A framework to uncover multiple alternative clusterings
Machine Learning(2013)
摘要
Clustering is often referred to as unsupervised learning which aims at uncovering hidden structures from data. Unfortunately, though widely being used as one of the principal tools to understand the data, most conventional clustering techniques are limited in achieving this goal since they only attempt to find a single clustering solution from the data. For many real-world applications, especially those being described in high dimensional data, it is common to see that the data can be grouped into different yet meaningful ways. This gives rise to the recently emerging research area of mining alternative clusterings. In this paper, we propose a framework named MACL that is capable of discovering multiple alternative clusterings from a given dataset. MACL seeks alternative clusterings in sequence and a novel solution is found by conditioning on all previously known clusterings. The framework takes a mathematically appealing approach by combining the maximum likelihood framework and mutual information. Consequently, its resultant clustering quality is achieved by the likelihood maximization over the data whereas the dissimilarity is ensured by the minimization over the information sharing amongst alternatives. We test the proposed algorithm on both synthetic and real-world datasets and the experimental results demonstrate its potential in discovering multiple alternative clusterings from data.
更多查看译文
关键词
Unsupervised learning,Alternative clustering,Expectation maximization,Mutual information
AI 理解论文
溯源树
样例
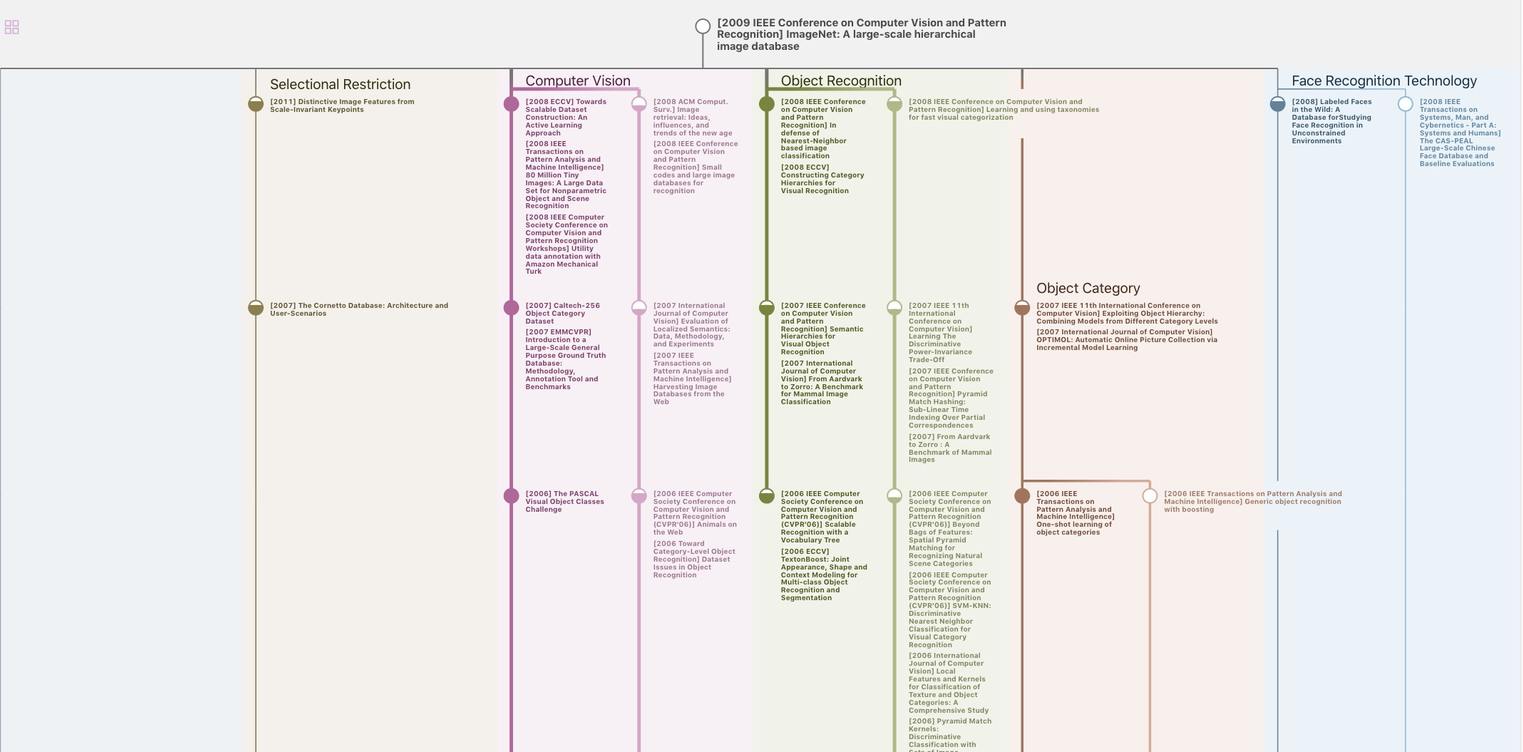
生成溯源树,研究论文发展脉络
Chat Paper
正在生成论文摘要