Transferring influence: Supervised learning for efficient influence maximization across networks
CollaborateCom(2014)
摘要
How to maximize influence through social networks is a key challenge behind many important applications in real life. For instance, marketers are interested in how to use limited resource to promote a new product as widely recognized by consumers. In recent years, researchers have conducted numerous studies to conquer this intriguing problem in single network scenario. In terms of the scale of achieved influence, the best solution is a greedy algorithm based on time-consuming Monte Carlo (MC) simulation. However, it is not scalable to large-scale social networks or the scenario of targeting multiple networks.We propose an innovative Transfer Influence Learning (TIL) method based on the study on three real networks, as well as statistics on network features of results generated by the greedy algorithm. The proposed method uses supervised learning technique to efficiently maximize influence across multiple networks. Once having the result of the greedy algorithm in one network, the TIL algorithm can avoid using MC simulation completely on other networks, which enables the algorithm to run very fast. The experiments show that the proposed TIL algorithm is able to generate a diffusion with closed scale comparing to the result of the greedy algorithm within a much faster time, while outperforms some other state-of-art heuristic algorithms.
更多查看译文
关键词
large-scale social networks,viral marketing,learning (artificial intelligence),real networks,monte carlo simulation,promotion (marketing),social network,network features,data mining,greedy algorithm,single-network scenario,influence maximization,transfer influence learning method,greedy algorithms,monte carlo methods,til method,multiple networks,supervised learning,mc simulation,network theory (graphs),supervised learning technique,product promotion
AI 理解论文
溯源树
样例
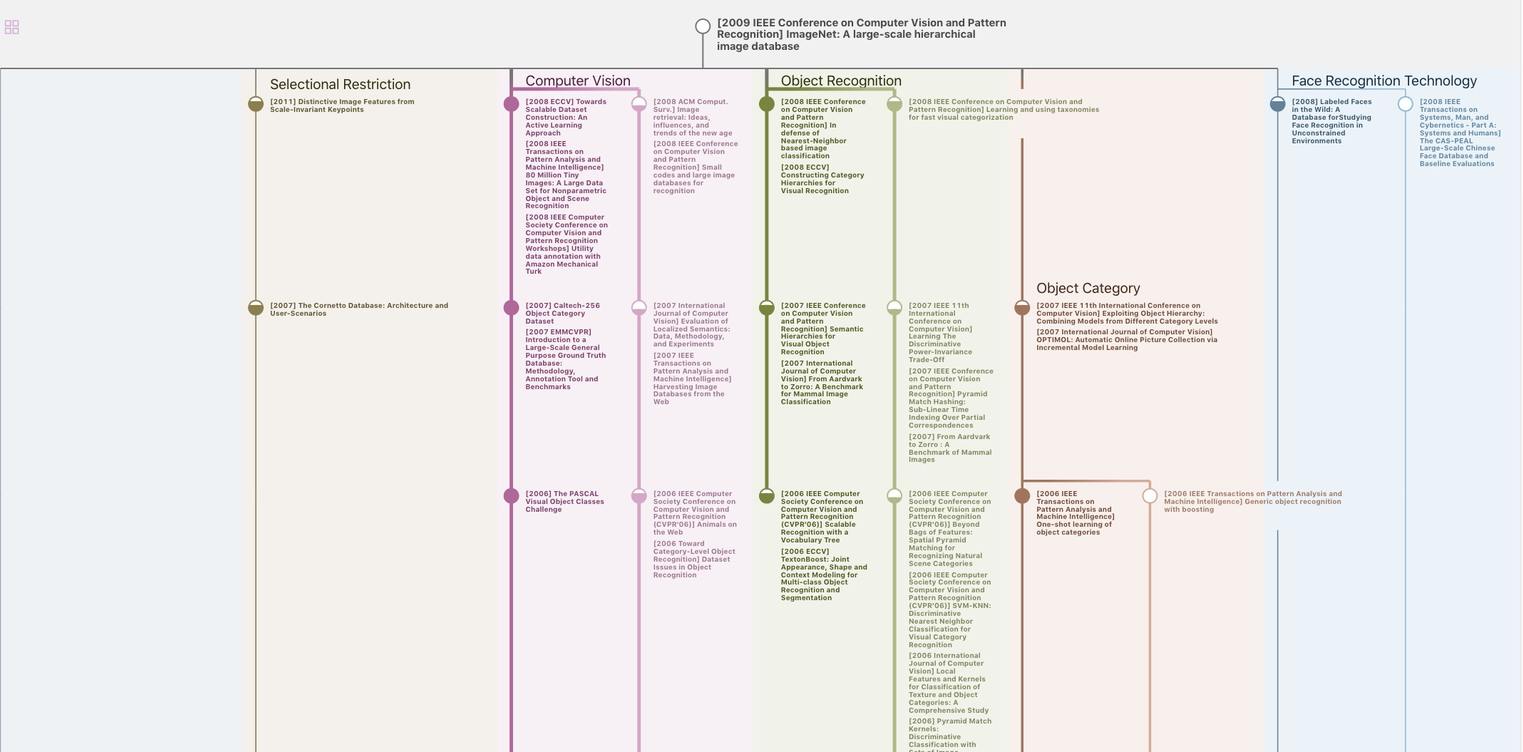
生成溯源树,研究论文发展脉络
Chat Paper
正在生成论文摘要