recovery
COMPUTER VISION - ACCV 2014, PT III(2014)
摘要
Detection of salient object regions is useful for many vision tasks. Recently, a variety of saliency detection models have been proposed. They often behave differently over an individual image, and these saliency detection results often complement each other. To make full use of the advantages of the existing saliency detection methods, in this paper, we propose a salience learning model which combines various saliency detection methods such that the aggregation result outperforms each individual one. In our model, we first obtain several saliency maps by different saliency detection methods. The background regions of each saliency map usually lie in a low-dimensional subspace as most of them tend to have lower salience values, while the object regions that deviating from this subspace can be considered as sparse noises. So, an individual saliency map can be represented as a low rank matrix plus a sparse matrix. We aim at learning a unified sparse matrix that represents the salient regions using these obtained individual saliency maps. The sparse matrix can be inferred by conducting low rank matrix recovery using the robust principal component analysis technique. Experiments show that our model consistently outperforms each individual saliency detection approach and state-of-the-art methods.
更多查看译文
关键词
Salient Object, Salient Region, Saliency Detection, Saliency Model, Robust Principal Component Analysis
AI 理解论文
溯源树
样例
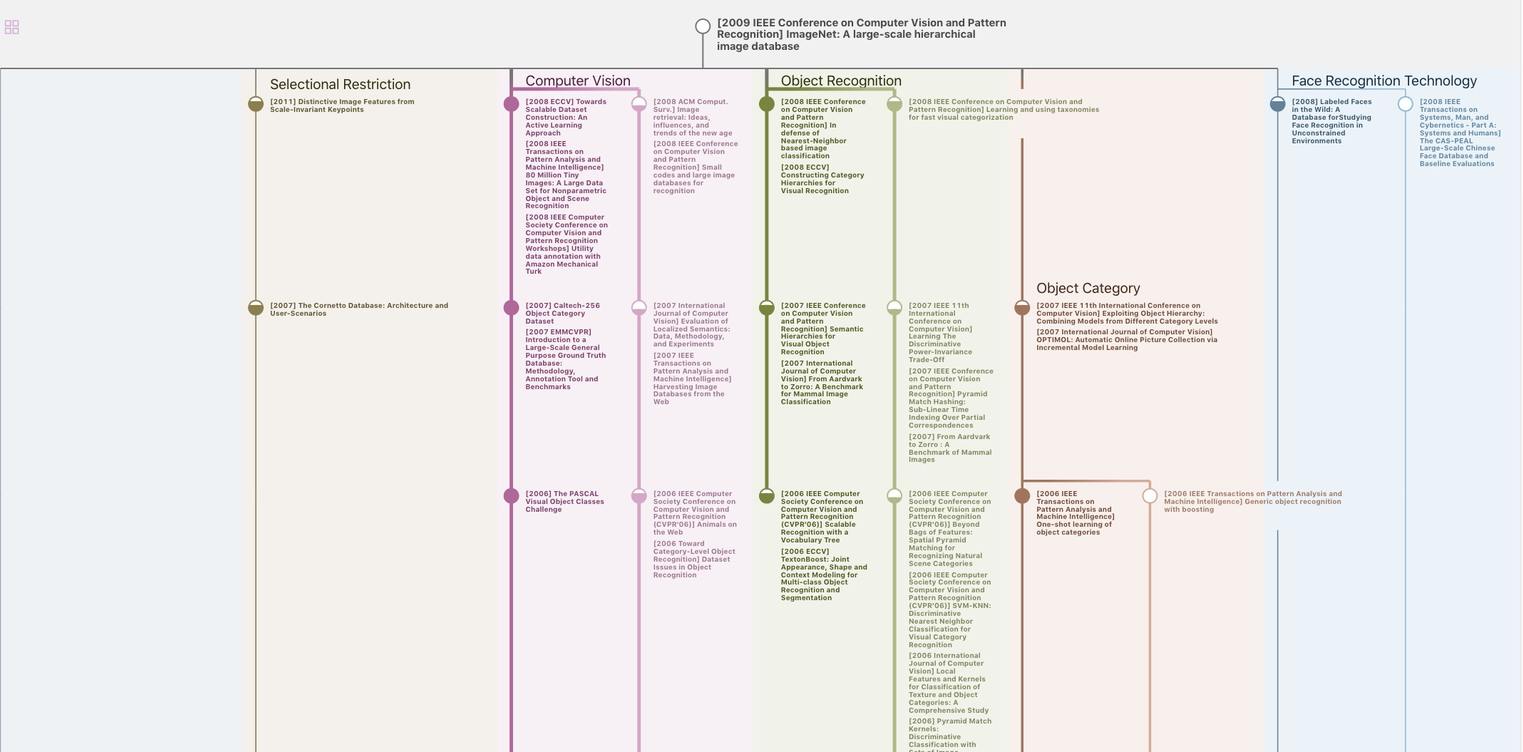
生成溯源树,研究论文发展脉络
Chat Paper
正在生成论文摘要