A new boosting design of Support Vector Machine classifiers
Information Fusion(2015)
摘要
Boosting algorithms pay attention to the particular structure of the training data when learning, by means of iteratively emphasizing the importance of the training samples according to their difficulty for being correctly classified. If common kernel Support Vector Machines (SVMs) are used as basic learners to construct a Real AdaBoost ensemble, the resulting ensemble can be easily compacted into a monolithic architecture by simply combining the weights that correspond to the same kernels when they appear in different learners, avoiding to increase the operation computational effort for the above potential advantage. This way, the performance advantage that boosting provides can be obtained for monolithic SVMs, i.e., without paying in classification computational effort because many learners are needed. However, SVMs are both stable and strong, and their use for boosting requires to unstabilize and to weaken them. Yet previous attempts in this direction show a moderate success.
更多查看译文
关键词
Real AdaBoost,Subsampling,Support Vector Machines,Linear programming,Ensemble classifiers
AI 理解论文
溯源树
样例
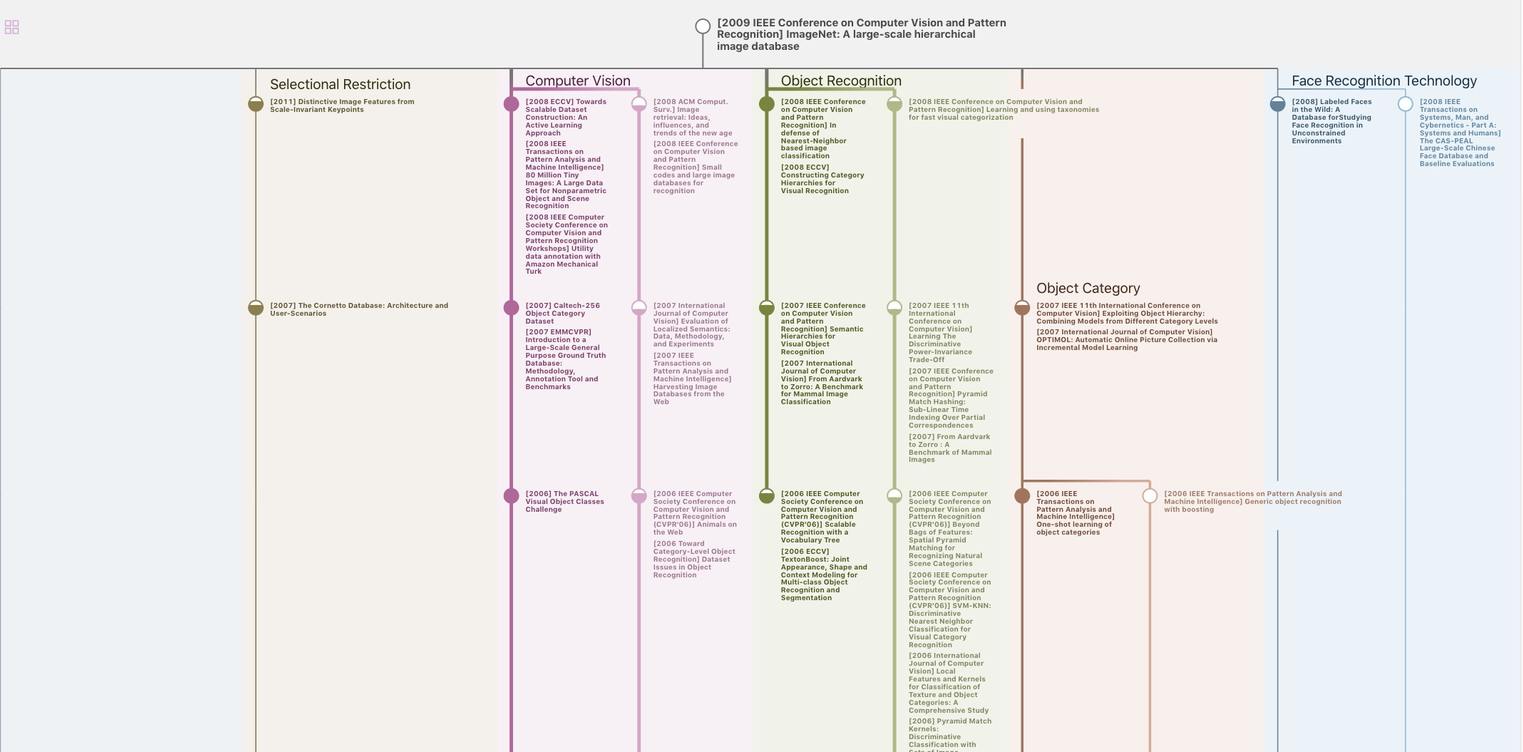
生成溯源树,研究论文发展脉络
Chat Paper
正在生成论文摘要