Discriminative Collaborative Representation For Classification
COMPUTER VISION - ACCV 2014, PT IV(2014)
摘要
The recently proposed l(2)-norm based collaborative representation for classification (CRC) model has shown inspiring performance on face recognition after the success of its predecessor - the l(1) - norm based sparse representation for classification (SRC) model. Though CRC is much faster than SRC as it has a closed-form solution, it may have the same weakness as SRC, i. e., relying on a "good" (properly controlled) training dataset for serving as its dictionary. Such a weakness limits the usage of CRC in real applications because the quality requirement is not easy to verify in practice. Inspired by the encouraging progress on dictionary learning for sparse representation, which can much alleviate this problem, we propose the discriminative collaborative representation (DCR) model. It has a novel classification model well fitting its discriminative learning model. As a result, DCR has the same advantage of being efficient as CRC, while at the same time showing even stronger discriminative power than existing dictionary learning methods. Extensive experiments on nine widely used benchmark datasets for both controlled and uncontrolled classification tasks demonstrate its consistent effectiveness and efficiency.
更多查看译文
AI 理解论文
溯源树
样例
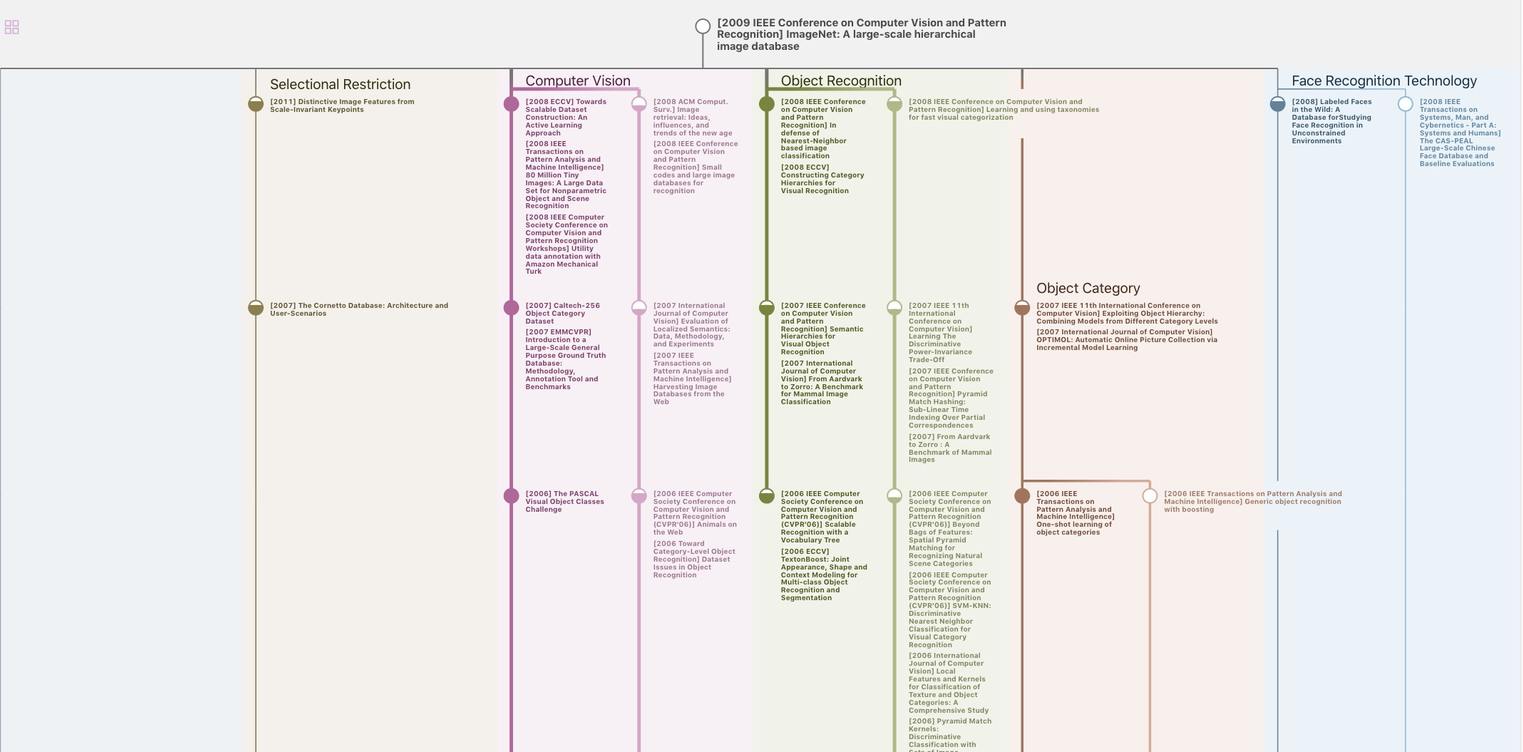
生成溯源树,研究论文发展脉络
Chat Paper
正在生成论文摘要