A pattern recognition framework for detecting dynamic changes on cyclic time series
Pattern Recognition(2015)
Abstract
This paper proposes a framework for binary classification of the time series with cyclic characteristics. The framework presents an iterative algorithm for learning the cyclic characteristics by introducing the discriminative frequency bands (DFBs) using the discriminant analysis along with k-means clustering method. The DFBs are employed by a hybrid model for learning dynamic characteristics of the time series within the cycles, using statistical and structural machine learning techniques. The framework offers a systematic procedure for finding the optimal design parameters associated with the hybrid model. The proposed model is optimized to detect the changes of the heart sound recordings (HSRs) related to aortic stenosis. Experimental results show that the proposed framework provides efficient tools for the classification of the HSRs based on the heart murmurs. It is also evidenced that the hybrid model, proposed by the framework, substantially improves the classification performance when it comes to detection of the heart disease. HighlightsWe present a novel framework for classifying cyclic stochastic time series.The framework suggests a novel hybrid HMM-SVM model.Cyclic contents of the time series are learned by in the preprocessing phase.Considerations for "one versus C classes" classification is taken into account.The performance is improved by the model in a demanding clinical study.
MoreTranslated text
Key words
phonocardiogram,time series,medical engineering
AI Read Science
Must-Reading Tree
Example
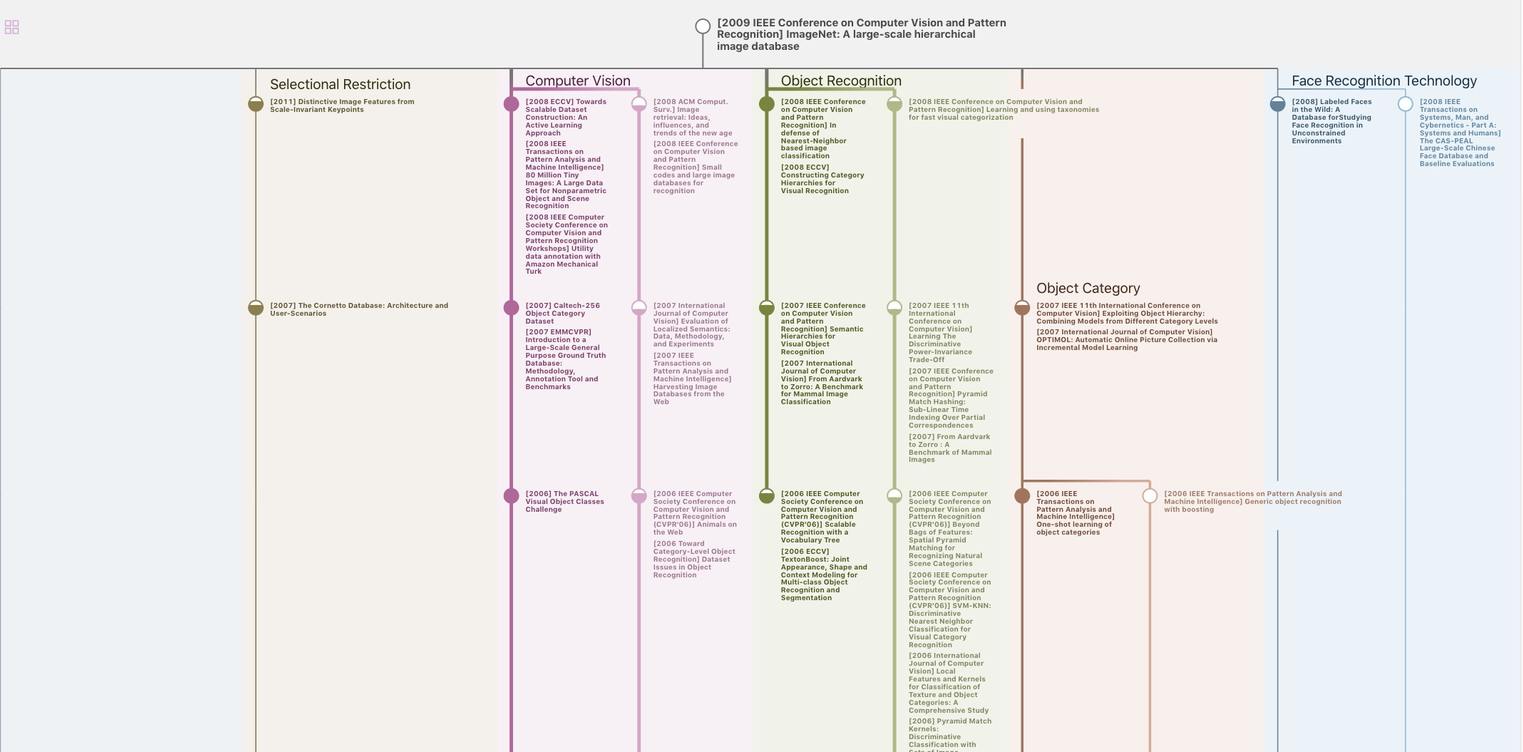
Generate MRT to find the research sequence of this paper
Chat Paper
Summary is being generated by the instructions you defined